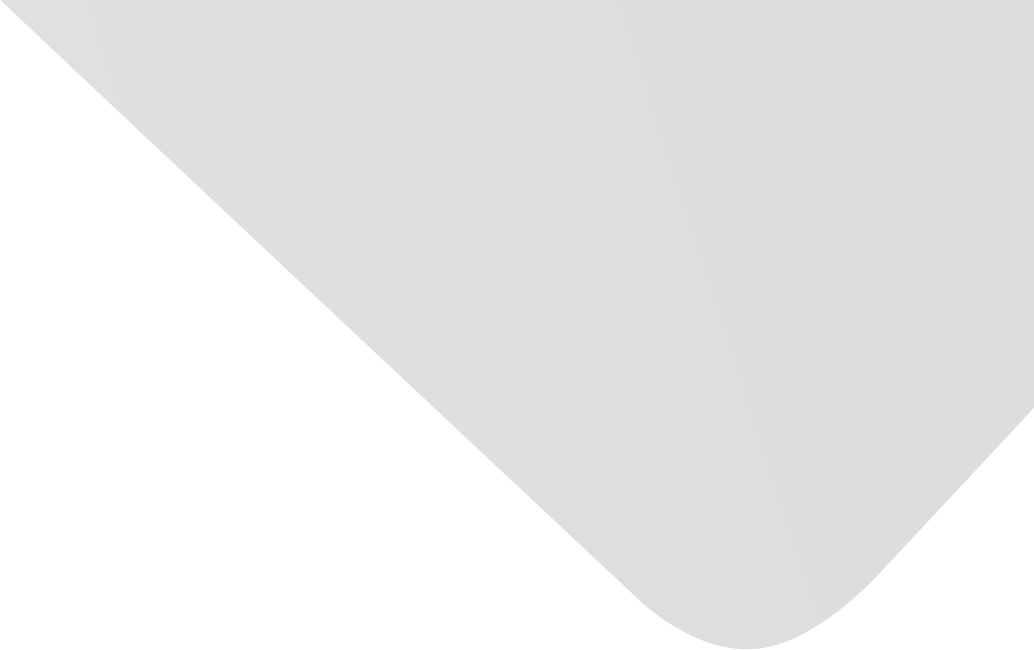
Traffic Accident Prediction Based on LSTM-GBRT Model
Joint Authors
Zhang, Zhihao
Yang, Wenzhong
Wushour, Silamu
Source
Journal of Control Science and Engineering
Issue
Vol. 2020, Issue 2020 (31 Dec. 2020), pp.1-10, 10 p.
Publisher
Hindawi Publishing Corporation
Publication Date
2020-03-05
Country of Publication
Egypt
No. of Pages
10
Main Subjects
Electronic engineering
Information Technology and Computer Science
Abstract EN
Road traffic accidents are a concrete manifestation of road traffic safety levels.
The current traffic accident prediction has a problem of low accuracy.
In order to provide traffic management departments with more accurate forecast data, it can be applied in the traffic management system to help make scientific decisions.
This paper establishes a traffic accident prediction model based on LSTM-GBRT (long short-term memory, gradient boosted regression trees) and predicts traffic accident safety level indicators by training traffic accident-related data.
Compared with various regression models and neural network models, the experimental results show that the LSTM-GBRT model has a good fitting effect and robustness.
The LSTM-GBRT model can accurately predict the safety level of traffic accidents, so that the traffic management department can better grasp the situation of traffic safety levels.
American Psychological Association (APA)
Zhang, Zhihao& Yang, Wenzhong& Wushour, Silamu. 2020. Traffic Accident Prediction Based on LSTM-GBRT Model. Journal of Control Science and Engineering،Vol. 2020, no. 2020, pp.1-10.
https://search.emarefa.net/detail/BIM-1182682
Modern Language Association (MLA)
Zhang, Zhihao…[et al.]. Traffic Accident Prediction Based on LSTM-GBRT Model. Journal of Control Science and Engineering No. 2020 (2020), pp.1-10.
https://search.emarefa.net/detail/BIM-1182682
American Medical Association (AMA)
Zhang, Zhihao& Yang, Wenzhong& Wushour, Silamu. Traffic Accident Prediction Based on LSTM-GBRT Model. Journal of Control Science and Engineering. 2020. Vol. 2020, no. 2020, pp.1-10.
https://search.emarefa.net/detail/BIM-1182682
Data Type
Journal Articles
Language
English
Notes
Includes bibliographical references
Record ID
BIM-1182682