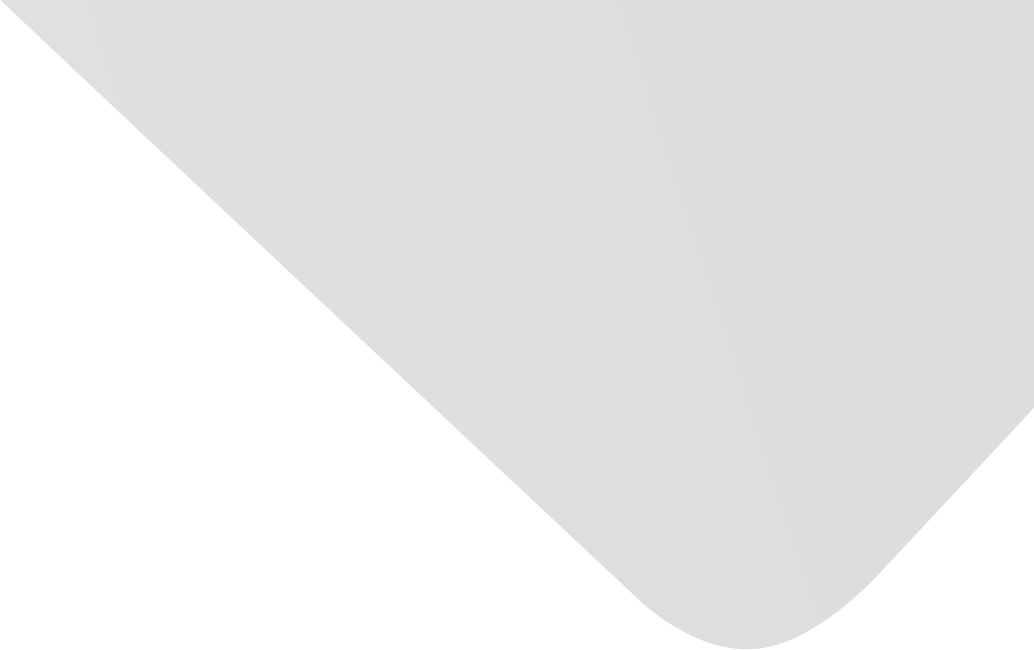
Gap Prediction in Hybrid Graphene-Hexagonal Boron Nitride Nanoflakes Using Artificial Neural Networks
Joint Authors
Nemnes, G. A.
Mitran, T. L.
Manolescu, A.
Source
Issue
Vol. 2019, Issue 2019 (31 Dec. 2019), pp.1-8, 8 p.
Publisher
Hindawi Publishing Corporation
Publication Date
2019-05-16
Country of Publication
Egypt
No. of Pages
8
Main Subjects
Abstract EN
The electronic properties of graphene nanoflakes (GNFs) with embedded hexagonal boron nitride (hBN) domains are investigated by combined ab initio density functional theory calculations and machine-learning techniques.
The energy gaps of the quasi-0D graphene-based systems, defined as the differences between LUMO and HOMO energies, depend not only on the sizes of the hBN domains relative to the size of the pristine graphene nanoflake but also on the position of the hBN domain.
The range of the energy gaps for different configurations increases as the hBN domains get larger.
We develop two artificial neural network (ANN) models able to reproduce the gap energies with high accuracies and investigate the tunability of the energy gap, by considering a set of GNFs with embedded rectangular hBN domains.
In one ANN model, the input is in one-to-one correspondence with the atoms in the GNF, while in the second model the inputs account for basic structures in the GNF, allowing potential use in upscaled systems.
We perform a statistical analysis over different configurations of ANNs to optimize the network structure.
The trained ANNs provide a correlation between the atomic system configuration and the magnitude of the energy gaps, which may be regarded as an efficient tool for optimizing the design of nanostructured graphene-based materials for specific electronic properties.
American Psychological Association (APA)
Nemnes, G. A.& Mitran, T. L.& Manolescu, A.. 2019. Gap Prediction in Hybrid Graphene-Hexagonal Boron Nitride Nanoflakes Using Artificial Neural Networks. Journal of Nanomaterials،Vol. 2019, no. 2019, pp.1-8.
https://search.emarefa.net/detail/BIM-1182856
Modern Language Association (MLA)
Nemnes, G. A.…[et al.]. Gap Prediction in Hybrid Graphene-Hexagonal Boron Nitride Nanoflakes Using Artificial Neural Networks. Journal of Nanomaterials No. 2019 (2019), pp.1-8.
https://search.emarefa.net/detail/BIM-1182856
American Medical Association (AMA)
Nemnes, G. A.& Mitran, T. L.& Manolescu, A.. Gap Prediction in Hybrid Graphene-Hexagonal Boron Nitride Nanoflakes Using Artificial Neural Networks. Journal of Nanomaterials. 2019. Vol. 2019, no. 2019, pp.1-8.
https://search.emarefa.net/detail/BIM-1182856
Data Type
Journal Articles
Language
English
Notes
Includes bibliographical references
Record ID
BIM-1182856