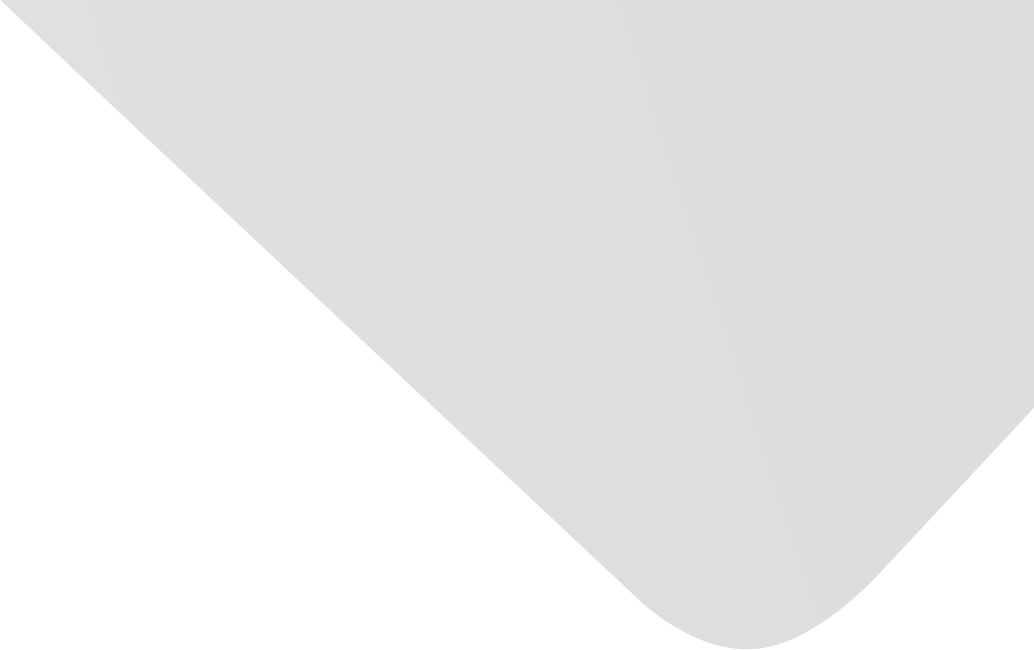
Multiple Feature Vectors Based Fault Classification for WSN Integrated Bearing of Rolling Mill
Joint Authors
Qin, Bo
Zhang, Luyang
Yin, Heng
Qin, Yan
Source
Journal of Control Science and Engineering
Issue
Vol. 2018, Issue 2018 (31 Dec. 2018), pp.1-11, 11 p.
Publisher
Hindawi Publishing Corporation
Publication Date
2018-04-01
Country of Publication
Egypt
No. of Pages
11
Main Subjects
Electronic engineering
Information Technology and Computer Science
Abstract EN
For rolling mill machines, the operation status of bearing has a close relationship with process safety and production effectiveness.
Therefore, reliable fault diagnosis and classification are indispensable.
Traditional methods always characterize fault feature using a single fault vector, which may fail to reveal whole fault influences caused by complex process disturbances.
Besides, it may also lead to poor fault classification accuracy.
To solve the above-mentioned problems, a fault extraction method is put forward to extract multiple feature vectors and then a classification model is developed.
First, to collect sufficient data, a data acquisition system based on wireless sensor network is constructed to replace the traditional wired system which may bring dangers during production.
Second, the measured signal is filtered by a morphological average filtering algorithm to remove process noise and then the empirical mode decomposition method is applied to extract the intrinsic mode function (IMF) which contains the fault information.
On the basis of the IMFs, a time domain index (energy) and a frequency index (singular values) are proposed through Hilbert envelope analysis.
From the above analysis, the energy index and the singular value matrix are used for fault classification modeling based on the enhanced extreme learning machine (ELM), which is optimized by the bat algorithm to adjust the input weights and threshold of hidden layer node.
In comparison with the fault classification methods based on SVM and ELM, the experimental results show that the proposed method has higher classification accuracy and better generalization ability.
American Psychological Association (APA)
Qin, Bo& Zhang, Luyang& Yin, Heng& Qin, Yan. 2018. Multiple Feature Vectors Based Fault Classification for WSN Integrated Bearing of Rolling Mill. Journal of Control Science and Engineering،Vol. 2018, no. 2018, pp.1-11.
https://search.emarefa.net/detail/BIM-1182931
Modern Language Association (MLA)
Qin, Bo…[et al.]. Multiple Feature Vectors Based Fault Classification for WSN Integrated Bearing of Rolling Mill. Journal of Control Science and Engineering No. 2018 (2018), pp.1-11.
https://search.emarefa.net/detail/BIM-1182931
American Medical Association (AMA)
Qin, Bo& Zhang, Luyang& Yin, Heng& Qin, Yan. Multiple Feature Vectors Based Fault Classification for WSN Integrated Bearing of Rolling Mill. Journal of Control Science and Engineering. 2018. Vol. 2018, no. 2018, pp.1-11.
https://search.emarefa.net/detail/BIM-1182931
Data Type
Journal Articles
Language
English
Notes
Includes bibliographical references
Record ID
BIM-1182931