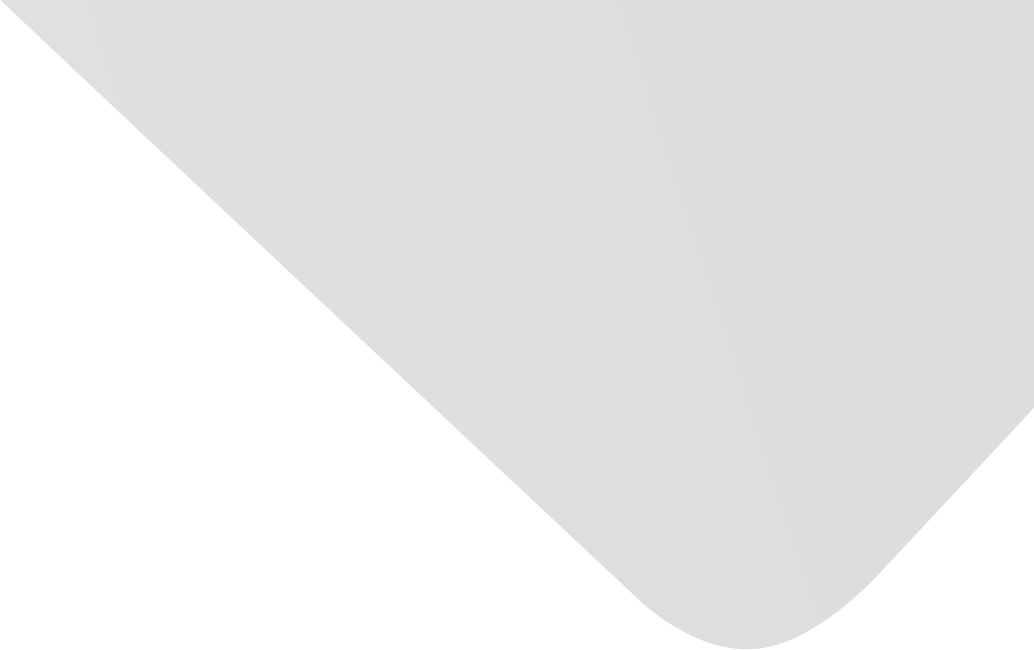
Deep Sparse Autoencoder for Feature Extraction and Diagnosis of Locomotive Adhesion Status
Joint Authors
Zhang, Chang-fan
He, Jing
Liu, Jianhua
Cheng, Xiang
Liu, Guangwei
Source
Journal of Control Science and Engineering
Issue
Vol. 2018, Issue 2018 (31 Dec. 2018), pp.1-9, 9 p.
Publisher
Hindawi Publishing Corporation
Publication Date
2018-07-15
Country of Publication
Egypt
No. of Pages
9
Main Subjects
Electronic engineering
Information Technology and Computer Science
Abstract EN
The model is difficult to establish because the principle of the locomotive adhesion process is complex.
This paper presents a data-driven adhesion status fault diagnosis method based on deep learning theory.
The adhesion coefficient and creep speed of a locomotive constitute the characteristic vector.
The sparse autoencoder unsupervised learning network studies the input vector, and the single-layer network is superimposed to form a deep neural network.
Finally, a small amount of labeled data is used to fine-tune training the entire deep neural network, and the locomotive adhesion state fault diagnosis model is established.
Experimental results show that the proposed method can achieve a 99.3% locomotive adhesion state diagnosis accuracy and satisfy actual engineering monitoring requirements.
American Psychological Association (APA)
Zhang, Chang-fan& Cheng, Xiang& Liu, Jianhua& He, Jing& Liu, Guangwei. 2018. Deep Sparse Autoencoder for Feature Extraction and Diagnosis of Locomotive Adhesion Status. Journal of Control Science and Engineering،Vol. 2018, no. 2018, pp.1-9.
https://search.emarefa.net/detail/BIM-1183084
Modern Language Association (MLA)
Zhang, Chang-fan…[et al.]. Deep Sparse Autoencoder for Feature Extraction and Diagnosis of Locomotive Adhesion Status. Journal of Control Science and Engineering No. 2018 (2018), pp.1-9.
https://search.emarefa.net/detail/BIM-1183084
American Medical Association (AMA)
Zhang, Chang-fan& Cheng, Xiang& Liu, Jianhua& He, Jing& Liu, Guangwei. Deep Sparse Autoencoder for Feature Extraction and Diagnosis of Locomotive Adhesion Status. Journal of Control Science and Engineering. 2018. Vol. 2018, no. 2018, pp.1-9.
https://search.emarefa.net/detail/BIM-1183084
Data Type
Journal Articles
Language
English
Notes
Includes bibliographical references
Record ID
BIM-1183084