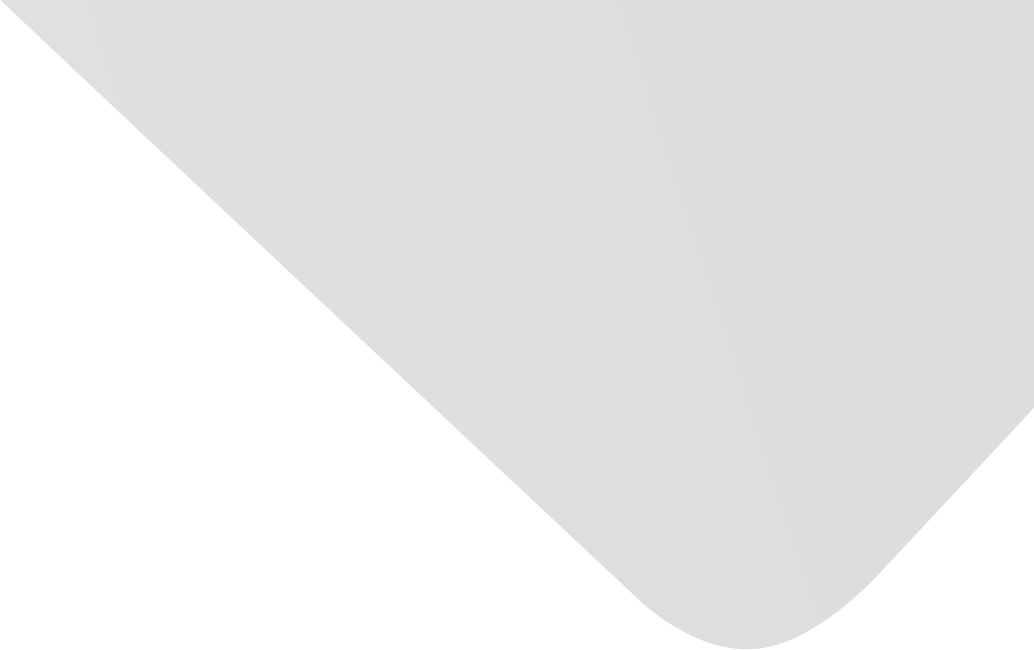
Comparison of Machine Learning Methods and Conventional Logistic Regressions for Predicting Gestational Diabetes Using Routine Clinical Data: A Retrospective Cohort Study
Joint Authors
Wu, Jiang-Nan
Zhou, Qiong-Jie
Ye, Yunzhen
Xiong, Yu
Xiao, Xirong
Li, Xiaotian
Source
Issue
Vol. 2020, Issue 2020 (31 Dec. 2020), pp.1-10, 10 p.
Publisher
Hindawi Publishing Corporation
Publication Date
2020-06-12
Country of Publication
Egypt
No. of Pages
10
Main Subjects
Abstract EN
Background.
Gestational diabetes mellitus (GDM) contributes to adverse pregnancy and birth outcomes.
In recent decades, extensive research has been devoted to the early prediction of GDM by various methods.
Machine learning methods are flexible prediction algorithms with potential advantages over conventional regression.
Objective.
The purpose of this study was to use machine learning methods to predict GDM and compare their performance with that of logistic regressions.
Methods.
We performed a retrospective, observational study including women who attended their routine first hospital visits during early pregnancy and had Down’s syndrome screening at 16-20 gestational weeks in a tertiary maternity hospital in China from 2013.1.1 to 2017.12.31.
A total of 22,242 singleton pregnancies were included, and 3182 (14.31%) women developed GDM.
Candidate predictors included maternal demographic characteristics and medical history (maternal factors) and laboratory values at early pregnancy.
The models were derived from the first 70% of the data and then validated with the next 30%.
Variables were trained in different machine learning models and traditional logistic regression models.
Eight common machine learning methods (GDBT, AdaBoost, LGB, Logistic, Vote, XGB, Decision Tree, and Random Forest) and two common regressions (stepwise logistic regression and logistic regression with RCS) were implemented to predict the occurrence of GDM.
Models were compared on discrimination and calibration metrics.
Results.
In the validation dataset, the machine learning and logistic regression models performed moderately (AUC 0.59-0.74).
Overall, the GBDT model performed best (AUC 0.74, 95% CI 0.71-0.76) among the machine learning methods, with negligible differences between them.
Fasting blood glucose, HbA1c, triglycerides, and BMI strongly contributed to GDM.
A cutoff point for the predictive value at 0.3 in the GBDT model had a negative predictive value of 74.1% (95% CI 69.5%-78.2%) and a sensitivity of 90% (95% CI 88.0%-91.7%), and the cutoff point at 0.7 had a positive predictive value of 93.2% (95% CI 88.2%-96.1%) and a specificity of 99% (95% CI 98.2%-99.4%).
Conclusion.
In this study, we found that several machine learning methods did not outperform logistic regression in predicting GDM.
We developed a model with cutoff points for risk stratification of GDM.
American Psychological Association (APA)
Ye, Yunzhen& Xiong, Yu& Zhou, Qiong-Jie& Wu, Jiang-Nan& Li, Xiaotian& Xiao, Xirong. 2020. Comparison of Machine Learning Methods and Conventional Logistic Regressions for Predicting Gestational Diabetes Using Routine Clinical Data: A Retrospective Cohort Study. Journal of Diabetes Research،Vol. 2020, no. 2020, pp.1-10.
https://search.emarefa.net/detail/BIM-1183117
Modern Language Association (MLA)
Ye, Yunzhen…[et al.]. Comparison of Machine Learning Methods and Conventional Logistic Regressions for Predicting Gestational Diabetes Using Routine Clinical Data: A Retrospective Cohort Study. Journal of Diabetes Research No. 2020 (2020), pp.1-10.
https://search.emarefa.net/detail/BIM-1183117
American Medical Association (AMA)
Ye, Yunzhen& Xiong, Yu& Zhou, Qiong-Jie& Wu, Jiang-Nan& Li, Xiaotian& Xiao, Xirong. Comparison of Machine Learning Methods and Conventional Logistic Regressions for Predicting Gestational Diabetes Using Routine Clinical Data: A Retrospective Cohort Study. Journal of Diabetes Research. 2020. Vol. 2020, no. 2020, pp.1-10.
https://search.emarefa.net/detail/BIM-1183117
Data Type
Journal Articles
Language
English
Notes
Includes bibliographical references
Record ID
BIM-1183117