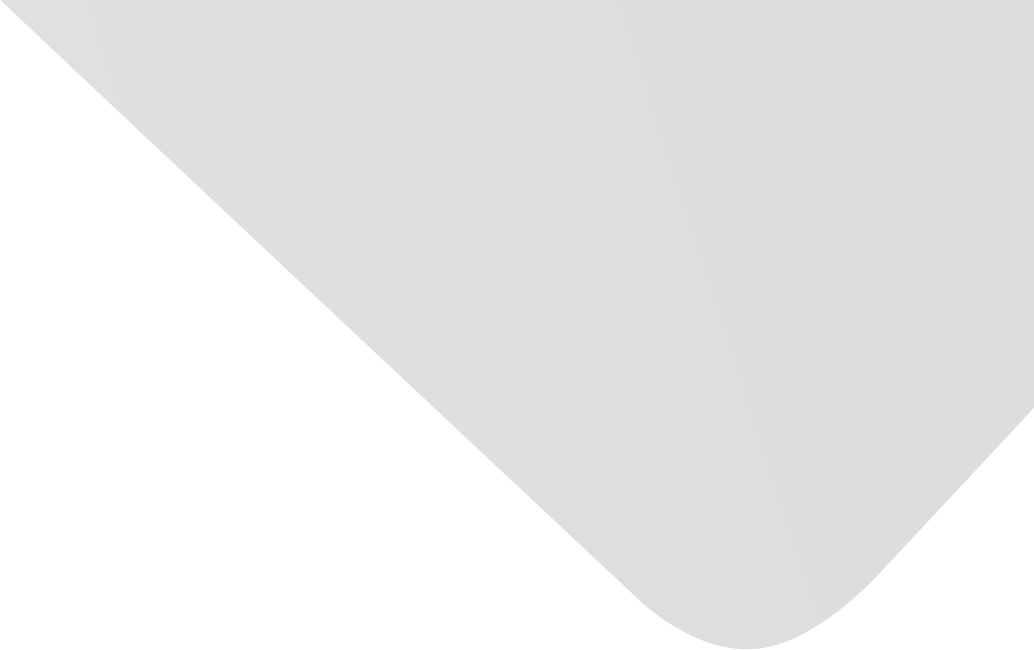
Hardware Module Design and Software Implementation of Multisensor Fire Detection and Notification System Using Fuzzy Logic and Convolutional Neural Networks (CNNs)
Joint Authors
Sowah, Robert A.
Apeadu, Kwaku O.
Gatsi, Francis
Ampadu, Kwame O.
Mensah, Baffour S.
Source
Issue
Vol. 2020, Issue 2020 (31 Dec. 2020), pp.1-16, 16 p.
Publisher
Hindawi Publishing Corporation
Publication Date
2020-02-01
Country of Publication
Egypt
No. of Pages
16
Main Subjects
Abstract EN
This paper presents the design and development of a fuzzy logic-based multisensor fire detection and a web-based notification system with trained convolutional neural networks for both proximity and wide-area fire detection.
Until recently, most consumer-grade fire detection systems relied solely on smoke detectors.
These offer limited protection due to the type of fire present and the detection technology at use.
To solve this problem, we present a multisensor data fusion with convolutional neural network (CNN) fire detection and notification technology.
Convolutional Neural Networks are mainstream methods of deep learning due to their ability to perform feature extraction and classification in the same architecture.
The system is designed to enable early detection of fire in residential, commercial, and industrial environments by using multiple fire signatures such as flames, smoke, and heat.
The incorporation of the convolutional neural networks enables broader coverage of the area of interest, using visuals from surveillance cameras.
With access granted to the web-based system, the fire and rescue crew gets notified in real-time with location information.
The efficiency of the fire detection and notification system employed by standard fire detectors and the multisensor remote-based notification approach adopted in this paper showed significant improvements with timely fire detection, alerting, and response time for firefighting.
The final experimental and performance evaluation results showed that the accuracy rate of CNN was 94% and that of the fuzzy logic unit is 90%.
American Psychological Association (APA)
Sowah, Robert A.& Apeadu, Kwaku O.& Gatsi, Francis& Ampadu, Kwame O.& Mensah, Baffour S.. 2020. Hardware Module Design and Software Implementation of Multisensor Fire Detection and Notification System Using Fuzzy Logic and Convolutional Neural Networks (CNNs). Journal of Engineering،Vol. 2020, no. 2020, pp.1-16.
https://search.emarefa.net/detail/BIM-1183677
Modern Language Association (MLA)
Sowah, Robert A.…[et al.]. Hardware Module Design and Software Implementation of Multisensor Fire Detection and Notification System Using Fuzzy Logic and Convolutional Neural Networks (CNNs). Journal of Engineering No. 2020 (2020), pp.1-16.
https://search.emarefa.net/detail/BIM-1183677
American Medical Association (AMA)
Sowah, Robert A.& Apeadu, Kwaku O.& Gatsi, Francis& Ampadu, Kwame O.& Mensah, Baffour S.. Hardware Module Design and Software Implementation of Multisensor Fire Detection and Notification System Using Fuzzy Logic and Convolutional Neural Networks (CNNs). Journal of Engineering. 2020. Vol. 2020, no. 2020, pp.1-16.
https://search.emarefa.net/detail/BIM-1183677
Data Type
Journal Articles
Language
English
Notes
Includes bibliographical references
Record ID
BIM-1183677