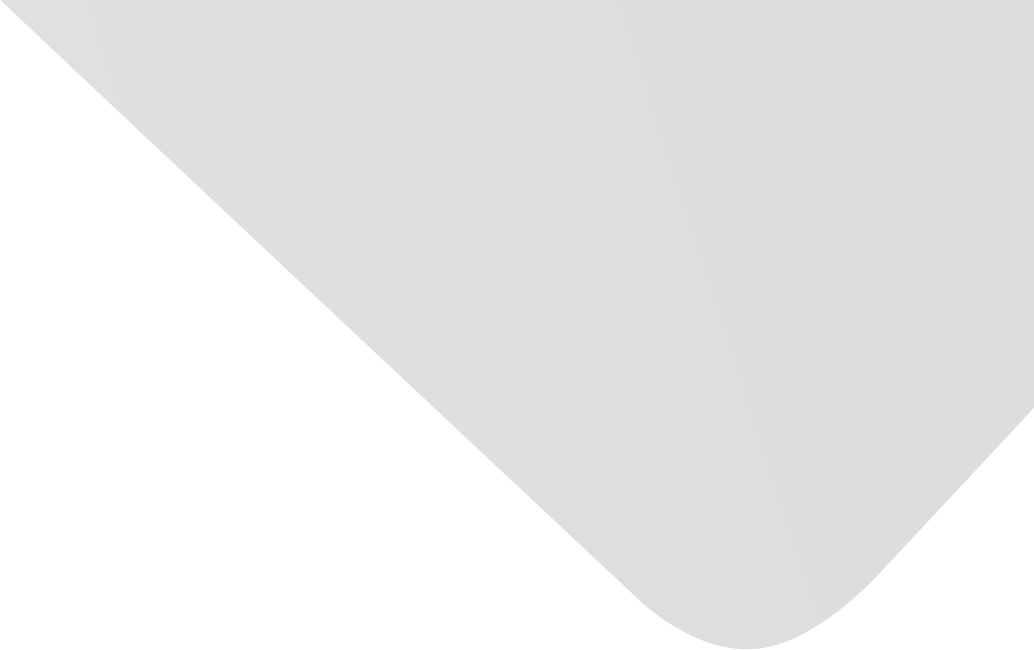
Feature Selection and ANN Solar Power Prediction
Joint Authors
Source
Issue
Vol. 2017, Issue 2017 (31 Dec. 2017), pp.1-7, 7 p.
Publisher
Hindawi Publishing Corporation
Publication Date
2017-11-08
Country of Publication
Egypt
No. of Pages
7
Main Subjects
Abstract EN
A novel method of solar power forecasting for individuals and small businesses is developed in this paper based on machine learning, image processing, and acoustic classification techniques.
Increases in the production of solar power at the consumer level require automated forecasting systems to minimize loss, cost, and environmental impact for homes and businesses that produce and consume power (prosumers).
These new participants in the energy market, prosumers, require new artificial neural network (ANN) performance tuning techniques to create accurate ANN forecasts.
Input masking, an ANN tuning technique developed for acoustic signal classification and image edge detection, is applied to prosumer solar data to improve prosumer forecast accuracy over traditional macrogrid ANN performance tuning techniques.
ANN inputs tailor time-of-day masking based on error clustering in the time domain.
Results show an improvement in prediction to target correlation, the R2 value, lowering inaccuracy of sample predictions by 14.4%, with corresponding drops in mean average error of 5.37% and root mean squared error of 6.83%.
American Psychological Association (APA)
O’Leary, Daniel& Kubby, Joel. 2017. Feature Selection and ANN Solar Power Prediction. Journal of Renewable Energy،Vol. 2017, no. 2017, pp.1-7.
https://search.emarefa.net/detail/BIM-1186451
Modern Language Association (MLA)
O’Leary, Daniel& Kubby, Joel. Feature Selection and ANN Solar Power Prediction. Journal of Renewable Energy No. 2017 (2017), pp.1-7.
https://search.emarefa.net/detail/BIM-1186451
American Medical Association (AMA)
O’Leary, Daniel& Kubby, Joel. Feature Selection and ANN Solar Power Prediction. Journal of Renewable Energy. 2017. Vol. 2017, no. 2017, pp.1-7.
https://search.emarefa.net/detail/BIM-1186451
Data Type
Journal Articles
Language
English
Notes
Includes bibliographical references
Record ID
BIM-1186451