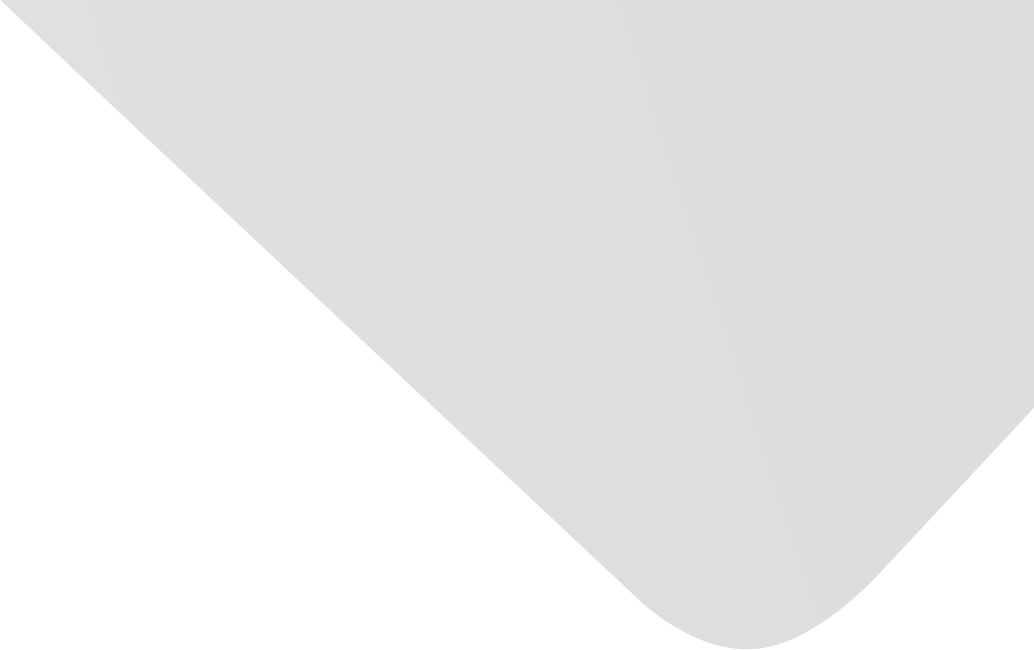
Automatic Semantic Segmentation of Brain Gliomas from MRI Images Using a Deep Cascaded Neural Network
Joint Authors
Jiang, Jingfeng
Cui, Shaoguo
Mao, Lei
Liu, Chang
Xiong, Shuyu
Source
Journal of Healthcare Engineering
Issue
Vol. 2018, Issue 2018 (31 Dec. 2018), pp.1-14, 14 p.
Publisher
Hindawi Publishing Corporation
Publication Date
2018-03-19
Country of Publication
Egypt
No. of Pages
14
Main Subjects
Abstract EN
Brain tumors can appear anywhere in the brain and have vastly different sizes and morphology.
Additionally, these tumors are often diffused and poorly contrasted.
Consequently, the segmentation of brain tumor and intratumor subregions using magnetic resonance imaging (MRI) data with minimal human interventions remains a challenging task.
In this paper, we present a novel fully automatic segmentation method from MRI data containing in vivo brain gliomas.
This approach can not only localize the entire tumor region but can also accurately segment the intratumor structure.
The proposed work was based on a cascaded deep learning convolutional neural network consisting of two subnetworks: (1) a tumor localization network (TLN) and (2) an intratumor classification network (ITCN).
The TLN, a fully convolutional network (FCN) in conjunction with the transfer learning technology, was used to first process MRI data.
The goal of the first subnetwork was to define the tumor region from an MRI slice.
Then, the ITCN was used to label the defined tumor region into multiple subregions.
Particularly, ITCN exploited a convolutional neural network (CNN) with deeper architecture and smaller kernel.
The proposed approach was validated on multimodal brain tumor segmentation (BRATS 2015) datasets, which contain 220 high-grade glioma (HGG) and 54 low-grade glioma (LGG) cases.
Dice similarity coefficient (DSC), positive predictive value (PPV), and sensitivity were used as evaluation metrics.
Our experimental results indicated that our method could obtain the promising segmentation results and had a faster segmentation speed.
More specifically, the proposed method obtained comparable and overall better DSC values (0.89, 0.77, and 0.80) on the combined (HGG + LGG) testing set, as compared to other methods reported in the literature.
Additionally, the proposed approach was able to complete a segmentation task at a rate of 1.54 seconds per slice.
American Psychological Association (APA)
Cui, Shaoguo& Mao, Lei& Jiang, Jingfeng& Liu, Chang& Xiong, Shuyu. 2018. Automatic Semantic Segmentation of Brain Gliomas from MRI Images Using a Deep Cascaded Neural Network. Journal of Healthcare Engineering،Vol. 2018, no. 2018, pp.1-14.
https://search.emarefa.net/detail/BIM-1187329
Modern Language Association (MLA)
Cui, Shaoguo…[et al.]. Automatic Semantic Segmentation of Brain Gliomas from MRI Images Using a Deep Cascaded Neural Network. Journal of Healthcare Engineering No. 2018 (2018), pp.1-14.
https://search.emarefa.net/detail/BIM-1187329
American Medical Association (AMA)
Cui, Shaoguo& Mao, Lei& Jiang, Jingfeng& Liu, Chang& Xiong, Shuyu. Automatic Semantic Segmentation of Brain Gliomas from MRI Images Using a Deep Cascaded Neural Network. Journal of Healthcare Engineering. 2018. Vol. 2018, no. 2018, pp.1-14.
https://search.emarefa.net/detail/BIM-1187329
Data Type
Journal Articles
Language
English
Notes
Includes bibliographical references
Record ID
BIM-1187329