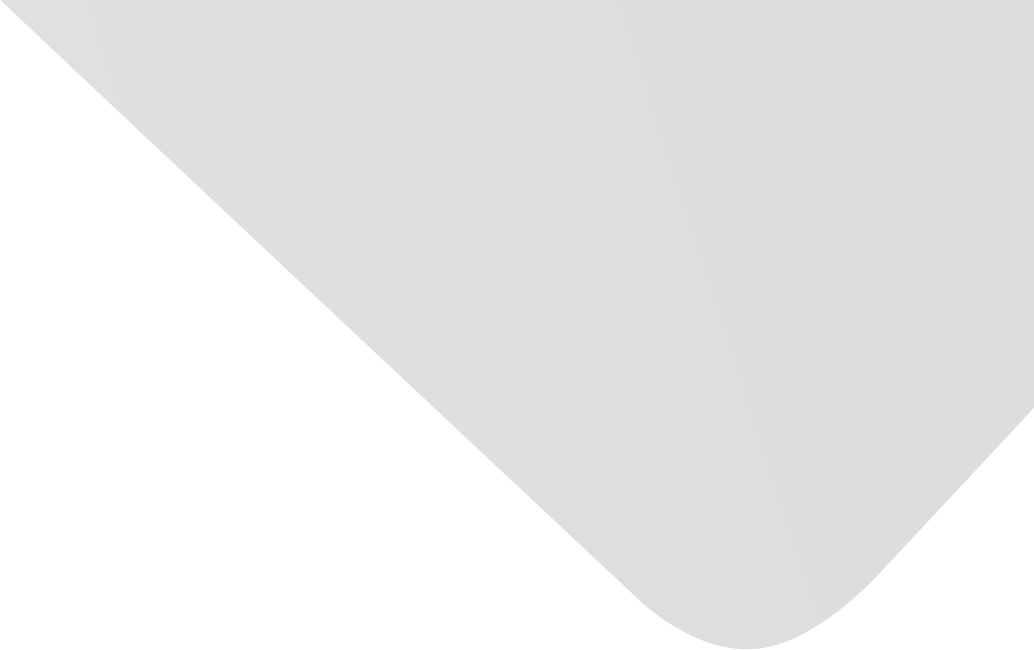
Local Binary Patterns Descriptor Based on Sparse Curvelet Coefficients for False-Positive Reduction in Mammograms
Joint Authors
Pawar, Meenakshi M.
Talbar, Sanjay N.
Dudhane, Akshay
Source
Journal of Healthcare Engineering
Issue
Vol. 2018, Issue 2018 (31 Dec. 2018), pp.1-16, 16 p.
Publisher
Hindawi Publishing Corporation
Publication Date
2018-09-25
Country of Publication
Egypt
No. of Pages
16
Main Subjects
Abstract EN
Breast Cancer is the most prevalent cancer among women across the globe.
Automatic detection of breast cancer using Computer Aided Diagnosis (CAD) system suffers from false positives (FPs).
Thus, reduction of FP is one of the challenging tasks to improve the performance of the diagnosis systems.
In the present work, new FP reduction technique has been proposed for breast cancer diagnosis.
It is based on appropriate integration of preprocessing, Self-organizing map (SOM) clustering, region of interest (ROI) extraction, and FP reduction.
In preprocessing, contrast enhancement of mammograms has been achieved using Local Entropy Maximization algorithm.
The unsupervised SOM clusters an image into number of segments to identify the cancerous region and extracts tumor regions (i.e., ROIs).
However, it also detects some FPs which affects the efficiency of the algorithm.
Therefore, to reduce the FPs, the output of the SOM is given to the FP reduction step which is aimed to classify the extracted ROIs into normal and abnormal class.
FP reduction consists of feature mining from the ROIs using proposed local sparse curvelet coefficients followed by classification using artificial neural network (ANN).
The performance of proposed algorithm has been validated using the local datasets as TMCH (Tata Memorial Cancer Hospital) and publicly available MIAS (Suckling et al., 1994) and DDSM (Heath et al., 2000) database.
The proposed technique results in reduction of FPs from 0.85 to 0.02 FP/image for MIAS, 4.81 to 0.16 FP/image for DDSM, and 2.32 to 0.05 FP/image for TMCH reflecting huge improvement in classification of mammograms.
American Psychological Association (APA)
Pawar, Meenakshi M.& Talbar, Sanjay N.& Dudhane, Akshay. 2018. Local Binary Patterns Descriptor Based on Sparse Curvelet Coefficients for False-Positive Reduction in Mammograms. Journal of Healthcare Engineering،Vol. 2018, no. 2018, pp.1-16.
https://search.emarefa.net/detail/BIM-1187442
Modern Language Association (MLA)
Pawar, Meenakshi M.…[et al.]. Local Binary Patterns Descriptor Based on Sparse Curvelet Coefficients for False-Positive Reduction in Mammograms. Journal of Healthcare Engineering No. 2018 (2018), pp.1-16.
https://search.emarefa.net/detail/BIM-1187442
American Medical Association (AMA)
Pawar, Meenakshi M.& Talbar, Sanjay N.& Dudhane, Akshay. Local Binary Patterns Descriptor Based on Sparse Curvelet Coefficients for False-Positive Reduction in Mammograms. Journal of Healthcare Engineering. 2018. Vol. 2018, no. 2018, pp.1-16.
https://search.emarefa.net/detail/BIM-1187442
Data Type
Journal Articles
Language
English
Notes
Includes bibliographical references
Record ID
BIM-1187442