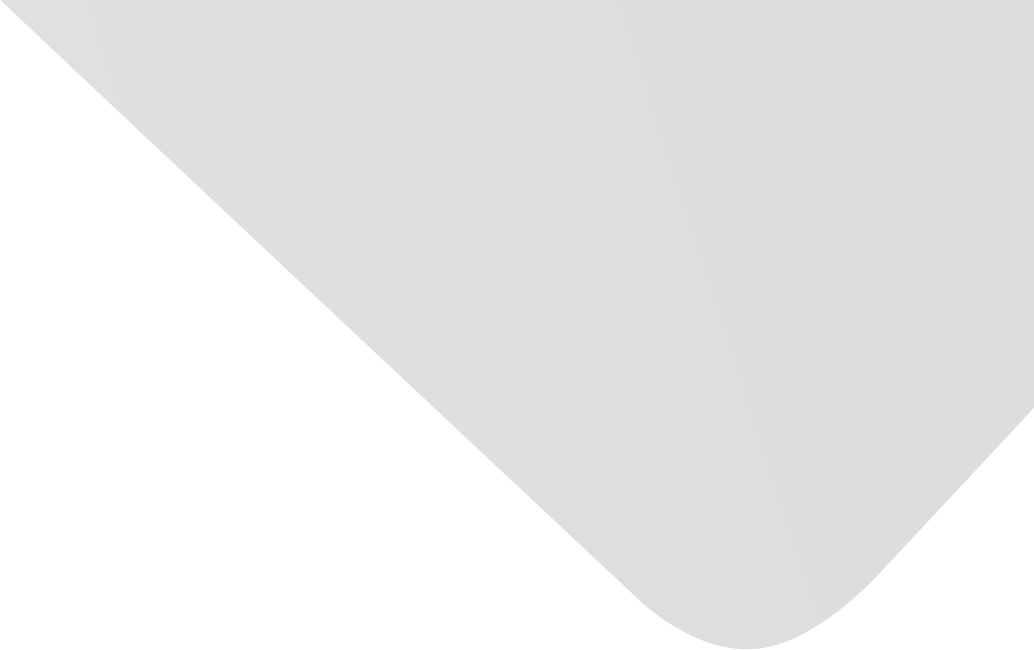
Deep Learning-Based GNSS Network-Based Real-Time Kinematic Improvement for Autonomous Ground Vehicle Navigation
Joint Authors
Source
Issue
Vol. 2019, Issue 2019 (31 Dec. 2019), pp.1-8, 8 p.
Publisher
Hindawi Publishing Corporation
Publication Date
2019-03-31
Country of Publication
Egypt
No. of Pages
8
Main Subjects
Abstract EN
Much navigation over the last several decades has been aided by the global navigation satellite system (GNSS).
In addition, with the advent of the multi-GNSS era, more and more satellites are available for navigation purposes.
However, the navigation is generally carried out by point positioning based on the pseudoranges.
The real-time kinematic (RTK) and the advanced technology, namely, the network RTK (NRTK), were introduced for better positioning and navigation.
Further improved navigation was also investigated by combining other sensors such as the inertial measurement unit (IMU).
On the other hand, a deep learning technique has been recently evolving in many fields, including automatic navigation of the vehicles.
This is because deep learning combines various sensors without complicated analytical modeling of each individual sensor.
In this study, we structured the multilayer recurrent neural networks (RNN) to improve the accuracy and the stability of the GNSS absolute solutions for the autonomous vehicle navigation.
Specifically, the long short-term memory (LSTM) is an especially useful algorithm for time series data such as navigation with moderate speed of platforms.
From an experiment conducted in a testing area, the LSTM algorithm developed the positioning accuracy by about 40% compared to GNSS-only navigation without any external bias information.
Once the bias is taken care of, the accuracy will significantly be improved up to 8 times better than the GNSS absolute positioning results.
The bias terms of the solution need to be estimated within the model by optimizing the layers as well as the nodes each layer, which should be done in further research.
American Psychological Association (APA)
Kim, Hee-Un& Bae, Tae-Suk. 2019. Deep Learning-Based GNSS Network-Based Real-Time Kinematic Improvement for Autonomous Ground Vehicle Navigation. Journal of Sensors،Vol. 2019, no. 2019, pp.1-8.
https://search.emarefa.net/detail/BIM-1187468
Modern Language Association (MLA)
Kim, Hee-Un& Bae, Tae-Suk. Deep Learning-Based GNSS Network-Based Real-Time Kinematic Improvement for Autonomous Ground Vehicle Navigation. Journal of Sensors No. 2019 (2019), pp.1-8.
https://search.emarefa.net/detail/BIM-1187468
American Medical Association (AMA)
Kim, Hee-Un& Bae, Tae-Suk. Deep Learning-Based GNSS Network-Based Real-Time Kinematic Improvement for Autonomous Ground Vehicle Navigation. Journal of Sensors. 2019. Vol. 2019, no. 2019, pp.1-8.
https://search.emarefa.net/detail/BIM-1187468
Data Type
Journal Articles
Language
English
Notes
Includes bibliographical references
Record ID
BIM-1187468