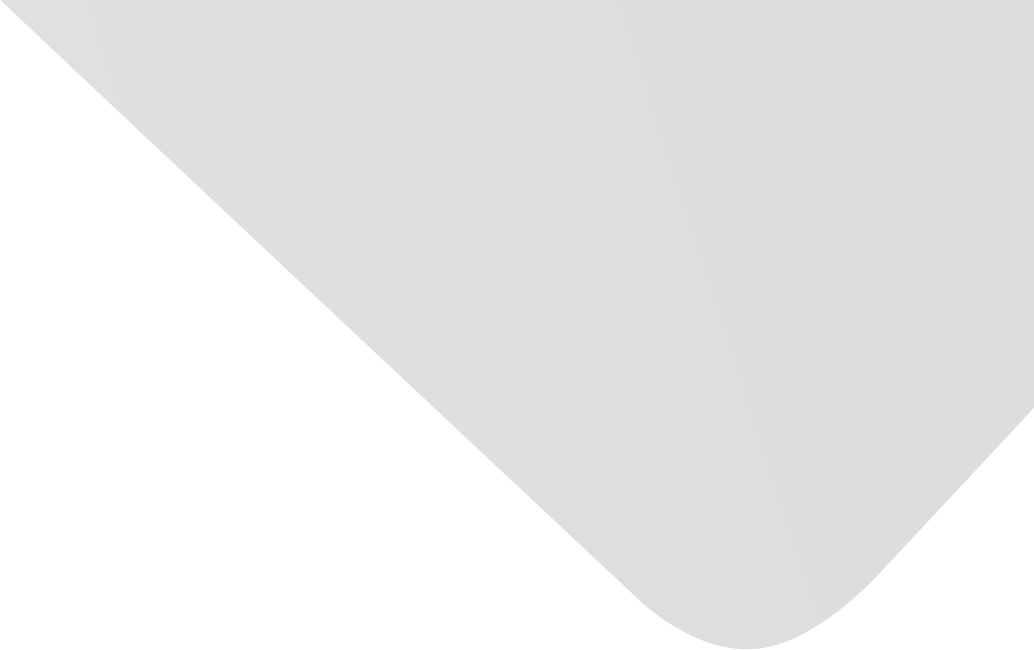
A Framework of Rebalancing Imbalanced Healthcare Data for Rare Events’ Classification: A Case of Look-Alike Sound-Alike Mix-Up Incident Detection
Joint Authors
Tsui, Kwok L.
Zhao, Yang
Wong, Zoie Shui-Yee
Source
Journal of Healthcare Engineering
Issue
Vol. 2018, Issue 2018 (31 Dec. 2018), pp.1-11, 11 p.
Publisher
Hindawi Publishing Corporation
Publication Date
2018-05-22
Country of Publication
Egypt
No. of Pages
11
Main Subjects
Abstract EN
Identifying rare but significant healthcare events in massive unstructured datasets has become a common task in healthcare data analytics.
However, imbalanced class distribution in many practical datasets greatly hampers the detection of rare events, as most classification methods implicitly assume an equal occurrence of classes and are designed to maximize the overall classification accuracy.
In this study, we develop a framework for learning healthcare data with imbalanced distribution via incorporating different rebalancing strategies.
The evaluation results showed that the developed framework can significantly improve the detection accuracy of medical incidents due to look-alike sound-alike (LASA) mix-ups.
Specifically, logistic regression combined with the synthetic minority oversampling technique (SMOTE) produces the best detection results, with a significant 45.3% increase in recall (recall=75.7%) compared with pure logistic regression (recall=52.1%).
American Psychological Association (APA)
Zhao, Yang& Wong, Zoie Shui-Yee& Tsui, Kwok L.. 2018. A Framework of Rebalancing Imbalanced Healthcare Data for Rare Events’ Classification: A Case of Look-Alike Sound-Alike Mix-Up Incident Detection. Journal of Healthcare Engineering،Vol. 2018, no. 2018, pp.1-11.
https://search.emarefa.net/detail/BIM-1187485
Modern Language Association (MLA)
Zhao, Yang…[et al.]. A Framework of Rebalancing Imbalanced Healthcare Data for Rare Events’ Classification: A Case of Look-Alike Sound-Alike Mix-Up Incident Detection. Journal of Healthcare Engineering No. 2018 (2018), pp.1-11.
https://search.emarefa.net/detail/BIM-1187485
American Medical Association (AMA)
Zhao, Yang& Wong, Zoie Shui-Yee& Tsui, Kwok L.. A Framework of Rebalancing Imbalanced Healthcare Data for Rare Events’ Classification: A Case of Look-Alike Sound-Alike Mix-Up Incident Detection. Journal of Healthcare Engineering. 2018. Vol. 2018, no. 2018, pp.1-11.
https://search.emarefa.net/detail/BIM-1187485
Data Type
Journal Articles
Language
English
Notes
Includes bibliographical references
Record ID
BIM-1187485