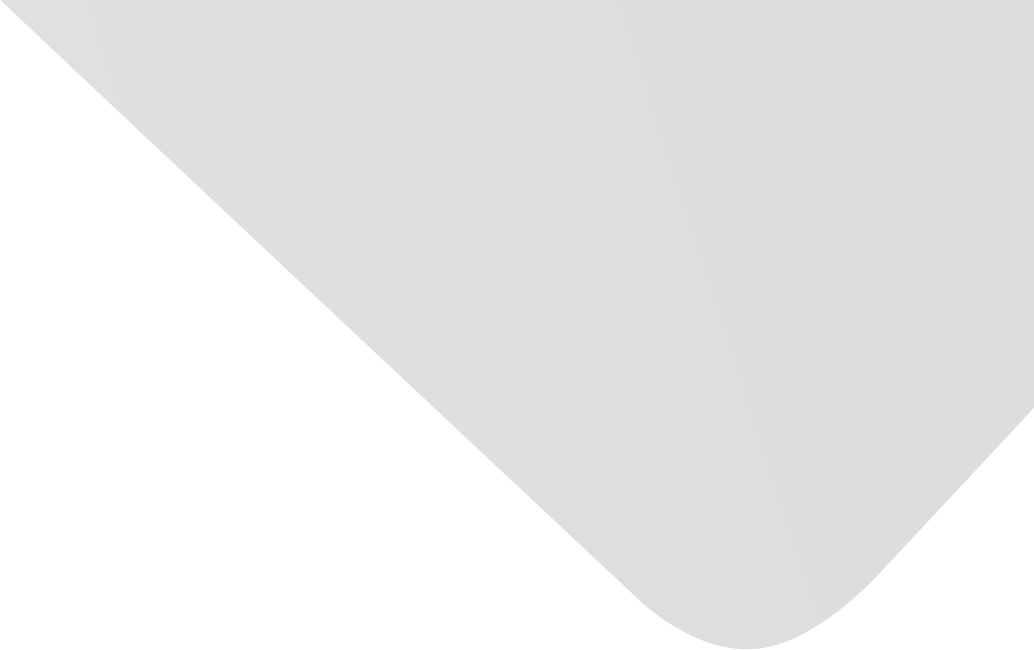
Study on MPGA-BP of Gravity Dam Deformation Prediction
Joint Authors
Wang, Xiaoyu
Yang, Kan
Shen, Changsong
Source
Mathematical Problems in Engineering
Issue
Vol. 2017, Issue 2017 (31 Dec. 2017), pp.1-13, 13 p.
Publisher
Hindawi Publishing Corporation
Publication Date
2017-01-03
Country of Publication
Egypt
No. of Pages
13
Main Subjects
Abstract EN
Displacement is an important physical quantity of hydraulic structures deformation monitoring, and its prediction accuracy is the premise of ensuring the safe operation.
Most existing metaheuristic methods have three problems: (1) falling into local minimum easily, (2) slowing convergence, and (3) the initial value’s sensitivity.
Resolving these three problems and improving the prediction accuracy necessitate the application of genetic algorithm-based backpropagation (GA-BP) neural network and multiple population genetic algorithm (MPGA).
A hybrid multiple population genetic algorithm backpropagation (MPGA-BP) neural network algorithm is put forward to optimize deformation prediction from periodic monitoring surveys of hydraulic structures.
This hybrid model is employed for analyzing the displacement of a gravity dam in China.
The results show the proposed model is superior to an ordinary BP neural network and statistical regression model in the aspect of global search, convergence speed, and prediction accuracy.
American Psychological Association (APA)
Wang, Xiaoyu& Yang, Kan& Shen, Changsong. 2017. Study on MPGA-BP of Gravity Dam Deformation Prediction. Mathematical Problems in Engineering،Vol. 2017, no. 2017, pp.1-13.
https://search.emarefa.net/detail/BIM-1189870
Modern Language Association (MLA)
Wang, Xiaoyu…[et al.]. Study on MPGA-BP of Gravity Dam Deformation Prediction. Mathematical Problems in Engineering No. 2017 (2017), pp.1-13.
https://search.emarefa.net/detail/BIM-1189870
American Medical Association (AMA)
Wang, Xiaoyu& Yang, Kan& Shen, Changsong. Study on MPGA-BP of Gravity Dam Deformation Prediction. Mathematical Problems in Engineering. 2017. Vol. 2017, no. 2017, pp.1-13.
https://search.emarefa.net/detail/BIM-1189870
Data Type
Journal Articles
Language
English
Notes
Includes bibliographical references
Record ID
BIM-1189870