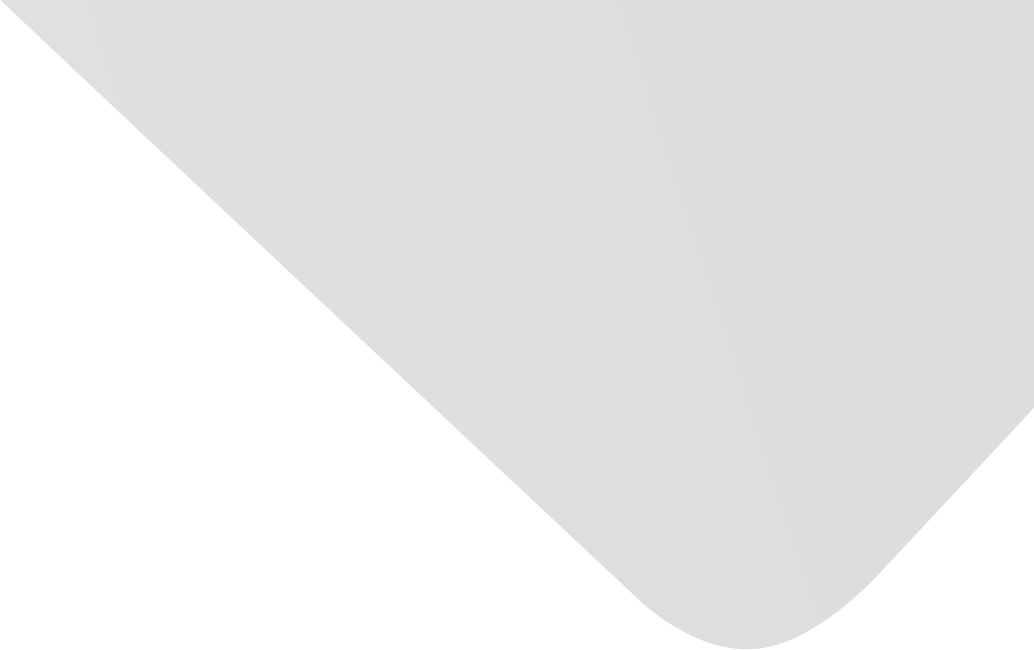
Personalized Recommendation via Suppressing Excessive Diffusion
Joint Authors
Tian, Hui
Zhu, Xuzhen
Chen, Guilin
Yang, Zhao
Source
Mathematical Problems in Engineering
Issue
Vol. 2017, Issue 2017 (31 Dec. 2017), pp.1-10, 10 p.
Publisher
Hindawi Publishing Corporation
Publication Date
2017-06-18
Country of Publication
Egypt
No. of Pages
10
Main Subjects
Abstract EN
Efficient recommendation algorithms are fundamental to solve the problem of information overload in modern society.
In physical dynamics, mass diffusion is a powerful tool to alleviate the long-standing problems of recommendation systems.
However, popularity bias and redundant similarity have not been adequately studied in the literature, which are essentially caused by excessive diffusion and will lead to similarity estimation deviation and recommendation performance degradation.
In this paper, we penalize the popular objects by appropriately dividing the popularity of objects and then leverage the second-order similarity to suppress excessive diffusion.
Evaluation on three real benchmark datasets (MovieLens, Amazon, and RYM) by 10-fold cross-validation demonstrates that our method outperforms the mainstream baselines in accuracy, diversity, and novelty.
American Psychological Association (APA)
Chen, Guilin& Zhu, Xuzhen& Yang, Zhao& Tian, Hui. 2017. Personalized Recommendation via Suppressing Excessive Diffusion. Mathematical Problems in Engineering،Vol. 2017, no. 2017, pp.1-10.
https://search.emarefa.net/detail/BIM-1189872
Modern Language Association (MLA)
Chen, Guilin…[et al.]. Personalized Recommendation via Suppressing Excessive Diffusion. Mathematical Problems in Engineering No. 2017 (2017), pp.1-10.
https://search.emarefa.net/detail/BIM-1189872
American Medical Association (AMA)
Chen, Guilin& Zhu, Xuzhen& Yang, Zhao& Tian, Hui. Personalized Recommendation via Suppressing Excessive Diffusion. Mathematical Problems in Engineering. 2017. Vol. 2017, no. 2017, pp.1-10.
https://search.emarefa.net/detail/BIM-1189872
Data Type
Journal Articles
Language
English
Notes
Includes bibliographical references
Record ID
BIM-1189872