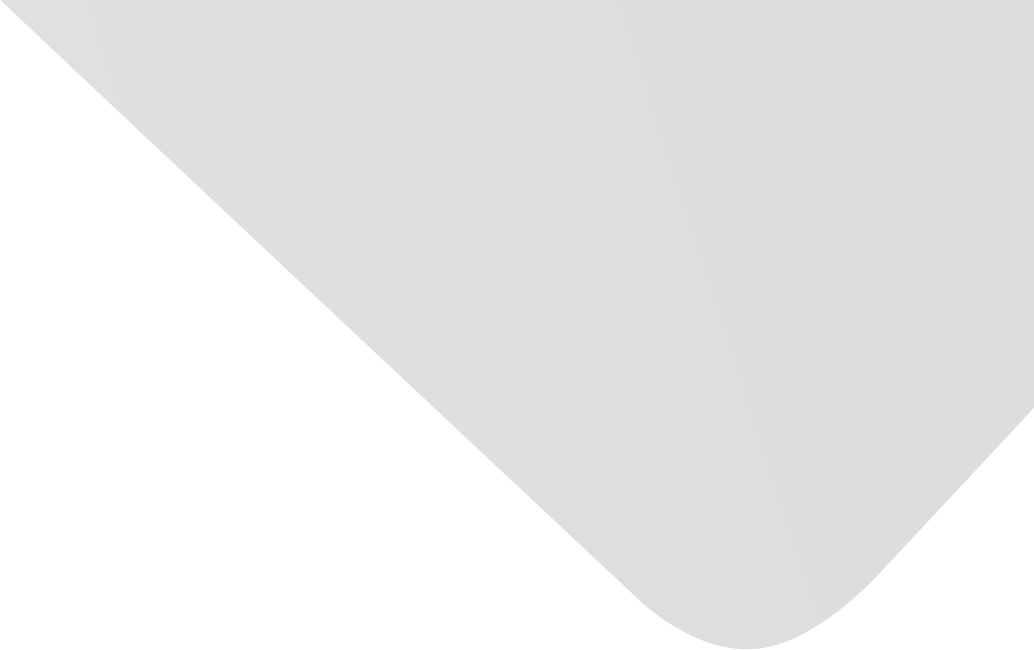
Land Cover Classification Using SegNet with Slope, Aspect, and Multidirectional Shaded Relief Images Derived from Digital Surface Model
Joint Authors
Lee, Dae Geon
Shin, Young Ha
Lee, Dong-Cheon
Source
Issue
Vol. 2020, Issue 2020 (31 Dec. 2020), pp.1-21, 21 p.
Publisher
Hindawi Publishing Corporation
Publication Date
2020-09-12
Country of Publication
Egypt
No. of Pages
21
Main Subjects
Abstract EN
Most object detection, recognition, and classification are performed using optical imagery.
Images are unable to fully represent the real-world due to the limited range of the visible light spectrum reflected light from the surfaces of the objects.
In this regard, physical and geometrical information from other data sources would compensate for the limitation of the optical imagery and bring a synergistic effect for training deep learning (DL) models.
In this paper, we propose to classify terrain features using convolutional neural network (CNN) based SegNet model by utilizing 3D geospatial data including infrared (IR) orthoimages, digital surface model (DSM), and derived information.
The slope, aspect, and shaded relief images (SRIs) were derived from the DSM and were used as training data for the DL model.
The experiments were carried out using the Vaihingen and Potsdam dataset provided by the German Society for Photogrammetry, Remote Sensing and Geoinformation (DGPF) through the International Society for Photogrammetry and Remote Sensing (ISPRS).
The dataset includes IR orthoimages, DSM, airborne LiDAR data, and label data.
The motivation of utilizing 3D data and derived information for training the DL model is that real-world objects are 3D features.
The experimental results demonstrate that the proposed approach of utilizing and integrating various informative feature data could improve the performance of the DL for semantic segmentation.
In particular, the accuracy of building classification is higher compared with other natural objects because derived information could provide geometric characteristics.
Intersection-of-union (IoU) of the buildings for the test data and the new unseen data with combining all derived data were 84.90% and 52.45%, respectively.
American Psychological Association (APA)
Lee, Dae Geon& Shin, Young Ha& Lee, Dong-Cheon. 2020. Land Cover Classification Using SegNet with Slope, Aspect, and Multidirectional Shaded Relief Images Derived from Digital Surface Model. Journal of Sensors،Vol. 2020, no. 2020, pp.1-21.
https://search.emarefa.net/detail/BIM-1190589
Modern Language Association (MLA)
Lee, Dae Geon…[et al.]. Land Cover Classification Using SegNet with Slope, Aspect, and Multidirectional Shaded Relief Images Derived from Digital Surface Model. Journal of Sensors No. 2020 (2020), pp.1-21.
https://search.emarefa.net/detail/BIM-1190589
American Medical Association (AMA)
Lee, Dae Geon& Shin, Young Ha& Lee, Dong-Cheon. Land Cover Classification Using SegNet with Slope, Aspect, and Multidirectional Shaded Relief Images Derived from Digital Surface Model. Journal of Sensors. 2020. Vol. 2020, no. 2020, pp.1-21.
https://search.emarefa.net/detail/BIM-1190589
Data Type
Journal Articles
Language
English
Notes
Includes bibliographical references
Record ID
BIM-1190589