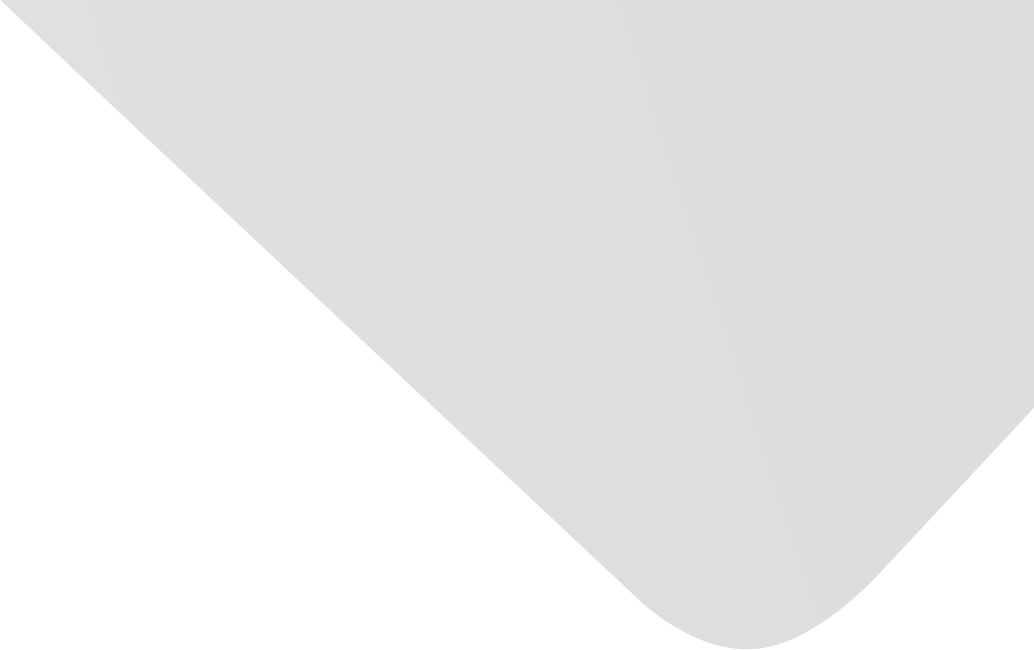
Low-Rank and Sparse Based Deep-Fusion Convolutional Neural Network for Crowd Counting
Joint Authors
Pan, Zhisong
Tang, Siqi
Zhou, Xingyu
Source
Mathematical Problems in Engineering
Issue
Vol. 2017, Issue 2017 (31 Dec. 2017), pp.1-11, 11 p.
Publisher
Hindawi Publishing Corporation
Publication Date
2017-09-25
Country of Publication
Egypt
No. of Pages
11
Main Subjects
Abstract EN
This paper proposes an accurate crowd counting method based on convolutional neural network and low-rank and sparse structure.
To this end, we firstly propose an effective deep-fusion convolutional neural network to promote the density map regression accuracy.
Furthermore, we figure out that most of the existing CNN based crowd counting methods obtain overall counting by direct integral of estimated density map, which limits the accuracy of counting.
Instead of direct integral, we adopt a regression method based on low-rank and sparse penalty to promote accuracy of the projection from density map to global counting.
Experiments demonstrate the importance of such regression process on promoting the crowd counting performance.
The proposed low-rank and sparse based deep-fusion convolutional neural network (LFCNN) outperforms existing crowd counting methods and achieves the state-of-the-art performance.
American Psychological Association (APA)
Tang, Siqi& Pan, Zhisong& Zhou, Xingyu. 2017. Low-Rank and Sparse Based Deep-Fusion Convolutional Neural Network for Crowd Counting. Mathematical Problems in Engineering،Vol. 2017, no. 2017, pp.1-11.
https://search.emarefa.net/detail/BIM-1190607
Modern Language Association (MLA)
Tang, Siqi…[et al.]. Low-Rank and Sparse Based Deep-Fusion Convolutional Neural Network for Crowd Counting. Mathematical Problems in Engineering No. 2017 (2017), pp.1-11.
https://search.emarefa.net/detail/BIM-1190607
American Medical Association (AMA)
Tang, Siqi& Pan, Zhisong& Zhou, Xingyu. Low-Rank and Sparse Based Deep-Fusion Convolutional Neural Network for Crowd Counting. Mathematical Problems in Engineering. 2017. Vol. 2017, no. 2017, pp.1-11.
https://search.emarefa.net/detail/BIM-1190607
Data Type
Journal Articles
Language
English
Notes
Includes bibliographical references
Record ID
BIM-1190607