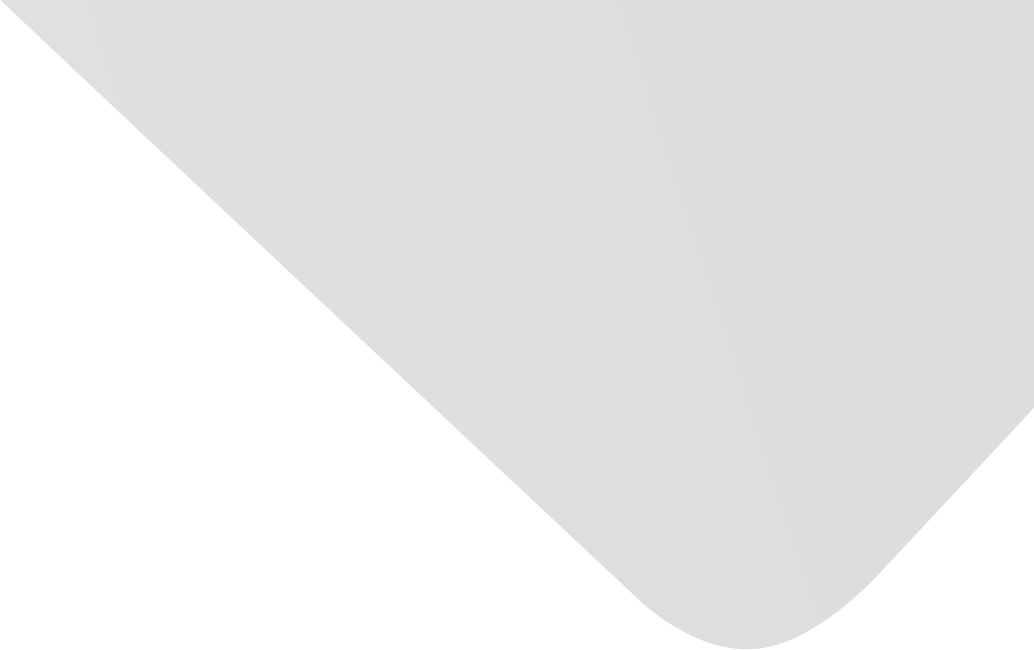
Traffic Data Imputation Algorithm Based on Improved Low-Rank Matrix Decomposition
Joint Authors
Luo, Xianglong
Meng, Xue
Gan, Wenjuan
Chen, Yonghong
Source
Issue
Vol. 2019, Issue 2019 (31 Dec. 2019), pp.1-11, 11 p.
Publisher
Hindawi Publishing Corporation
Publication Date
2019-07-01
Country of Publication
Egypt
No. of Pages
11
Main Subjects
Abstract EN
Traffic data plays a very important role in Intelligent Transportation Systems (ITS).
ITS requires complete traffic data in transportation control, management, guidance, and evaluation.
However, the traffic data collected from many different types of sensors often includes missing data due to sensor damage or data transmission error, which affects the effectiveness and reliability of ITS.
In order to ensure the quality and integrity of traffic flow data, it is very important to propose a satisfying data imputation method.
However, most of the existing imputation methods cannot fully consider the impact of sensor data with data missing and the spatiotemporal correlation characteristics of traffic flow on imputation results.
In this paper, a traffic data imputation method is proposed based on improved low-rank matrix decomposition (ILRMD), which fully considers the influence of missing data and effectively utilizes the spatiotemporal correlation characteristics among traffic data.
The proposed method uses not only the traffic data around the sensor including missing data, but also the sensor data with data missing.
The information of missing data is reflected into the coefficient matrix, and the spatiotemporal correlation characteristics are applied in order to obtain more accurate imputation results.
The real traffic data collected from the Caltrans Performance Measurement System (PeMS) are used to evaluate the imputation performance of the proposed method.
Experiment results show that the average imputation accuracy with proposed method can be improved 87.07% compared with the SVR, ARIMA, KNN, DBN-SVR, WNN, and traditional MC methods, and it is an effective method for data imputation.
American Psychological Association (APA)
Luo, Xianglong& Meng, Xue& Gan, Wenjuan& Chen, Yonghong. 2019. Traffic Data Imputation Algorithm Based on Improved Low-Rank Matrix Decomposition. Journal of Sensors،Vol. 2019, no. 2019, pp.1-11.
https://search.emarefa.net/detail/BIM-1191482
Modern Language Association (MLA)
Luo, Xianglong…[et al.]. Traffic Data Imputation Algorithm Based on Improved Low-Rank Matrix Decomposition. Journal of Sensors No. 2019 (2019), pp.1-11.
https://search.emarefa.net/detail/BIM-1191482
American Medical Association (AMA)
Luo, Xianglong& Meng, Xue& Gan, Wenjuan& Chen, Yonghong. Traffic Data Imputation Algorithm Based on Improved Low-Rank Matrix Decomposition. Journal of Sensors. 2019. Vol. 2019, no. 2019, pp.1-11.
https://search.emarefa.net/detail/BIM-1191482
Data Type
Journal Articles
Language
English
Notes
Includes bibliographical references
Record ID
BIM-1191482