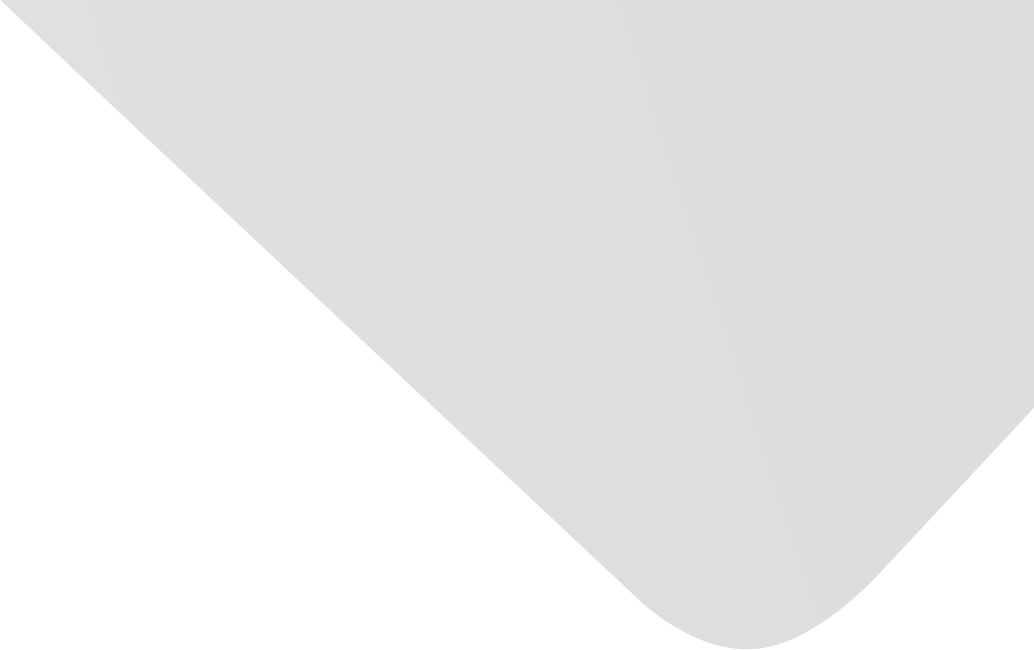
Bearing Fault Diagnosis Based on Improved Locality-Constrained Linear Coding and Adaptive PSO-Optimized SVM
Joint Authors
Yuan, Haodong
Chen, Jin
Dong, Guangming
Source
Mathematical Problems in Engineering
Issue
Vol. 2017, Issue 2017 (31 Dec. 2017), pp.1-16, 16 p.
Publisher
Hindawi Publishing Corporation
Publication Date
2017-08-16
Country of Publication
Egypt
No. of Pages
16
Main Subjects
Abstract EN
A novel bearing fault diagnosis method based on improved locality-constrained linear coding (LLC) and adaptive PSO-optimized support vector machine (SVM) is proposed.
In traditional LLC, each feature is encoded by using a fixed number of bases without considering the distribution of the features and the weight of the bases.
To address these problems, an improved LLC algorithm based on adaptive and weighted bases is proposed.
Firstly, preliminary features are obtained by wavelet packet node energy.
Then, dictionary learning with class-wise K-SVD algorithm is implemented.
Subsequently, based on the learned dictionary the LLC codes can be solved using the improved LLC algorithm.
Finally, SVM optimized by adaptive particle swarm optimization (PSO) is utilized to classify the discriminative LLC codes and thus bearing fault diagnosis is realized.
In the dictionary leaning stage, other methods such as selecting the samples themselves as dictionary and K-means are also conducted for comparison.
The experiment results show that the LLC codes can effectively extract the bearing fault characteristics and the improved LLC outperforms traditional LLC.
The dictionary learned by class-wise K-SVD achieves the best performance.
Additionally, adaptive PSO-optimized SVM can greatly enhance the classification accuracy comparing with SVM using default parameters and linear SVM.
American Psychological Association (APA)
Yuan, Haodong& Chen, Jin& Dong, Guangming. 2017. Bearing Fault Diagnosis Based on Improved Locality-Constrained Linear Coding and Adaptive PSO-Optimized SVM. Mathematical Problems in Engineering،Vol. 2017, no. 2017, pp.1-16.
https://search.emarefa.net/detail/BIM-1191723
Modern Language Association (MLA)
Yuan, Haodong…[et al.]. Bearing Fault Diagnosis Based on Improved Locality-Constrained Linear Coding and Adaptive PSO-Optimized SVM. Mathematical Problems in Engineering No. 2017 (2017), pp.1-16.
https://search.emarefa.net/detail/BIM-1191723
American Medical Association (AMA)
Yuan, Haodong& Chen, Jin& Dong, Guangming. Bearing Fault Diagnosis Based on Improved Locality-Constrained Linear Coding and Adaptive PSO-Optimized SVM. Mathematical Problems in Engineering. 2017. Vol. 2017, no. 2017, pp.1-16.
https://search.emarefa.net/detail/BIM-1191723
Data Type
Journal Articles
Language
English
Notes
Includes bibliographical references
Record ID
BIM-1191723