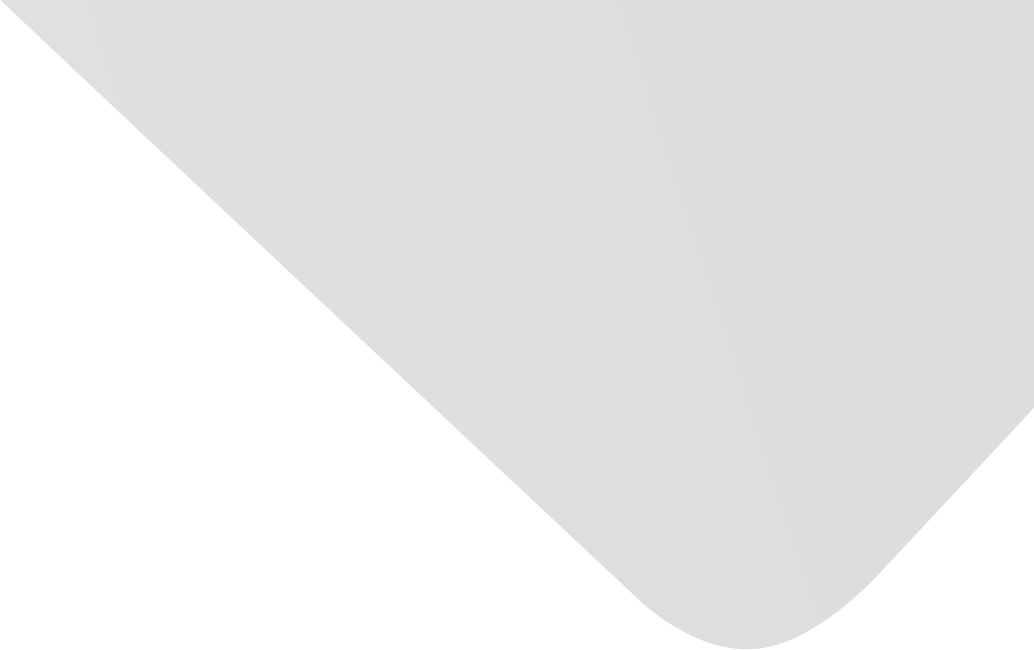
Efficient and Effective Learning of HMMs Based on Identification of Hidden States
Joint Authors
Source
Mathematical Problems in Engineering
Issue
Vol. 2017, Issue 2017 (31 Dec. 2017), pp.1-26, 26 p.
Publisher
Hindawi Publishing Corporation
Publication Date
2017-02-23
Country of Publication
Egypt
No. of Pages
26
Main Subjects
Abstract EN
The predominant learning algorithm for Hidden Markov Models (HMMs) is local search heuristics, of which the Baum-Welch (BW) algorithm is mostly used.
It is an iterative learning procedure starting with a predefined size of state spaces and randomly chosen initial parameters.
However, wrongly chosen initial parameters may cause the risk of falling into a local optimum and a low convergence speed.
To overcome these drawbacks, we propose to use a more suitable model initialization approach, a Segmentation-Clustering and Transient analysis (SCT) framework, to estimate the number of states and model parameters directly from the input data.
Based on an analysis of the information flow through HMMs, we demystify the structure of models and show that high-impact states are directly identifiable from the properties of observation sequences.
States having a high impact on the log-likelihood make HMMs highly specific.
Experimental results show that even though the identification accuracy drops to 87.9% when random models are considered, the SCT method is around 50 to 260 times faster than the BW algorithm with 100% correct identification for highly specific models whose specificity is greater than 0.06.
American Psychological Association (APA)
Liu, Tingting& Lemeire, Jan. 2017. Efficient and Effective Learning of HMMs Based on Identification of Hidden States. Mathematical Problems in Engineering،Vol. 2017, no. 2017, pp.1-26.
https://search.emarefa.net/detail/BIM-1191781
Modern Language Association (MLA)
Liu, Tingting& Lemeire, Jan. Efficient and Effective Learning of HMMs Based on Identification of Hidden States. Mathematical Problems in Engineering No. 2017 (2017), pp.1-26.
https://search.emarefa.net/detail/BIM-1191781
American Medical Association (AMA)
Liu, Tingting& Lemeire, Jan. Efficient and Effective Learning of HMMs Based on Identification of Hidden States. Mathematical Problems in Engineering. 2017. Vol. 2017, no. 2017, pp.1-26.
https://search.emarefa.net/detail/BIM-1191781
Data Type
Journal Articles
Language
English
Notes
Includes bibliographical references
Record ID
BIM-1191781