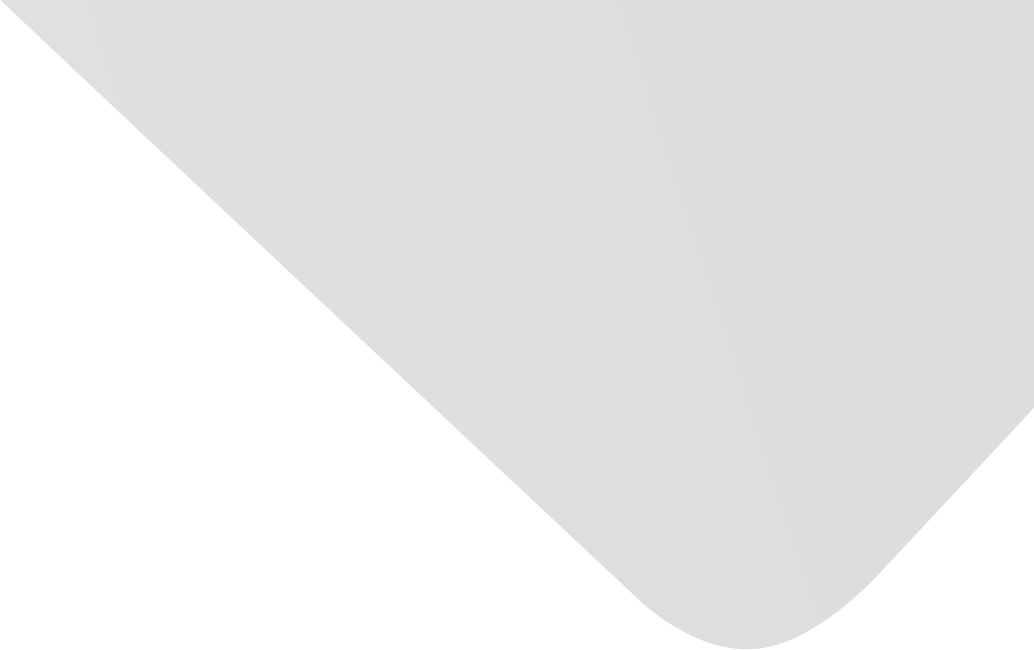
SiameseDenseU-Net-based Semantic Segmentation of Urban Remote Sensing Images
Joint Authors
Dong, Rongsheng
Li, Fengying
Bai, Lulu
Source
Mathematical Problems in Engineering
Issue
Vol. 2020, Issue 2020 (31 Dec. 2020), pp.1-14, 14 p.
Publisher
Hindawi Publishing Corporation
Publication Date
2020-03-23
Country of Publication
Egypt
No. of Pages
14
Main Subjects
Abstract EN
Boundary pixel blur and category imbalance are common problems that occur during semantic segmentation of urban remote sensing images.
Inspired by DenseU-Net, this paper proposes a new end-to-end network—SiameseDenseU-Net.
First, the network simultaneously uses both true orthophoto (TOP) images and their corresponding normalized digital surface model (nDSM) as the input of the network structure.
The deep image features are extracted in parallel by downsampling blocks.
Information such as shallow textures and high-level abstract semantic features are fused throughout the connected channels.
The features extracted by the two parallel processing chains are then fused.
Finally, a softmax layer is used to perform prediction to generate dense label maps.
Experiments on the Vaihingen dataset show that SiameseDenseU-Net improves the F1-score by 8.2% and 7.63% compared with the Hourglass-ShapeNetwork (HSN) model and with the U-Net model.
Regarding the boundary pixels, when using the same focus loss function based on median frequency balance weighting, compared with the original DenseU-Net, the small-target “car” category F1-score of SiameseDenseU-Net improved by 0.92%.
The overall accuracy and the average F1-score also improved to varying degrees.
The proposed SiameseDenseU-Net is better at identifying small-target categories and boundary pixels, and it is numerically and visually superior to the contrast model.
American Psychological Association (APA)
Dong, Rongsheng& Bai, Lulu& Li, Fengying. 2020. SiameseDenseU-Net-based Semantic Segmentation of Urban Remote Sensing Images. Mathematical Problems in Engineering،Vol. 2020, no. 2020, pp.1-14.
https://search.emarefa.net/detail/BIM-1193287
Modern Language Association (MLA)
Dong, Rongsheng…[et al.]. SiameseDenseU-Net-based Semantic Segmentation of Urban Remote Sensing Images. Mathematical Problems in Engineering No. 2020 (2020), pp.1-14.
https://search.emarefa.net/detail/BIM-1193287
American Medical Association (AMA)
Dong, Rongsheng& Bai, Lulu& Li, Fengying. SiameseDenseU-Net-based Semantic Segmentation of Urban Remote Sensing Images. Mathematical Problems in Engineering. 2020. Vol. 2020, no. 2020, pp.1-14.
https://search.emarefa.net/detail/BIM-1193287
Data Type
Journal Articles
Language
English
Notes
Includes bibliographical references
Record ID
BIM-1193287