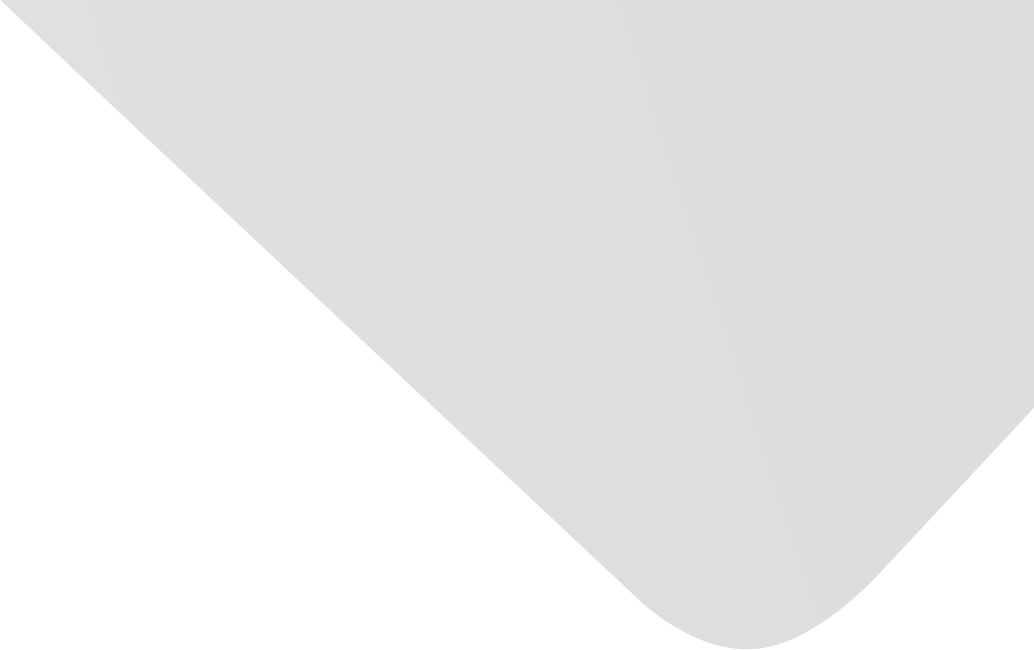
Deep Q-Network with Predictive State Models in Partially Observable Domains
Joint Authors
Yu, Danning
Ni, Kun
Liu, Yunlong
Source
Mathematical Problems in Engineering
Issue
Vol. 2020, Issue 2020 (31 Dec. 2020), pp.1-9, 9 p.
Publisher
Hindawi Publishing Corporation
Publication Date
2020-07-16
Country of Publication
Egypt
No. of Pages
9
Main Subjects
Abstract EN
While deep reinforcement learning (DRL) has achieved great success in some large domains, most of the related algorithms assume that the state of the underlying system is fully observable.
However, many real-world problems are actually partially observable.
For systems with continuous observation, most of the related algorithms, e.g., the deep Q-network (DQN) and deep recurrent Q-network (DRQN), use history observations to represent states; however, they often make computation-expensive and ignore the information of actions.
Predictive state representations (PSRs) can offer a powerful framework for modelling partially observable dynamical systems with discrete or continuous state space, which represents the latent state using completely observable actions and observations.
In this paper, we present a PSR model-based DQN approach which combines the strengths of the PSR model and DQN planning.
We use a recurrent network to establish the recurrent PSR model, which can fully learn dynamics of the partially continuous observable environment.
Then, the model is used for the state representation and update of DQN, which makes DQN no longer rely on a fixed number of history observations or recurrent neural network (RNN) to represent states in the case of partially observable environments.
The strong performance of the proposed approach is demonstrated on a set of robotic control tasks from OpenAI Gym by comparing with the technique with the memory-based DRQN and the state-of-the-art recurrent predictive state policy (RPSP) networks.
Source code is available at https://github.com/RPSR-DQN/paper-code.git.
American Psychological Association (APA)
Yu, Danning& Ni, Kun& Liu, Yunlong. 2020. Deep Q-Network with Predictive State Models in Partially Observable Domains. Mathematical Problems in Engineering،Vol. 2020, no. 2020, pp.1-9.
https://search.emarefa.net/detail/BIM-1193352
Modern Language Association (MLA)
Yu, Danning…[et al.]. Deep Q-Network with Predictive State Models in Partially Observable Domains. Mathematical Problems in Engineering No. 2020 (2020), pp.1-9.
https://search.emarefa.net/detail/BIM-1193352
American Medical Association (AMA)
Yu, Danning& Ni, Kun& Liu, Yunlong. Deep Q-Network with Predictive State Models in Partially Observable Domains. Mathematical Problems in Engineering. 2020. Vol. 2020, no. 2020, pp.1-9.
https://search.emarefa.net/detail/BIM-1193352
Data Type
Journal Articles
Language
English
Notes
Includes bibliographical references
Record ID
BIM-1193352