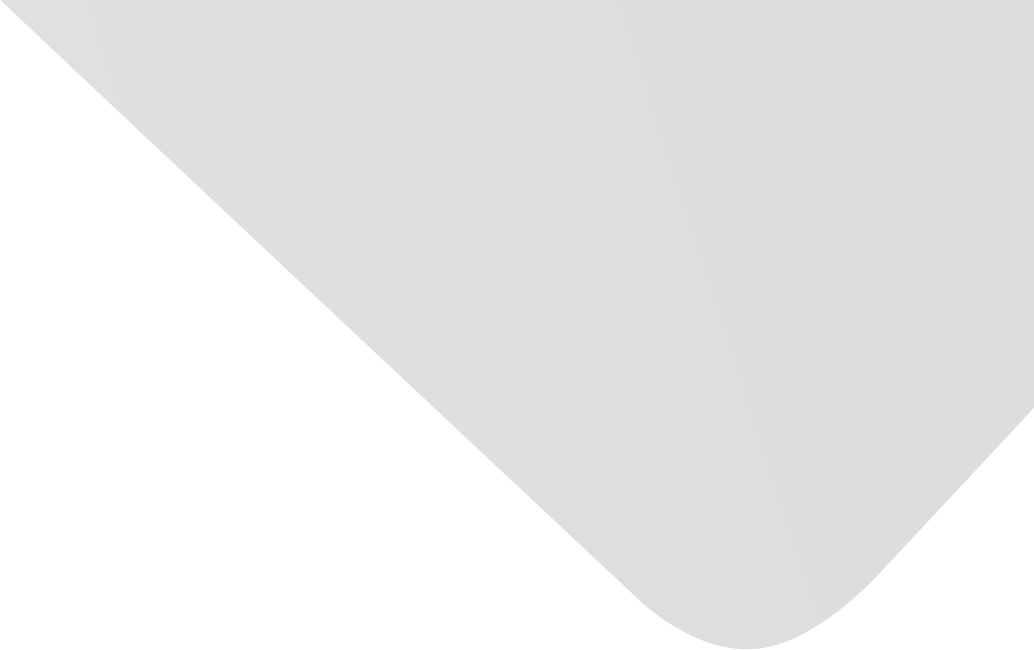
Wear Identification of Vibration Drilling Bit Based on Improved LMD and BP Neural Network
Joint Authors
Shi, Yaochen
Liu, Hongyan
Zhang, Xuechen
Li, Qinghua
Guo, Xiaocheng
Source
Mathematical Problems in Engineering
Issue
Vol. 2020, Issue 2020 (31 Dec. 2020), pp.1-9, 9 p.
Publisher
Hindawi Publishing Corporation
Publication Date
2020-08-14
Country of Publication
Egypt
No. of Pages
9
Main Subjects
Abstract EN
In view of the low accuracy of single signal monitoring for the wear state of vibration drilling bit, a multisignal acquisition system for the wear state of ultrasonic axial vibration drilling bit is built to collect the drilling force, vibration, and acoustic emission signals under three different wear states.
The drilling force, vibration and acoustic emission signals of the bit in the drilling process are processed by using wavelet decomposition technology, and the signals are extracted from the wear state of the bit, The wavelet energy coefficient with high state correlation is used as the feature parameter to identify the bit wear state.
The feature parameter is trained by the combination of noise assisted LMD method and BP neural network.
The experiment of single signal and multisignal fusion monitoring bit wear state is carried out, and the neural network structure is optimized according to the error.
The results show that the accuracy of monitoring bit wear with a single signal of drilling force is 83.3%, the accuracy of monitoring bit wear with a single signal of vibration is 91.6%, the accuracy of monitoring bit wear with a single signal of acoustic emission is 91.6%, and the accuracy of monitoring bit wear with multisignal fusion is 95.8%; when the number of network layer is 4, the vibration is monitored with the fusion of force signal, acoustic emission signal, and vibration signal The accuracy of the state of drilling tool is up to 100%.
The structure model of neural network is optimized reasonably to improve the recognition rate of bit wear in vibration drilling.
American Psychological Association (APA)
Shi, Yaochen& Liu, Hongyan& Zhang, Xuechen& Li, Qinghua& Guo, Xiaocheng. 2020. Wear Identification of Vibration Drilling Bit Based on Improved LMD and BP Neural Network. Mathematical Problems in Engineering،Vol. 2020, no. 2020, pp.1-9.
https://search.emarefa.net/detail/BIM-1193796
Modern Language Association (MLA)
Shi, Yaochen…[et al.]. Wear Identification of Vibration Drilling Bit Based on Improved LMD and BP Neural Network. Mathematical Problems in Engineering No. 2020 (2020), pp.1-9.
https://search.emarefa.net/detail/BIM-1193796
American Medical Association (AMA)
Shi, Yaochen& Liu, Hongyan& Zhang, Xuechen& Li, Qinghua& Guo, Xiaocheng. Wear Identification of Vibration Drilling Bit Based on Improved LMD and BP Neural Network. Mathematical Problems in Engineering. 2020. Vol. 2020, no. 2020, pp.1-9.
https://search.emarefa.net/detail/BIM-1193796
Data Type
Journal Articles
Language
English
Notes
Includes bibliographical references
Record ID
BIM-1193796