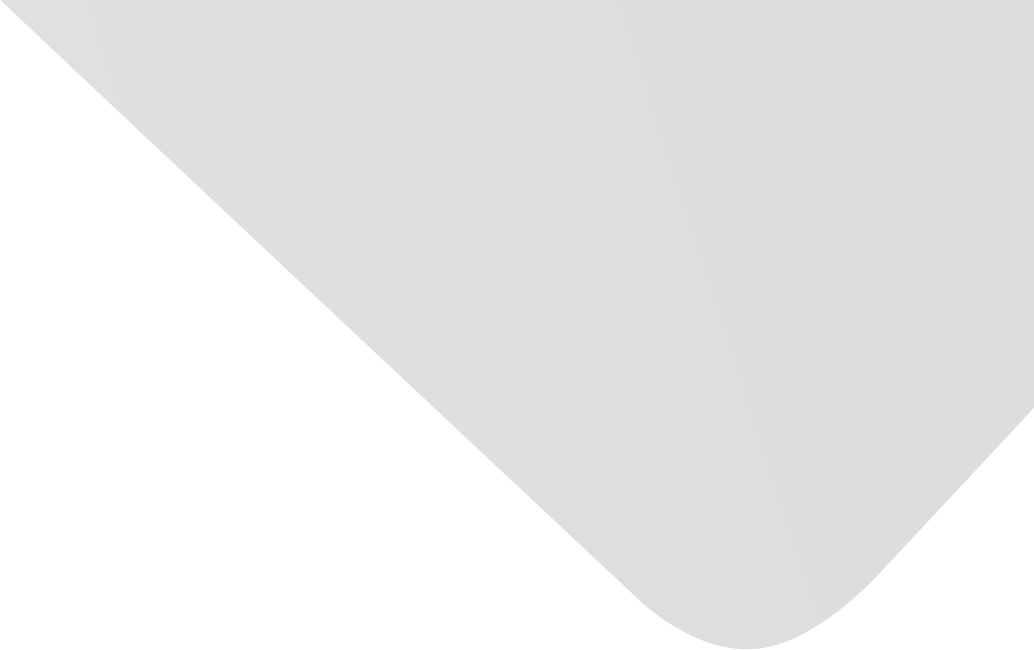
Computer-Assisted Diagnosis for Diabetic Retinopathy Based on Fundus Images Using Deep Convolutional Neural Network
Joint Authors
Chen, Shih-Jen
Li, Yung-Hui
Yeh, Nai-Ning
Chung, Yu-Chien
Source
Issue
Vol. 2019, Issue 2019 (31 Dec. 2019), pp.1-14, 14 p.
Publisher
Hindawi Publishing Corporation
Publication Date
2019-01-02
Country of Publication
Egypt
No. of Pages
14
Main Subjects
Telecommunications Engineering
Abstract EN
Diabetic retinopathy (DR) is a complication of long-standing diabetes, which is hard to detect in its early stage because it only shows a few symptoms.
Nowadays, the diagnosis of DR usually requires taking digital fundus images, as well as images using optical coherence tomography (OCT).
Since OCT equipment is very expensive, it will benefit both the patients and the ophthalmologists if an accurate diagnosis can be made, based solely on reading digital fundus images.
In the paper, we present a novel algorithm based on deep convolutional neural network (DCNN).
Unlike the traditional DCNN approach, we replace the commonly used max-pooling layers with fractional max-pooling.
Two of these DCNNs with a different number of layers are trained to derive more discriminative features for classification.
After combining features from metadata of the image and DCNNs, we train a support vector machine (SVM) classifier to learn the underlying boundary of distributions of each class.
For the experiments, we used the publicly available DR detection database provided by Kaggle.
We used 34,124 training images and 1,000 validation images to build our model and tested with 53,572 testing images.
The proposed DR classifier classifies the stages of DR into five categories, labeled with an integer ranging between zero and four.
The experimental results show that the proposed method can achieve a recognition rate up to 86.17%, which is higher than previously reported in the literature.
In addition to designing a machine learning algorithm, we also develop an app called “Deep Retina.” Equipped with a handheld ophthalmoscope, the average person can take fundus images by themselves and obtain an immediate result, calculated by our algorithm.
It is beneficial for home care, remote medical care, and self-examination.
American Psychological Association (APA)
Li, Yung-Hui& Yeh, Nai-Ning& Chen, Shih-Jen& Chung, Yu-Chien. 2019. Computer-Assisted Diagnosis for Diabetic Retinopathy Based on Fundus Images Using Deep Convolutional Neural Network. Mobile Information Systems،Vol. 2019, no. 2019, pp.1-14.
https://search.emarefa.net/detail/BIM-1193828
Modern Language Association (MLA)
Li, Yung-Hui…[et al.]. Computer-Assisted Diagnosis for Diabetic Retinopathy Based on Fundus Images Using Deep Convolutional Neural Network. Mobile Information Systems No. 2019 (2019), pp.1-14.
https://search.emarefa.net/detail/BIM-1193828
American Medical Association (AMA)
Li, Yung-Hui& Yeh, Nai-Ning& Chen, Shih-Jen& Chung, Yu-Chien. Computer-Assisted Diagnosis for Diabetic Retinopathy Based on Fundus Images Using Deep Convolutional Neural Network. Mobile Information Systems. 2019. Vol. 2019, no. 2019, pp.1-14.
https://search.emarefa.net/detail/BIM-1193828
Data Type
Journal Articles
Language
English
Notes
Includes bibliographical references
Record ID
BIM-1193828