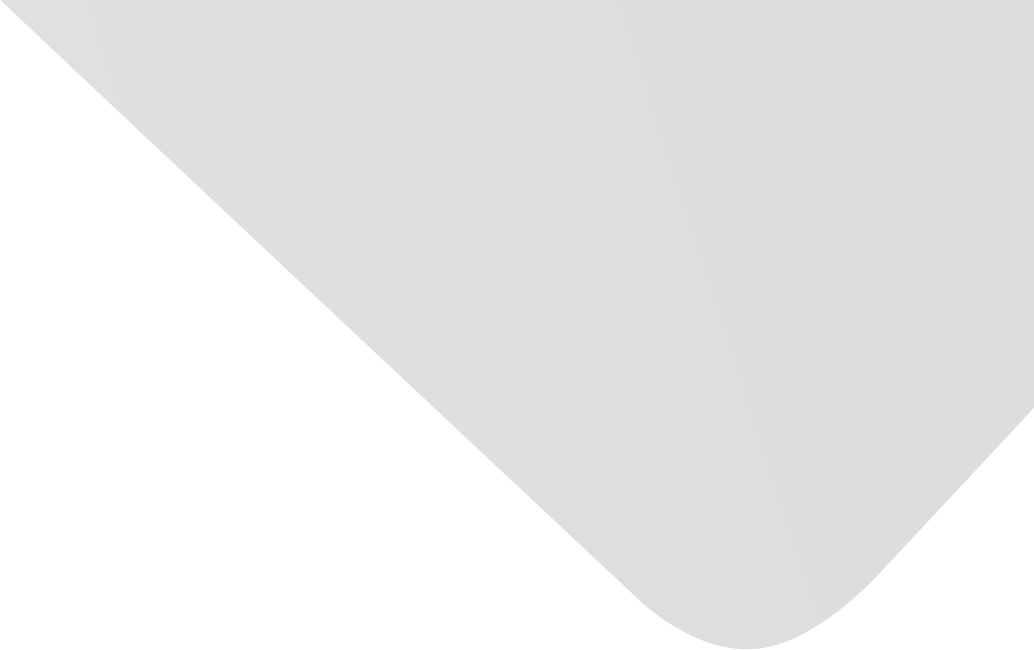
Depression Episodes Detection in Unipolar and Bipolar Patients: A Methodology with Feature Extraction and Feature Selection with Genetic Algorithms Using Activity Motion Signal as Information Source
Joint Authors
Gamboa-Rosales, Hamurabi
Galván-Tejada, Carlos E.
Galván-Tejada, Jorge I.
Zanella-Calzada, Laura A.
Celaya-Padilla, José M.
Magallanes-Quintanar, Rafael
Chávez-Lamas, Nubia M.
Gracia-Cortés, Ma. del Carmen
Source
Issue
Vol. 2019, Issue 2019 (31 Dec. 2019), pp.1-12, 12 p.
Publisher
Hindawi Publishing Corporation
Publication Date
2019-04-23
Country of Publication
Egypt
No. of Pages
12
Main Subjects
Telecommunications Engineering
Abstract EN
Depression is a mental disorder which typically includes recurrent sadness and loss of interest in the enjoyment of the positive aspects of life, and in severe cases fatigue, causing inability to perform daily activities, leading to a progressive loss of quality of life.
Monitoring depression (unipolar and bipolar patients) stats relays on traditional method reports from patients; however, bias is commonly present, given the patients’ interpretation of the experiences.
Nevertheless, to overcome this problem, Ecological Momentary Assessment (EMA) reports have been proposed and widely used.
These reports includes data of the behaviour, feelings, and other type of activities recorded almost in real time using different types of portable devices, which nowadays include smartphones and other wearables such as smartwatches.
In this study is proposed a methodology to detect depressive patients with the motion data generated by patient activity, recorded with a smartband, obtained from the “Depresjon” database.
Using this signal as information source, a feature extraction approach of statistical features, in time and spectral evolution of the signal, is done.
Subsequently, a clever feature selection with a genetic algorithm approach is done to reduce the amount of information required to give a fast noninvasive diagnostic.
Results show that the feature extraction approach can achieve a value of 0.734 of area under the curve (AUC), and after applying feature selection approach, a model comprised by two features from the motion signal can achieve a 0.647 AUC.
These results allow us to conclude that using the activity signal from a smartband, it is possible to distinguish between depressive states, providing a preliminary and automated tool to specialists for the diagnosis of depression almost in real time.
American Psychological Association (APA)
Galván-Tejada, Carlos E.& Zanella-Calzada, Laura A.& Gamboa-Rosales, Hamurabi& Galván-Tejada, Jorge I.& Chávez-Lamas, Nubia M.& Gracia-Cortés, Ma. del Carmen…[et al.]. 2019. Depression Episodes Detection in Unipolar and Bipolar Patients: A Methodology with Feature Extraction and Feature Selection with Genetic Algorithms Using Activity Motion Signal as Information Source. Mobile Information Systems،Vol. 2019, no. 2019, pp.1-12.
https://search.emarefa.net/detail/BIM-1193894
Modern Language Association (MLA)
Galván-Tejada, Carlos E.…[et al.]. Depression Episodes Detection in Unipolar and Bipolar Patients: A Methodology with Feature Extraction and Feature Selection with Genetic Algorithms Using Activity Motion Signal as Information Source. Mobile Information Systems No. 2019 (2019), pp.1-12.
https://search.emarefa.net/detail/BIM-1193894
American Medical Association (AMA)
Galván-Tejada, Carlos E.& Zanella-Calzada, Laura A.& Gamboa-Rosales, Hamurabi& Galván-Tejada, Jorge I.& Chávez-Lamas, Nubia M.& Gracia-Cortés, Ma. del Carmen…[et al.]. Depression Episodes Detection in Unipolar and Bipolar Patients: A Methodology with Feature Extraction and Feature Selection with Genetic Algorithms Using Activity Motion Signal as Information Source. Mobile Information Systems. 2019. Vol. 2019, no. 2019, pp.1-12.
https://search.emarefa.net/detail/BIM-1193894
Data Type
Journal Articles
Language
English
Notes
Includes bibliographical references
Record ID
BIM-1193894