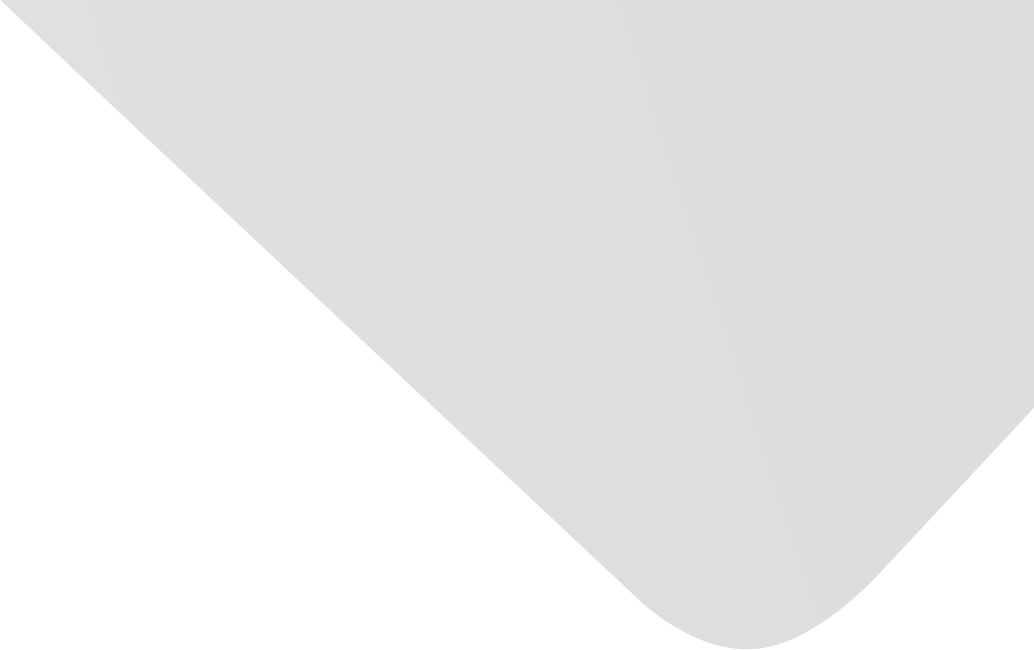
Wasserstein Generative Adversarial Network and Convolutional Neural Network (WG-CNN) for Bearing Fault Diagnosis
Joint Authors
Zuo, Jiankai
Yin, Hang
Li, Zhongzhi
Liu, Hedan
Yang, Kang
Li, Fei
Source
Mathematical Problems in Engineering
Issue
Vol. 2020, Issue 2020 (31 Dec. 2020), pp.1-16, 16 p.
Publisher
Hindawi Publishing Corporation
Publication Date
2020-05-11
Country of Publication
Egypt
No. of Pages
16
Main Subjects
Abstract EN
In recent years, intelligent fault diagnosis technology with deep learning algorithms has been widely used in industry, and they have achieved gratifying results.
Most of these methods require large amount of training data.
However, in actual industrial systems, it is difficult to obtain enough and balanced sample data, which pose challenges in fault identification and classification.
In order to solve the problems, this paper proposes a data generation strategy based on Wasserstein generative adversarial network and convolutional neural network (WG-CNN), which uses generator and discriminator to conduct confrontation training, expands a small sample set into a high-quality dataset, and uses one-dimensional convolutional neural network (1D-CNN) to learn sample characteristics and classify different fault types.
Experimental results over the standard Case Western Reserve University (CWRU) bearing fault diagnosis benchmark dataset showed that the proposed method has obvious and satisfactory fault diagnosis effect with 100% classification accuracy for few-shot learning.
In different noise environments, this method also has excellent performance.
American Psychological Association (APA)
Yin, Hang& Li, Zhongzhi& Zuo, Jiankai& Liu, Hedan& Yang, Kang& Li, Fei. 2020. Wasserstein Generative Adversarial Network and Convolutional Neural Network (WG-CNN) for Bearing Fault Diagnosis. Mathematical Problems in Engineering،Vol. 2020, no. 2020, pp.1-16.
https://search.emarefa.net/detail/BIM-1193974
Modern Language Association (MLA)
Yin, Hang…[et al.]. Wasserstein Generative Adversarial Network and Convolutional Neural Network (WG-CNN) for Bearing Fault Diagnosis. Mathematical Problems in Engineering No. 2020 (2020), pp.1-16.
https://search.emarefa.net/detail/BIM-1193974
American Medical Association (AMA)
Yin, Hang& Li, Zhongzhi& Zuo, Jiankai& Liu, Hedan& Yang, Kang& Li, Fei. Wasserstein Generative Adversarial Network and Convolutional Neural Network (WG-CNN) for Bearing Fault Diagnosis. Mathematical Problems in Engineering. 2020. Vol. 2020, no. 2020, pp.1-16.
https://search.emarefa.net/detail/BIM-1193974
Data Type
Journal Articles
Language
English
Notes
Includes bibliographical references
Record ID
BIM-1193974