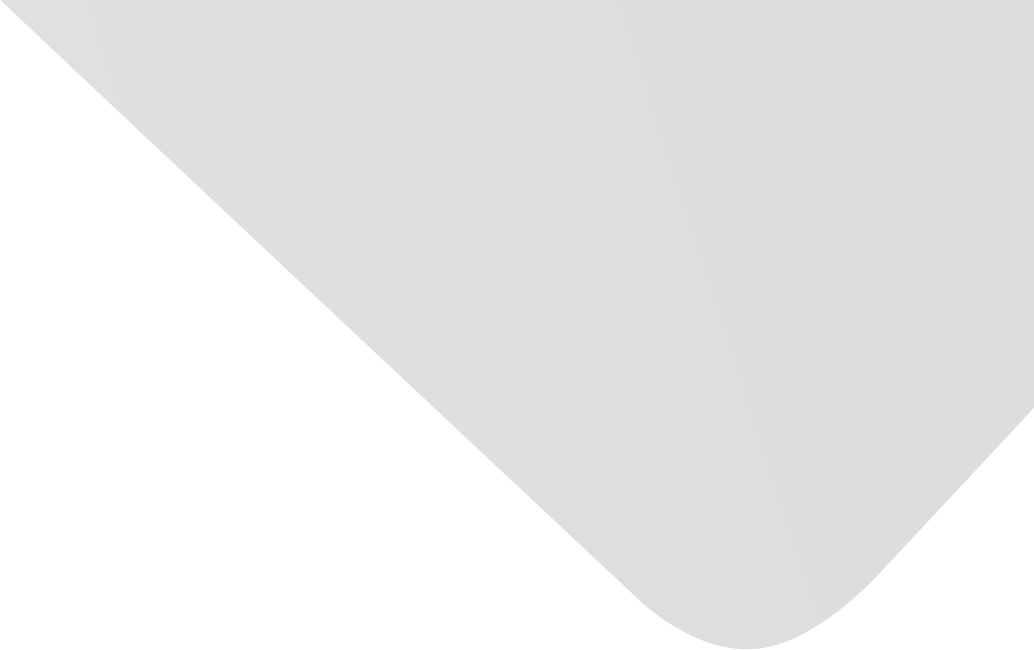
Towards Automatic Image Exposure Level Assessment
Joint Authors
Zhang, Lin
Yang, Xilin
Zhang, Lijun
Liu, Xiao
Zhao, Shengjie
Ma, Yong
Source
Mathematical Problems in Engineering
Issue
Vol. 2020, Issue 2020 (31 Dec. 2020), pp.1-14, 14 p.
Publisher
Hindawi Publishing Corporation
Publication Date
2020-11-23
Country of Publication
Egypt
No. of Pages
14
Main Subjects
Abstract EN
The quality of acquired images can be surely reduced by improper exposures.
Thus, in many vision-related industries, such as imaging sensor manufacturing and video surveillance, an approach that can routinely and accurately evaluate exposure levels of images is in urgent need.
Taking an image as input, such a method is expected to output a scalar value, which can represent the overall perceptual exposure level of the examined image, ranging from extremely underexposed to extremely overexposed.
However, studies focusing on image exposure level assessment (IELA) are quite sporadic.
It should be noted that blind NR-IQA (no-reference image quality assessment) algorithms or metrics used to measure the quality of contrast-distorted images cannot be used for IELA.
The root reason is that though these algorithms can quantify quality distortion of images, they do not know whether the distortion is due to underexposure or overexposure.
This paper aims to resolve the issue of IELA to some extent and contributes to two aspects.
Firstly, an Image Exposure Database (IEpsD) is constructed to facilitate the study of IELA.
IEpsD comprises 24,500 images with various exposure levels, and for each image a subjective exposure score is provided, which represents its perceptual exposure level.
Secondly, as IELA can be naturally formulated as a regression problem, we thoroughly evaluate the performance of modern deep CNN architectures for solving this specific task.
Our evaluation results can serve as a baseline when the other researchers develop even more sophisticated IELA approaches.
To facilitate the other researchers to reproduce our results, we have released the dataset and the relevant source code at https://cslinzhang.github.io/imgExpo/.
American Psychological Association (APA)
Zhang, Lin& Yang, Xilin& Zhang, Lijun& Liu, Xiao& Zhao, Shengjie& Ma, Yong. 2020. Towards Automatic Image Exposure Level Assessment. Mathematical Problems in Engineering،Vol. 2020, no. 2020, pp.1-14.
https://search.emarefa.net/detail/BIM-1194030
Modern Language Association (MLA)
Zhang, Lin…[et al.]. Towards Automatic Image Exposure Level Assessment. Mathematical Problems in Engineering No. 2020 (2020), pp.1-14.
https://search.emarefa.net/detail/BIM-1194030
American Medical Association (AMA)
Zhang, Lin& Yang, Xilin& Zhang, Lijun& Liu, Xiao& Zhao, Shengjie& Ma, Yong. Towards Automatic Image Exposure Level Assessment. Mathematical Problems in Engineering. 2020. Vol. 2020, no. 2020, pp.1-14.
https://search.emarefa.net/detail/BIM-1194030
Data Type
Journal Articles
Language
English
Notes
Includes bibliographical references
Record ID
BIM-1194030