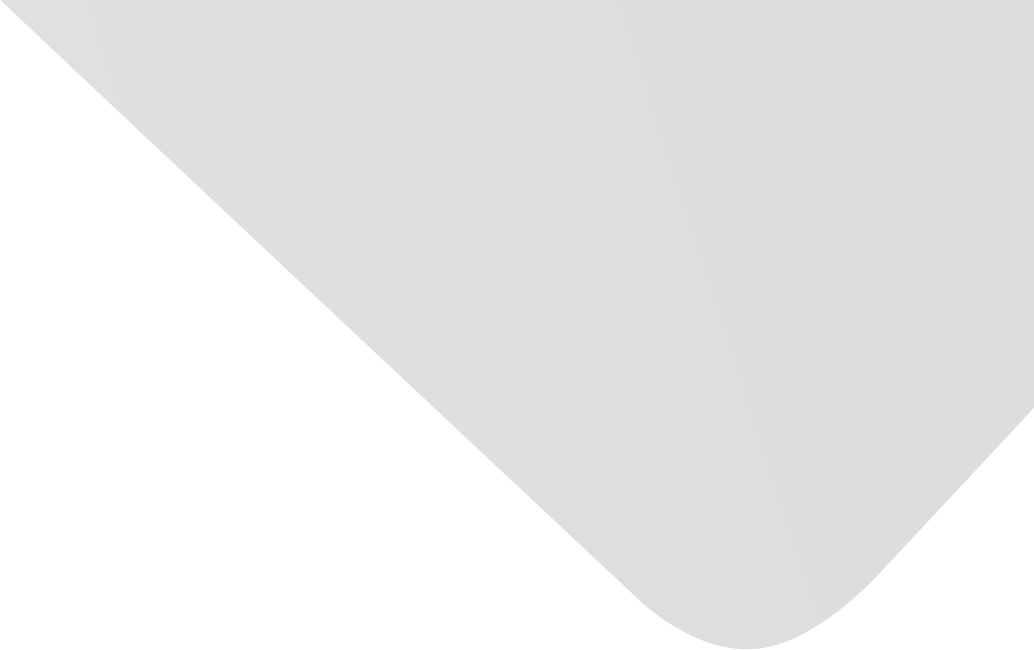
A Multichannel LSTM-CNN Method for Fault Diagnosis of Chemical Process
Joint Authors
Shao, Bilin
Hu, Xiaoli
Bian, Genqing
Zhao, Yu
Source
Mathematical Problems in Engineering
Issue
Vol. 2019, Issue 2019 (31 Dec. 2019), pp.1-14, 14 p.
Publisher
Hindawi Publishing Corporation
Publication Date
2019-12-05
Country of Publication
Egypt
No. of Pages
14
Main Subjects
Abstract EN
The identification and classification of faults in chemical processes can provide decision basis for equipment maintenance personnel to ensure the safe operation of the production process.
In this paper, we combine long short-term memory neural network (LSTM) with convolutional neural network (CNN) and propose a new fault diagnosis method based on multichannel LSTM-CNN (MCLSTM-CNN).
The primary methodology here includes three aspects.
In the initial state, the fault data are input into the LSTM to obtain the output of the hidden layer, which stores the relevant temporal and spatial domain information.
Due to the diversity of data features, convolutional kernels with different sizes are utilized to form multiple channels to extract the output characteristics of the hidden layer simultaneously.
Finally, the fault data are classified by fully connected layers.
The Tennessee Eastman (TE) chemical process is used for experimental analysis, and the MCLSTM-CNN model is compared with the LSTM-CNN, LSTM, CNN, RF and KPCA + SVM models.
The experimental results show that the MCLSTM-CNN model has higher diagnostic accuracy, and the fault classification results are superior to other models.
American Psychological Association (APA)
Shao, Bilin& Hu, Xiaoli& Bian, Genqing& Zhao, Yu. 2019. A Multichannel LSTM-CNN Method for Fault Diagnosis of Chemical Process. Mathematical Problems in Engineering،Vol. 2019, no. 2019, pp.1-14.
https://search.emarefa.net/detail/BIM-1194199
Modern Language Association (MLA)
Shao, Bilin…[et al.]. A Multichannel LSTM-CNN Method for Fault Diagnosis of Chemical Process. Mathematical Problems in Engineering No. 2019 (2019), pp.1-14.
https://search.emarefa.net/detail/BIM-1194199
American Medical Association (AMA)
Shao, Bilin& Hu, Xiaoli& Bian, Genqing& Zhao, Yu. A Multichannel LSTM-CNN Method for Fault Diagnosis of Chemical Process. Mathematical Problems in Engineering. 2019. Vol. 2019, no. 2019, pp.1-14.
https://search.emarefa.net/detail/BIM-1194199
Data Type
Journal Articles
Language
English
Notes
Includes bibliographical references
Record ID
BIM-1194199