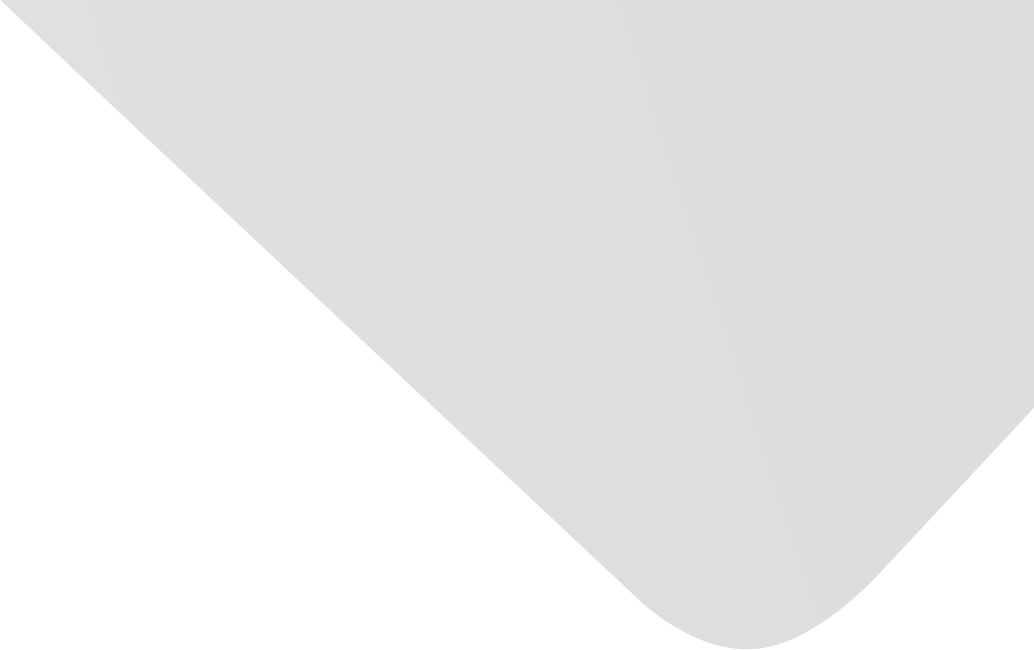
Time Series Prediction of Electricity Demand Using Adaptive Neuro-Fuzzy Inference Systems
Joint Authors
Acakpovi, Amevi
Ternor, Alfred Tettey
Asabere, Nana Yaw
Adjei, Patrick
Iddrisu, Abdul-Shakud
Source
Mathematical Problems in Engineering
Issue
Vol. 2020, Issue 2020 (31 Dec. 2020), pp.1-14, 14 p.
Publisher
Hindawi Publishing Corporation
Publication Date
2020-08-08
Country of Publication
Egypt
No. of Pages
14
Main Subjects
Abstract EN
This paper is concerned with the reliable prediction of electricity demands using the Adaptive Neuro-Fuzzy Inference System (ANFIS).
The need for electricity demand prediction is fundamental and vital for power resource planning and monitoring.
A dataset of electricity demands covering the period of 2003 to 2018 was collected from the Electricity Distribution Company of Ghana, covering three urban areas namely Mallam, Achimota, and Ga East, all in Ghana.
The dataset was divided into two parts: one part covering a period of 0 to 500 hours was used for training of the ANFIS algorithm while the second part was used for validation.
Three scenarios were considered for the simulation exercise that was done with the MATLAB software.
Scenario one considered four inputs sampled data, scenario two considered an additional input making it 5, and scenario 3 was similar to scenario 1 with the exception of the number of membership functions that increased from 2 to 3.
The performance of the ANFIS algorithm was assessed by comparing its predictions with other three forecast models namely Support Vector Regression (SVR), Least Square Support Vector Machine (LS-SVM), and Auto-Regressive Integrated Moving Average (ARIMA).
Findings revealed that the ANFIS algorithm can perform the prediction accurately, the ANFIS algorithm converges faster with an increase in the data used for training, and increasing the membership function resulted in overfitting of data which adversely affected the RMSE values.
Comparison of the ANFIS results to other previously used methods of predicting electricity demands including SVR, LS-SVM, and ARIMA revealed that there is merit to the potentials of the ANFIS algorithm for improved predictive accuracy while relying on a quality data for training and reliable setting of tuning parameters.
American Psychological Association (APA)
Acakpovi, Amevi& Ternor, Alfred Tettey& Asabere, Nana Yaw& Adjei, Patrick& Iddrisu, Abdul-Shakud. 2020. Time Series Prediction of Electricity Demand Using Adaptive Neuro-Fuzzy Inference Systems. Mathematical Problems in Engineering،Vol. 2020, no. 2020, pp.1-14.
https://search.emarefa.net/detail/BIM-1195015
Modern Language Association (MLA)
Acakpovi, Amevi…[et al.]. Time Series Prediction of Electricity Demand Using Adaptive Neuro-Fuzzy Inference Systems. Mathematical Problems in Engineering No. 2020 (2020), pp.1-14.
https://search.emarefa.net/detail/BIM-1195015
American Medical Association (AMA)
Acakpovi, Amevi& Ternor, Alfred Tettey& Asabere, Nana Yaw& Adjei, Patrick& Iddrisu, Abdul-Shakud. Time Series Prediction of Electricity Demand Using Adaptive Neuro-Fuzzy Inference Systems. Mathematical Problems in Engineering. 2020. Vol. 2020, no. 2020, pp.1-14.
https://search.emarefa.net/detail/BIM-1195015
Data Type
Journal Articles
Language
English
Notes
Includes bibliographical references
Record ID
BIM-1195015