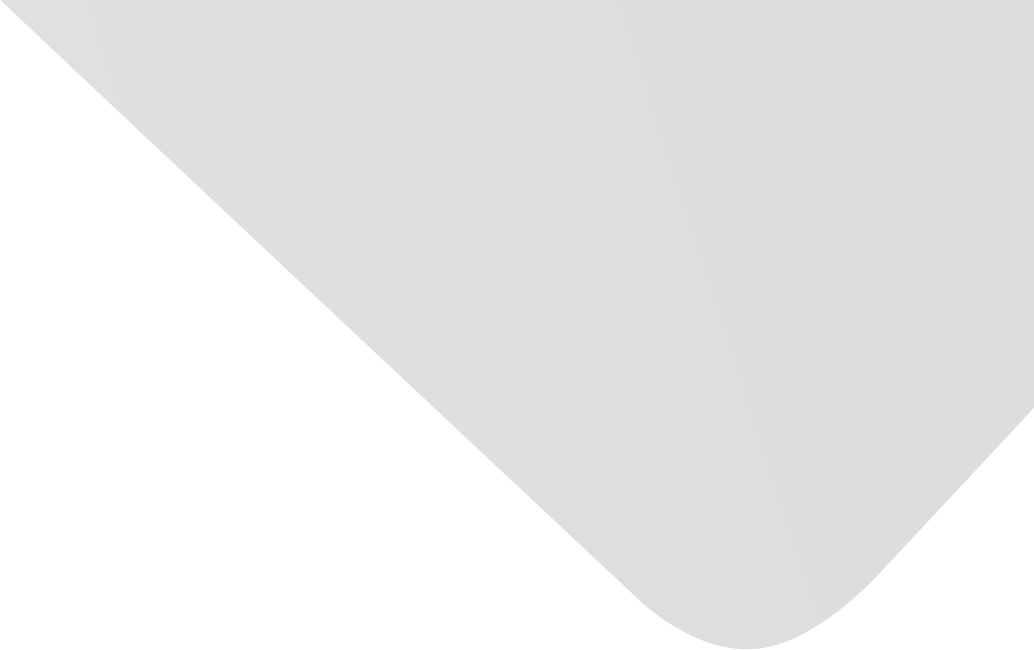
Deep Ensemble Reinforcement Learning with Multiple Deep Deterministic Policy Gradient Algorithm
Joint Authors
Source
Mathematical Problems in Engineering
Issue
Vol. 2020, Issue 2020 (31 Dec. 2020), pp.1-12, 12 p.
Publisher
Hindawi Publishing Corporation
Publication Date
2020-01-22
Country of Publication
Egypt
No. of Pages
12
Main Subjects
Abstract EN
Deep deterministic policy gradient algorithm operating over continuous space of actions has attracted great attention for reinforcement learning.
However, the exploration strategy through dynamic programming within the Bayesian belief state space is rather inefficient even for simple systems.
Another problem is the sequential and iterative training data with autonomous vehicles subject to the law of causality, which is against the i.i.d.
(independent identically distributed) data assumption of the training samples.
This usually results in failure of the standard bootstrap when learning an optimal policy.
In this paper, we propose a framework of m-out-of-n bootstrapped and aggregated multiple deep deterministic policy gradient to accelerate the training process and increase the performance.
Experiment results on the 2D robot arm game show that the reward gained by the aggregated policy is 10%–50% better than those gained by subpolicies.
Experiment results on the open racing car simulator (TORCS) demonstrate that the new algorithm can learn successful control policies with less training time by 56.7%.
Analysis on convergence is also given from the perspective of probability and statistics.
These results verify that the proposed method outperforms the existing algorithms in both efficiency and performance.
American Psychological Association (APA)
Wu, Junta& Li, Huiyun. 2020. Deep Ensemble Reinforcement Learning with Multiple Deep Deterministic Policy Gradient Algorithm. Mathematical Problems in Engineering،Vol. 2020, no. 2020, pp.1-12.
https://search.emarefa.net/detail/BIM-1195068
Modern Language Association (MLA)
Wu, Junta& Li, Huiyun. Deep Ensemble Reinforcement Learning with Multiple Deep Deterministic Policy Gradient Algorithm. Mathematical Problems in Engineering No. 2020 (2020), pp.1-12.
https://search.emarefa.net/detail/BIM-1195068
American Medical Association (AMA)
Wu, Junta& Li, Huiyun. Deep Ensemble Reinforcement Learning with Multiple Deep Deterministic Policy Gradient Algorithm. Mathematical Problems in Engineering. 2020. Vol. 2020, no. 2020, pp.1-12.
https://search.emarefa.net/detail/BIM-1195068
Data Type
Journal Articles
Language
English
Notes
Includes bibliographical references
Record ID
BIM-1195068