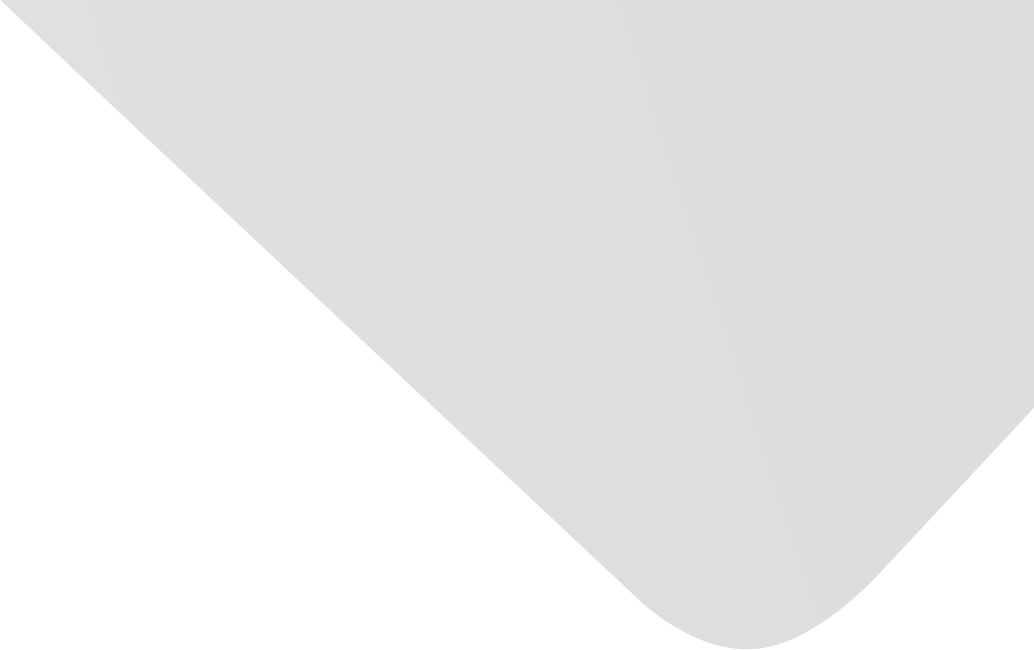
A Multimodal Music Emotion Classification Method Based on Multifeature Combined Network Classifier
Joint Authors
Source
Mathematical Problems in Engineering
Issue
Vol. 2020, Issue 2020 (31 Dec. 2020), pp.1-11, 11 p.
Publisher
Hindawi Publishing Corporation
Publication Date
2020-08-01
Country of Publication
Egypt
No. of Pages
11
Main Subjects
Abstract EN
Aiming at the shortcomings of single network classification model, this paper applies CNN-LSTM (convolutional neural networks-long short-term memory) combined network in the field of music emotion classification and proposes a multifeature combined network classifier based on CNN-LSTM which combines 2D (two-dimensional) feature input through CNN-LSTM and 1D (single-dimensional) feature input through DNN (deep neural networks) to make up for the deficiencies of original single feature models.
The model uses multiple convolution kernels in CNN for 2D feature extraction, BiLSTM (bidirectional LSTM) for serialization processing and is used, respectively, for audio and lyrics single-modal emotion classification output.
In the audio feature extraction, music audio is finely divided and the human voice is separated to obtain pure background sound clips; the spectrogram and LLDs (Low Level Descriptors) are extracted therefrom.
In the lyrics feature extraction, the chi-squared test vector and word embedding extracted by Word2vec are, respectively, used as the feature representation of the lyrics.
Combining the two types of heterogeneous features selected by audio and lyrics through the classification model can improve the classification performance.
In order to fuse the emotional information of the two modals of music audio and lyrics, this paper proposes a multimodal ensemble learning method based on stacking, which is different from existing feature-level and decision-level fusion methods, the method avoids information loss caused by direct dimensionality reduction, and the original features are converted into label results for fusion, effectively solving the problem of feature heterogeneity.
Experiments on million song dataset show that the audio classification accuracy of the multifeature combined network classifier in this paper reaches 68%, and the lyrics classification accuracy reaches 74%.
The average classification accuracy of the multimodal reaches 78%, which is significantly improved compared with the single-modal.
American Psychological Association (APA)
Chen, Changfeng& Li, Qiang. 2020. A Multimodal Music Emotion Classification Method Based on Multifeature Combined Network Classifier. Mathematical Problems in Engineering،Vol. 2020, no. 2020, pp.1-11.
https://search.emarefa.net/detail/BIM-1195283
Modern Language Association (MLA)
Chen, Changfeng& Li, Qiang. A Multimodal Music Emotion Classification Method Based on Multifeature Combined Network Classifier. Mathematical Problems in Engineering No. 2020 (2020), pp.1-11.
https://search.emarefa.net/detail/BIM-1195283
American Medical Association (AMA)
Chen, Changfeng& Li, Qiang. A Multimodal Music Emotion Classification Method Based on Multifeature Combined Network Classifier. Mathematical Problems in Engineering. 2020. Vol. 2020, no. 2020, pp.1-11.
https://search.emarefa.net/detail/BIM-1195283
Data Type
Journal Articles
Language
English
Notes
Includes bibliographical references
Record ID
BIM-1195283