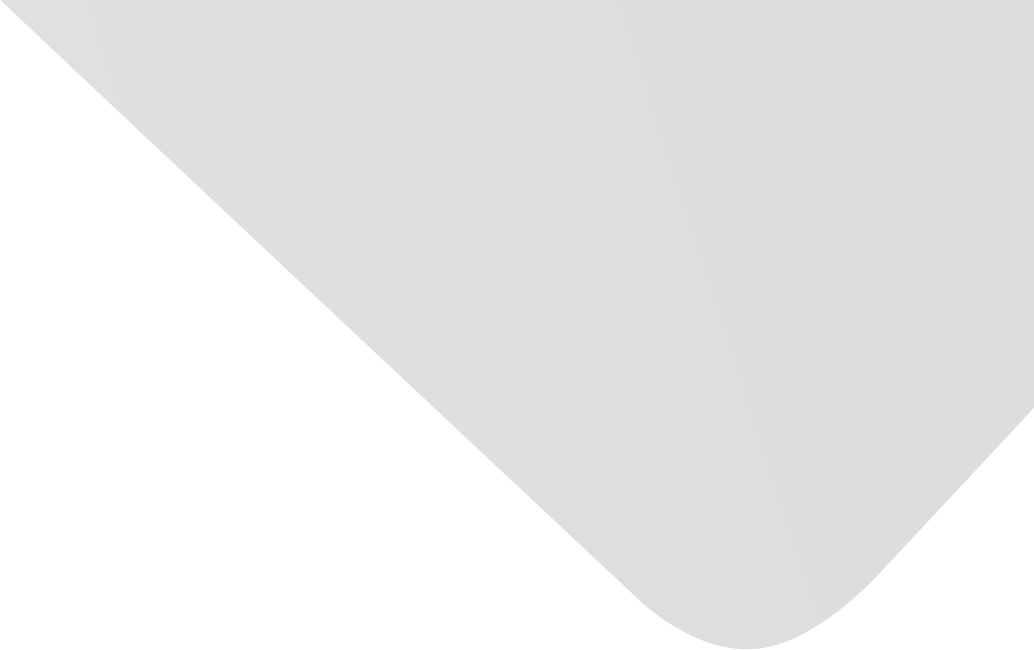
Exact k-Component Graph Learning for Image Clustering
Joint Authors
Source
Mathematical Problems in Engineering
Issue
Vol. 2020, Issue 2020 (31 Dec. 2020), pp.1-10, 10 p.
Publisher
Hindawi Publishing Corporation
Publication Date
2020-08-06
Country of Publication
Egypt
No. of Pages
10
Main Subjects
Abstract EN
The performance of graph-based clustering methods highly depends on the quality of the data affinity graph as a good affinity graph can approximate well the pairwise similarity between data samples.
To a large extent, existing graph-based clustering methods construct the affinity graph based on a fixed distance metric, which is often not an accurate representation of the underlying data structure.
Also, they require postprocessing on the affinity graph to obtain clustering results.
Thus, the results are sensitive to the particular graph construction methods.
To address these two drawbacks, we propose a k-component graph clustering (k-GC) approach to learn an intrinsic affinity graph and to obtain clustering results simultaneously.
Specifically, k-GC learns the data affinity graph by assigning the adaptive and optimal neighbors for each data point based on the local distances.
Efficient iterative updating algorithms are derived for k-GC, along with proofs of convergence.
Experiments on several benchmark datasets have demonstrated the effectiveness of k-GC.
American Psychological Association (APA)
Min, Yufang& Zhang, Yaonan. 2020. Exact k-Component Graph Learning for Image Clustering. Mathematical Problems in Engineering،Vol. 2020, no. 2020, pp.1-10.
https://search.emarefa.net/detail/BIM-1195728
Modern Language Association (MLA)
Min, Yufang& Zhang, Yaonan. Exact k-Component Graph Learning for Image Clustering. Mathematical Problems in Engineering No. 2020 (2020), pp.1-10.
https://search.emarefa.net/detail/BIM-1195728
American Medical Association (AMA)
Min, Yufang& Zhang, Yaonan. Exact k-Component Graph Learning for Image Clustering. Mathematical Problems in Engineering. 2020. Vol. 2020, no. 2020, pp.1-10.
https://search.emarefa.net/detail/BIM-1195728
Data Type
Journal Articles
Language
English
Notes
Includes bibliographical references
Record ID
BIM-1195728