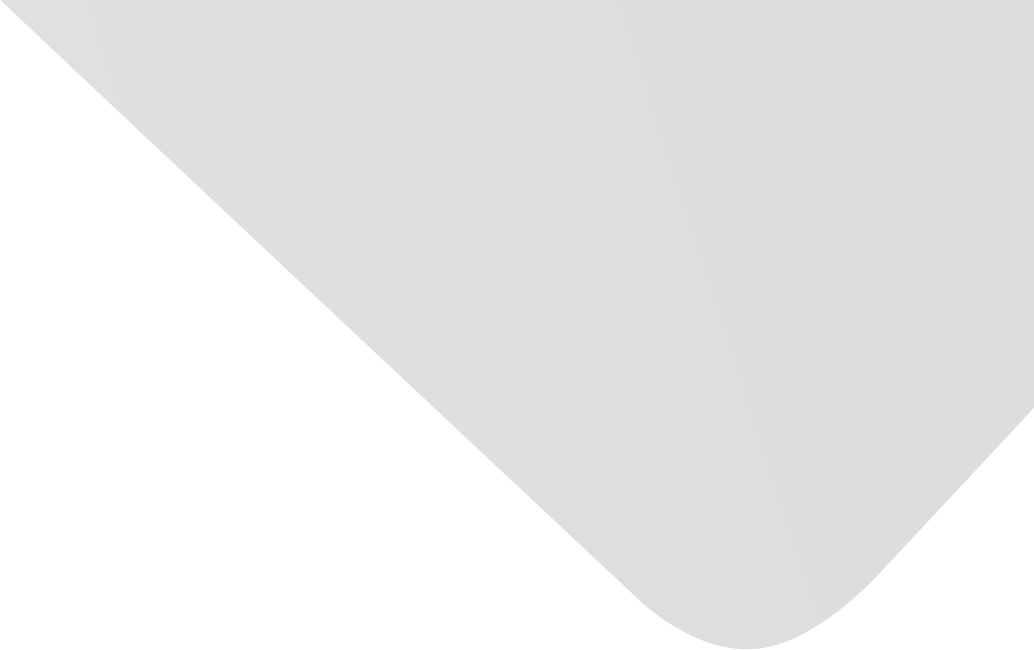
A Study of Continuous Maximum Entropy Deep Inverse Reinforcement Learning
Joint Authors
Chen, Xi-liang
Cao, Lei
Xu, Zhi-xiong
Lai, Jun
Li, Chen-xi
Source
Mathematical Problems in Engineering
Issue
Vol. 2019, Issue 2019 (31 Dec. 2019), pp.1-8, 8 p.
Publisher
Hindawi Publishing Corporation
Publication Date
2019-04-08
Country of Publication
Egypt
No. of Pages
8
Main Subjects
Abstract EN
The assumption of IRL is that demonstrations are optimally acting in an environment.
In the past, most of the work on IRL needed to calculate optimal policies for different reward functions.
However, this requirement is difficult to satisfy in large or continuous state space tasks.
Let alone continuous action space.
We propose a continuous maximum entropy deep inverse reinforcement learning algorithm for continuous state space and continues action space, which realizes the depth cognition of the environment model by the way of reconstructing the reward function based on the demonstrations, and a hot start mechanism based on demonstrations to make the training process faster and better.
We compare this new approach to well-known IRL algorithms using Maximum Entropy IRL, DDPG, hot start DDPG, etc.
Empirical results on classical control environments on OpenAI Gym: MountainCarContinues-v0 show that our approach is able to learn policies faster and better.
American Psychological Association (APA)
Chen, Xi-liang& Cao, Lei& Xu, Zhi-xiong& Lai, Jun& Li, Chen-xi. 2019. A Study of Continuous Maximum Entropy Deep Inverse Reinforcement Learning. Mathematical Problems in Engineering،Vol. 2019, no. 2019, pp.1-8.
https://search.emarefa.net/detail/BIM-1195770
Modern Language Association (MLA)
Chen, Xi-liang…[et al.]. A Study of Continuous Maximum Entropy Deep Inverse Reinforcement Learning. Mathematical Problems in Engineering No. 2019 (2019), pp.1-8.
https://search.emarefa.net/detail/BIM-1195770
American Medical Association (AMA)
Chen, Xi-liang& Cao, Lei& Xu, Zhi-xiong& Lai, Jun& Li, Chen-xi. A Study of Continuous Maximum Entropy Deep Inverse Reinforcement Learning. Mathematical Problems in Engineering. 2019. Vol. 2019, no. 2019, pp.1-8.
https://search.emarefa.net/detail/BIM-1195770
Data Type
Journal Articles
Language
English
Notes
Includes bibliographical references
Record ID
BIM-1195770