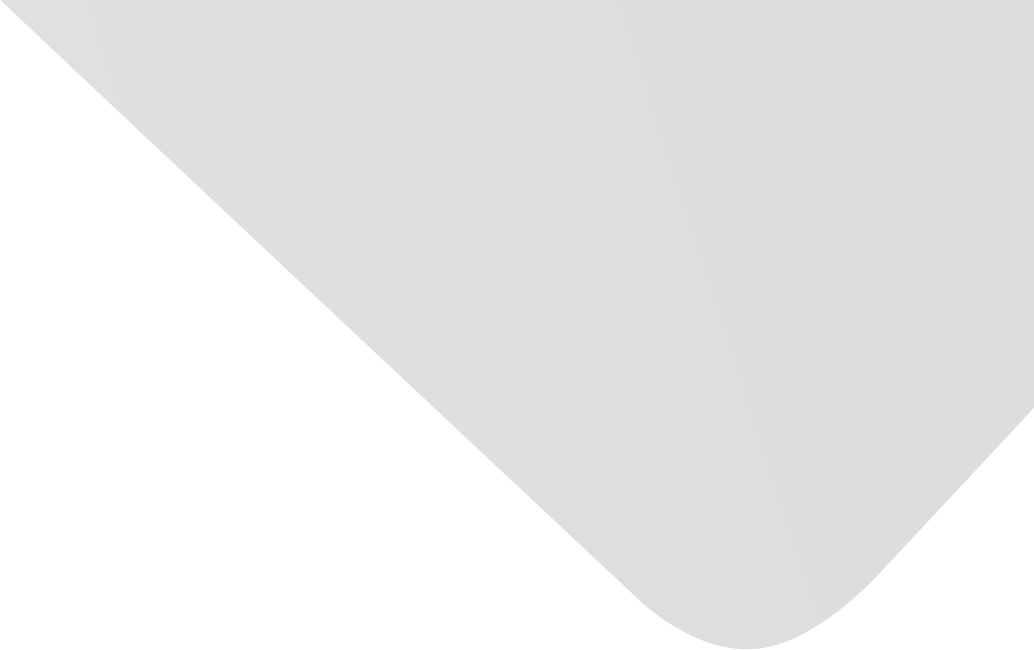
Deep Forest-Based Fault Diagnosis Method for Chemical Process
Joint Authors
You, Jinguo
Jia, Lianyin
Ding, Jiaman
Luo, Qingbo
Source
Mathematical Problems in Engineering
Issue
Vol. 2020, Issue 2020 (31 Dec. 2020), pp.1-15, 15 p.
Publisher
Hindawi Publishing Corporation
Publication Date
2020-01-25
Country of Publication
Egypt
No. of Pages
15
Main Subjects
Abstract EN
With the rapid expanding of big data in all domains, data-driven and deep learning-based fault diagnosis methods in chemical industry have become a major research topic in recent years.
In addition to a deep neural network, deep forest also provides a new idea for deep representation learning and overcomes the shortcomings of a deep neural network such as strong parameter dependence and large training cost.
However, the ability of each base classifier is not taken into account in the standard cascade forest, which may lead to its indistinct discrimination.
In this paper, a multigrained scanning-based weighted cascade forest (WCForest) is proposed and has been applied to fault diagnosis in chemical processes.
In view of the high-dimensional nonlinear data in the process of chemical industry, WCForest first designs a set of relatively suitable windows for the multigrained scan strategy to learn its data representation.
Next, considering the fitting quality of each forest classifier, a weighting strategy is proposed to calculate the weight of each forest in the cascade structure without additional calculation cost, so as to improve the overall performance of the model.
In order to prove the effectiveness of WCForest, its application has been carried out in the benchmark Tennessee Eastman (TE) process.
Experiments demonstrate that WCForest achieves better results than other related approaches across various evaluation metrics.
American Psychological Association (APA)
Ding, Jiaman& Luo, Qingbo& Jia, Lianyin& You, Jinguo. 2020. Deep Forest-Based Fault Diagnosis Method for Chemical Process. Mathematical Problems in Engineering،Vol. 2020, no. 2020, pp.1-15.
https://search.emarefa.net/detail/BIM-1195815
Modern Language Association (MLA)
Ding, Jiaman…[et al.]. Deep Forest-Based Fault Diagnosis Method for Chemical Process. Mathematical Problems in Engineering No. 2020 (2020), pp.1-15.
https://search.emarefa.net/detail/BIM-1195815
American Medical Association (AMA)
Ding, Jiaman& Luo, Qingbo& Jia, Lianyin& You, Jinguo. Deep Forest-Based Fault Diagnosis Method for Chemical Process. Mathematical Problems in Engineering. 2020. Vol. 2020, no. 2020, pp.1-15.
https://search.emarefa.net/detail/BIM-1195815
Data Type
Journal Articles
Language
English
Notes
Includes bibliographical references
Record ID
BIM-1195815