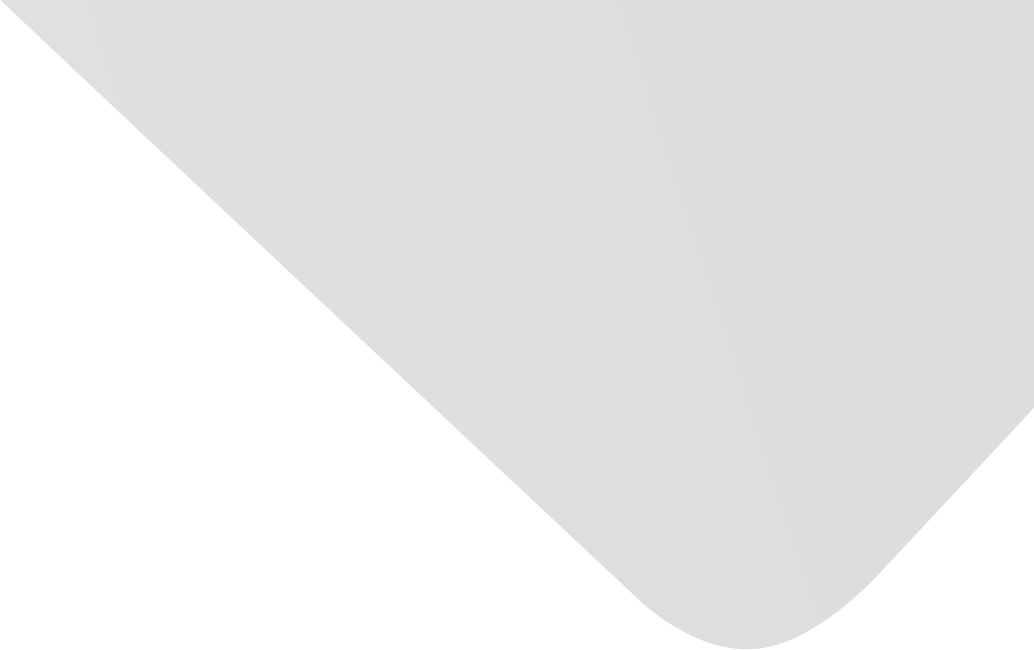
An Integrated Health Condition Detection Method for Rotating Machinery Using Refined Composite Multivariate Multiscale Amplitude-Aware Permutation Entropy
Joint Authors
Zhou, Fuming
Liu, Wuqiang
Feng, Ke
Shen, Jinxing
Gong, Peiping
Source
Mathematical Problems in Engineering
Issue
Vol. 2020, Issue 2020 (31 Dec. 2020), pp.1-23, 23 p.
Publisher
Hindawi Publishing Corporation
Publication Date
2020-12-22
Country of Publication
Egypt
No. of Pages
23
Main Subjects
Abstract EN
With a view to realizing the fault diagnosis of rotating machinery effectively, an integrated health condition detection approach for rotating machinery based on refined composite multivariate multiscale amplitude-aware permutation entropy (RCmvMAAPE), max-relevance and min-redundancy (mRmR), and whale optimization algorithm-based kernel extreme learning machine (WOA-KELM) is presented in this paper.
The approach contains two crucial parts: health detection and fault recognition.
In health detection stage, multivariate amplitude-aware permutation entropy (mvAAPE) is proposed to detect whether there is a fault in rotating machinery.
Afterward, if it is detected that there is a fault, RCmvMAAPE is employed to extract the initial fault features that represent the fault states from the multivariate vibration signals.
Based on the multivariate expansion and multiscale expansion of amplitude-aware permutation entropy, RCmvMAAPE enjoys the ability to effectively extract state information on multiple scales from multichannel series, thereby overcoming the defect of information loss in traditional methods.
Then, mRmR is adopted to screen the sensitive features so as to form sensitive feature vectors, which are input into the WOA-KELM classifier for fault classification.
Two typical rotating machinery cases are conducted to prove the effectiveness of the raised approach.
The experimental results demonstrate that mvAAPE shows excellent performance in fault detection and can effectively detect the fault of rotating machinery.
Meanwhile, the feature extraction method based on RCmvMAAPE and mRmR, as well as the classifier based on WOA-KELM, shows superior performance in feature extraction and fault recognition, respectively.
Compared with other fault identification methods, the raised method enjoys better performance and the average fault recognition accuracy of the two typical cases in this paper can all reach above 98%.
American Psychological Association (APA)
Zhou, Fuming& Liu, Wuqiang& Feng, Ke& Shen, Jinxing& Gong, Peiping. 2020. An Integrated Health Condition Detection Method for Rotating Machinery Using Refined Composite Multivariate Multiscale Amplitude-Aware Permutation Entropy. Mathematical Problems in Engineering،Vol. 2020, no. 2020, pp.1-23.
https://search.emarefa.net/detail/BIM-1195854
Modern Language Association (MLA)
Zhou, Fuming…[et al.]. An Integrated Health Condition Detection Method for Rotating Machinery Using Refined Composite Multivariate Multiscale Amplitude-Aware Permutation Entropy. Mathematical Problems in Engineering No. 2020 (2020), pp.1-23.
https://search.emarefa.net/detail/BIM-1195854
American Medical Association (AMA)
Zhou, Fuming& Liu, Wuqiang& Feng, Ke& Shen, Jinxing& Gong, Peiping. An Integrated Health Condition Detection Method for Rotating Machinery Using Refined Composite Multivariate Multiscale Amplitude-Aware Permutation Entropy. Mathematical Problems in Engineering. 2020. Vol. 2020, no. 2020, pp.1-23.
https://search.emarefa.net/detail/BIM-1195854
Data Type
Journal Articles
Language
English
Notes
Includes bibliographical references
Record ID
BIM-1195854