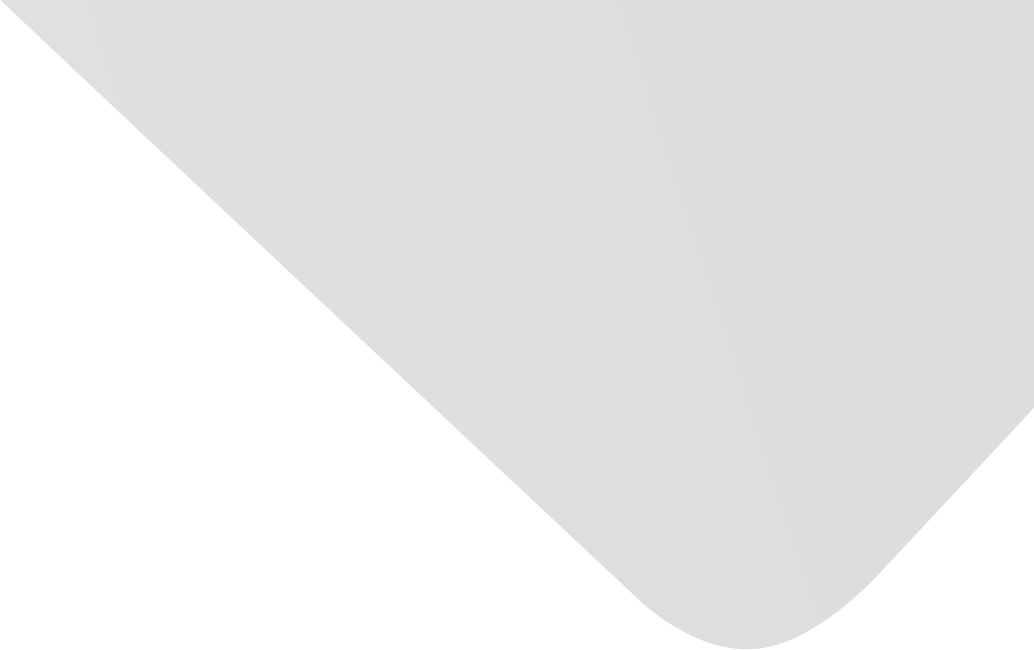
Enhanced Unsupervised Graph Embedding via Hierarchical Graph Convolution Network
Joint Authors
Source
Mathematical Problems in Engineering
Issue
Vol. 2020, Issue 2020 (31 Dec. 2020), pp.1-9, 9 p.
Publisher
Hindawi Publishing Corporation
Publication Date
2020-07-26
Country of Publication
Egypt
No. of Pages
9
Main Subjects
Abstract EN
Graph embedding aims to learn the low-dimensional representation of nodes in the network, which has been paid more and more attention in many graph-based tasks recently.
Graph Convolution Network (GCN) is a typical deep semisupervised graph embedding model, which can acquire node representation from the complex network.
However, GCN usually needs to use a lot of labeled data and additional expressive features in the graph embedding learning process, so the model cannot be effectively applied to undirected graphs with only network structure information.
In this paper, we propose a novel unsupervised graph embedding method via hierarchical graph convolution network (HGCN).
Firstly, HGCN builds the initial node embedding and pseudo-labels for the undirected graphs, and then further uses GCNs to learn the node embedding and update labels, finally combines HGCN output representation with the initial embedding to get the graph embedding.
Furthermore, we improve the model to match the different undirected networks according to the number of network node label types.
Comprehensive experiments demonstrate that our proposed HGCN and HGCN∗ can significantly enhance the performance of the node classification task.
American Psychological Association (APA)
Zhang, H.& Zhou, J. J.& Li, R.. 2020. Enhanced Unsupervised Graph Embedding via Hierarchical Graph Convolution Network. Mathematical Problems in Engineering،Vol. 2020, no. 2020, pp.1-9.
https://search.emarefa.net/detail/BIM-1196149
Modern Language Association (MLA)
Zhang, H.…[et al.]. Enhanced Unsupervised Graph Embedding via Hierarchical Graph Convolution Network. Mathematical Problems in Engineering No. 2020 (2020), pp.1-9.
https://search.emarefa.net/detail/BIM-1196149
American Medical Association (AMA)
Zhang, H.& Zhou, J. J.& Li, R.. Enhanced Unsupervised Graph Embedding via Hierarchical Graph Convolution Network. Mathematical Problems in Engineering. 2020. Vol. 2020, no. 2020, pp.1-9.
https://search.emarefa.net/detail/BIM-1196149
Data Type
Journal Articles
Language
English
Notes
Includes bibliographical references
Record ID
BIM-1196149