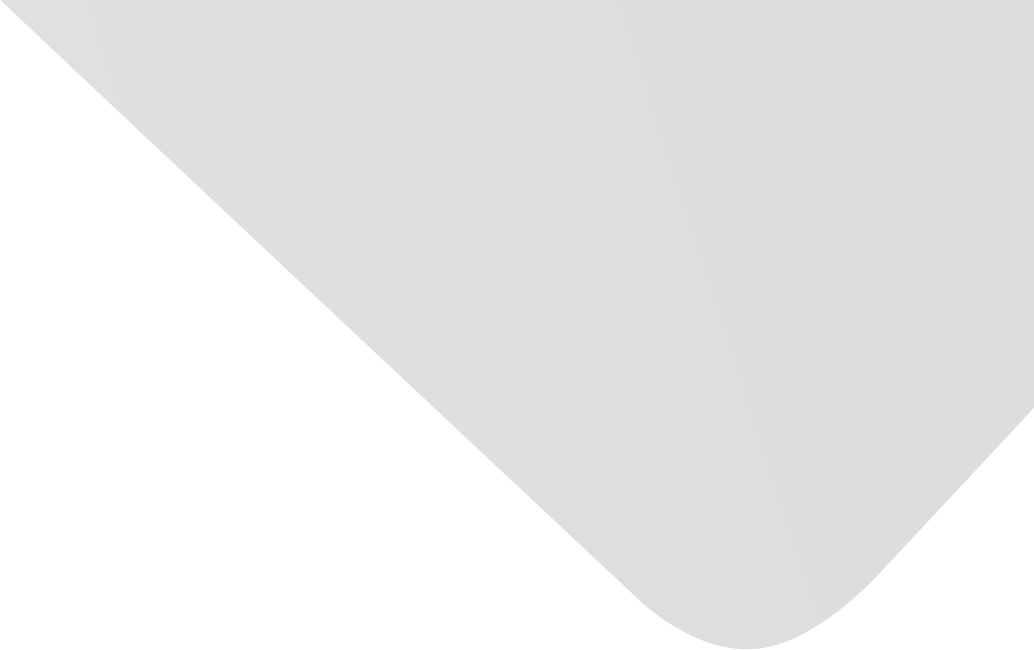
A Road Network Traffic State Identification Method Based on Macroscopic Fundamental Diagram and Spectral Clustering and Support Vector Machine
Author
Source
Mathematical Problems in Engineering
Issue
Vol. 2019, Issue 2019 (31 Dec. 2019), pp.1-10, 10 p.
Publisher
Hindawi Publishing Corporation
Publication Date
2019-04-21
Country of Publication
Egypt
No. of Pages
10
Main Subjects
Abstract EN
Accurate identification of road network traffic status is the key to improve the efficiency of urban traffic control and management.
Both data mining method and MFD-based methods can divide the traffic state of road network, but each has its own advantages and disadvantages.
The data mining method is oriented to traffic data with high efficiency, but it can only discriminate traffic status from microlevel, while the MFD of road network can discriminate traffic status from macrolevel, but there are still some problems, such as the fact that the discriminant method of equivalence points based on MFD lacks theoretical support or that traffic status could not be subdivided.
If data mining methods and road network’s MFD are combined, the accuracy of road network traffic state identification will be greatly improved.
In addition, the research shows that the combination of unsupervised learning clustering analysis method (such as spectral clustering algorithm) and supervised learning machine algorithm (such as support vector machine algorithm (SVM)) is more accurate in traffic state identification.
Therefore, a traffic state identification method based on MFD and spectral clustering and SVM is proposed, combining the advantages of spectral clustering algorithm and SVM algorithm.
Firstly, spectral clustering algorithm is used to classify the traffic state of road network’s MFD.
Secondly, SVM multiclassifier is trained with the partitioned road network’s MFD parameters, and the accuracy evaluation method of classification results based on obfuscation matrix is given.
Finally, the connected-vehicle network simulation platform is built for empirical analysis.
The results show that the classification results of spectral clustering algorithm are closer to the theoretical values, compared with K-means algorithm, and the accuracy of SVM multiclassifier is 96.3%.
It can be seen that our algorithm can identify the road network traffic state more effectively from the macrolevel.
American Psychological Association (APA)
Lin, Xiaohui. 2019. A Road Network Traffic State Identification Method Based on Macroscopic Fundamental Diagram and Spectral Clustering and Support Vector Machine. Mathematical Problems in Engineering،Vol. 2019, no. 2019, pp.1-10.
https://search.emarefa.net/detail/BIM-1196624
Modern Language Association (MLA)
Lin, Xiaohui. A Road Network Traffic State Identification Method Based on Macroscopic Fundamental Diagram and Spectral Clustering and Support Vector Machine. Mathematical Problems in Engineering No. 2019 (2019), pp.1-10.
https://search.emarefa.net/detail/BIM-1196624
American Medical Association (AMA)
Lin, Xiaohui. A Road Network Traffic State Identification Method Based on Macroscopic Fundamental Diagram and Spectral Clustering and Support Vector Machine. Mathematical Problems in Engineering. 2019. Vol. 2019, no. 2019, pp.1-10.
https://search.emarefa.net/detail/BIM-1196624
Data Type
Journal Articles
Language
English
Notes
Includes bibliographical references
Record ID
BIM-1196624