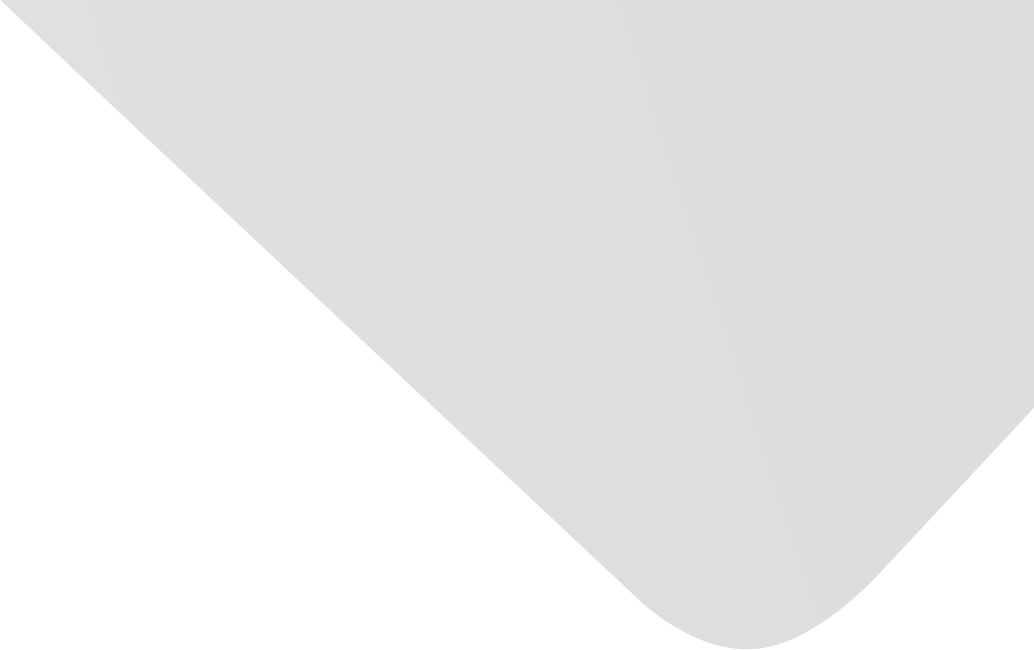
Modern Machine Learning Techniques for Univariate Tunnel Settlement Forecasting: A Comparative Study
Joint Authors
Ji, Zhiwei
Hu, Haigen
Yan, Ke
Hu, Min
Li, Wei
Source
Mathematical Problems in Engineering
Issue
Vol. 2019, Issue 2019 (31 Dec. 2019), pp.1-12, 12 p.
Publisher
Hindawi Publishing Corporation
Publication Date
2019-04-09
Country of Publication
Egypt
No. of Pages
12
Main Subjects
Abstract EN
Tunnel settlement commonly occurs during the tunnel construction processes in large cities.
Existing forecasting methods for tunnel settlements include model-based approaches and artificial intelligence (AI) enhanced approaches.
Compared with traditional forecasting methods, artificial neural networks can be easily implemented, with high performance efficiency and forecasting accuracy.
In this study, an extended machine learning framework is proposed combining particle swarm optimization (PSO) with support vector regression (SVR), back-propagation neural network (BPNN), and extreme learning machine (ELM) to forecast the surface settlement for tunnel construction in two large cities of China P.R.
Based on real-world data verification, the PSO-SVR method shows the highest forecasting accuracy among the three proposed forecasting algorithms.
American Psychological Association (APA)
Hu, Min& Li, Wei& Yan, Ke& Ji, Zhiwei& Hu, Haigen. 2019. Modern Machine Learning Techniques for Univariate Tunnel Settlement Forecasting: A Comparative Study. Mathematical Problems in Engineering،Vol. 2019, no. 2019, pp.1-12.
https://search.emarefa.net/detail/BIM-1196809
Modern Language Association (MLA)
Hu, Min…[et al.]. Modern Machine Learning Techniques for Univariate Tunnel Settlement Forecasting: A Comparative Study. Mathematical Problems in Engineering No. 2019 (2019), pp.1-12.
https://search.emarefa.net/detail/BIM-1196809
American Medical Association (AMA)
Hu, Min& Li, Wei& Yan, Ke& Ji, Zhiwei& Hu, Haigen. Modern Machine Learning Techniques for Univariate Tunnel Settlement Forecasting: A Comparative Study. Mathematical Problems in Engineering. 2019. Vol. 2019, no. 2019, pp.1-12.
https://search.emarefa.net/detail/BIM-1196809
Data Type
Journal Articles
Language
English
Notes
Includes bibliographical references
Record ID
BIM-1196809