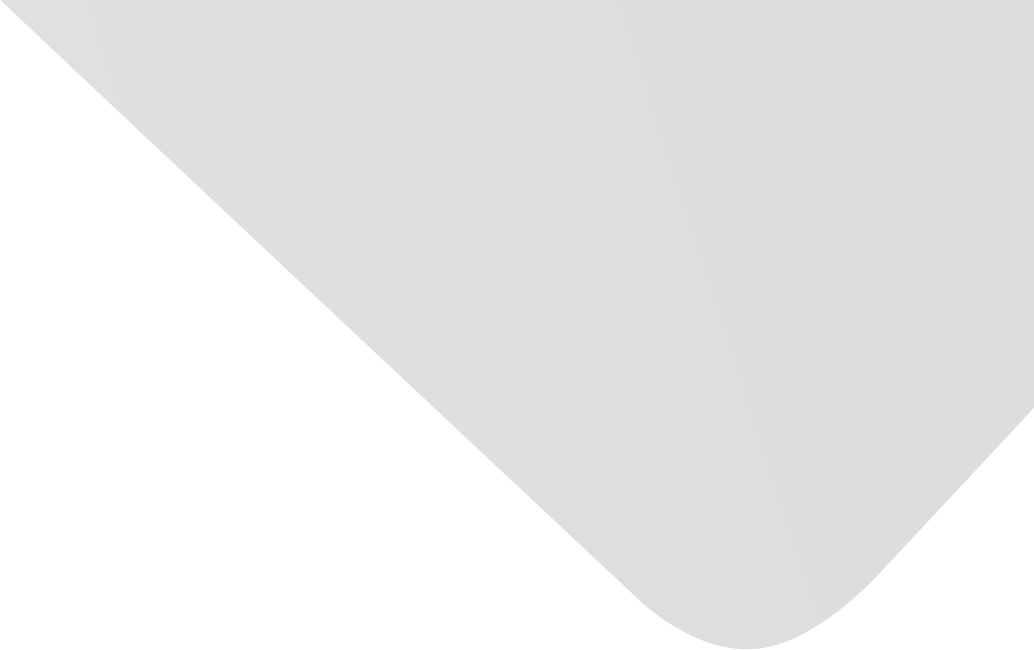
Joint Geosequential Preference and Distance Metric Factorization for Point-of-Interest Recommendation
Joint Authors
Liu, Jiping
Liu, Chunyang
Liu, Chao
Xin, Haiqiang
Xu, Shenghua
Wang, Jian
Source
Mathematical Problems in Engineering
Issue
Vol. 2020, Issue 2020 (31 Dec. 2020), pp.1-14, 14 p.
Publisher
Hindawi Publishing Corporation
Publication Date
2020-10-30
Country of Publication
Egypt
No. of Pages
14
Main Subjects
Abstract EN
Point-of-interest (POI) recommendation is a valuable service to help users discover attractive locations in location-based social networks (LBSNs).
It focuses on capturing users’ movement patterns and location preferences by using massive historical check-in data.
In the past decade, matrix factorization has become a mature and widely used technology in POI recommendation.
However, the inner product of latent vectors adopted in matrix factorization methods does not satisfy the triangle inequality property, which may limit the expressiveness and lead to suboptimal solutions.
Besides, the extreme sparsity of check-in data makes it challenging to capture users’ movement preferences accurately.
In this paper, we propose a joint geosequential preference and distance metric factorization framework, called GeoSeDMF, for POI recommendation.
First, we introduce a distance metric factorization method that is capable of learning users’ personalized preferences from a position and distance perspective in the metric space.
Specifically, we convert the user-POI interaction matrix into a distance matrix and factorize it into user and POI dense embeddings.
Additionally, we measure users’ personalized preference for the POI by using the Euclidean distance metric instead of the inner product.
Then, we model the users’ geospatial preference by applying a geographic weight coefficient and model the users’ sequential preference by using the Euclidean distance of continuous check-in locations.
Moreover, a pointwise loss strategy and AdaGrad algorithm are adopted to optimize the positions and relationships of users and POIs in a metric space.
Finally, experimental results on three large-scale real-world datasets demonstrate the effectiveness and superiority of the proposed method.
American Psychological Association (APA)
Liu, Chunyang& Liu, Chao& Xin, Haiqiang& Wang, Jian& Liu, Jiping& Xu, Shenghua. 2020. Joint Geosequential Preference and Distance Metric Factorization for Point-of-Interest Recommendation. Mathematical Problems in Engineering،Vol. 2020, no. 2020, pp.1-14.
https://search.emarefa.net/detail/BIM-1196888
Modern Language Association (MLA)
Liu, Chunyang…[et al.]. Joint Geosequential Preference and Distance Metric Factorization for Point-of-Interest Recommendation. Mathematical Problems in Engineering No. 2020 (2020), pp.1-14.
https://search.emarefa.net/detail/BIM-1196888
American Medical Association (AMA)
Liu, Chunyang& Liu, Chao& Xin, Haiqiang& Wang, Jian& Liu, Jiping& Xu, Shenghua. Joint Geosequential Preference and Distance Metric Factorization for Point-of-Interest Recommendation. Mathematical Problems in Engineering. 2020. Vol. 2020, no. 2020, pp.1-14.
https://search.emarefa.net/detail/BIM-1196888
Data Type
Journal Articles
Language
English
Notes
Includes bibliographical references
Record ID
BIM-1196888