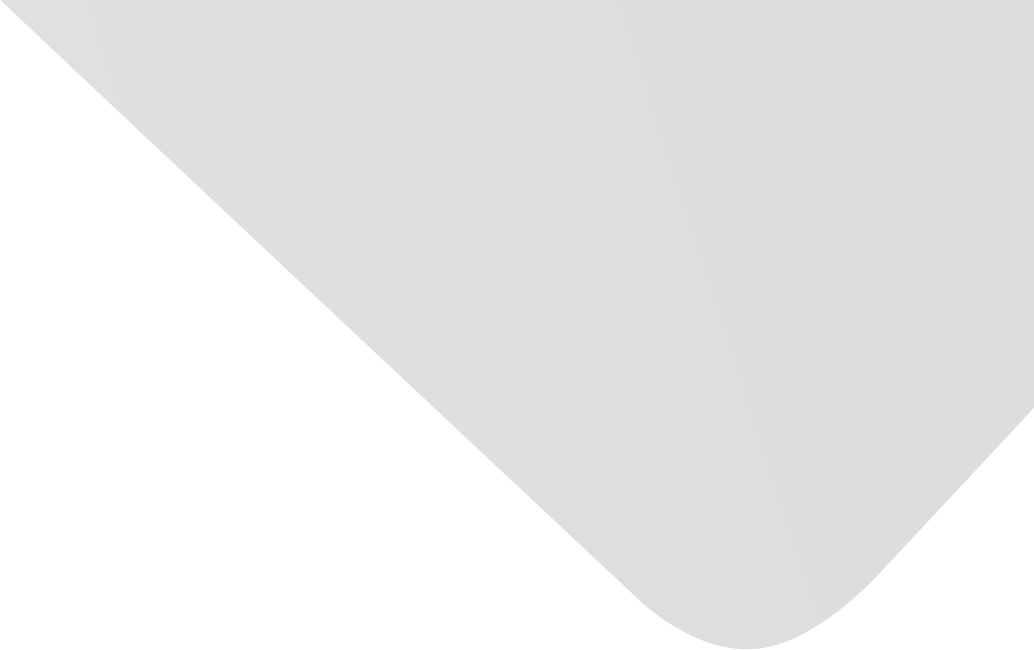
Design and Application of Deep Belief Network Based on Stochastic Adaptive Particle Swarm Optimization
Joint Authors
Yang, Jianjian
Chang, Boshen
Wang, Xiaolin
Zhang, Qiang
Wang, Chao
Wang, Fan
Wu, Miao
Source
Mathematical Problems in Engineering
Issue
Vol. 2020, Issue 2020 (31 Dec. 2020), pp.1-10, 10 p.
Publisher
Hindawi Publishing Corporation
Publication Date
2020-08-24
Country of Publication
Egypt
No. of Pages
10
Main Subjects
Abstract EN
Due to the problem of poor recognition of data with deep fault attribute in the case of traditional superficial network under semisupervised and weak labeling, a deep belief network (DBN) was proposed for deep fault detection.
Due to the problems of deep belief network (DBN) network structure and training parameter selection, a stochastic adaptive particle swarm optimization (RSAPSO) algorithm was proposed in this study to optimize the DBN.
A stochastic criterion was proposed in this method to make the particles jump out of the original position search with a certain probability and reduce the probability of falling into the local optimum.
The RSAPSO-DBN method used sample data to train the DBN and used the final diagnostic error rate to construct the fitness value function of the particle swarm algorithm.
By comparing the minimum fitness value of each particle to determine the advantages and disadvantages of the model, the corresponding minimum fitness value was selected.
Using the number of network nodes, learning rate, and momentum parameters, the optimal DBN classifier was generated for fault diagnosis.
Finally, the validity of the method was verified by bearing data from Case Western Reserve University in the United States and data collected in the laboratory.
Comparing BP (BP neural network), support vector machine, and heterogeneous particle swarm optimization DBN methods, the proposed method demonstrated the highest recognition rates of 87.75% and 93.75%.
This proves that the proposed method possesses universality in fault diagnosis and provides new ideas for data identification with different fault depth attributes.
American Psychological Association (APA)
Yang, Jianjian& Chang, Boshen& Wang, Xiaolin& Zhang, Qiang& Wang, Chao& Wang, Fan…[et al.]. 2020. Design and Application of Deep Belief Network Based on Stochastic Adaptive Particle Swarm Optimization. Mathematical Problems in Engineering،Vol. 2020, no. 2020, pp.1-10.
https://search.emarefa.net/detail/BIM-1196908
Modern Language Association (MLA)
Yang, Jianjian…[et al.]. Design and Application of Deep Belief Network Based on Stochastic Adaptive Particle Swarm Optimization. Mathematical Problems in Engineering No. 2020 (2020), pp.1-10.
https://search.emarefa.net/detail/BIM-1196908
American Medical Association (AMA)
Yang, Jianjian& Chang, Boshen& Wang, Xiaolin& Zhang, Qiang& Wang, Chao& Wang, Fan…[et al.]. Design and Application of Deep Belief Network Based on Stochastic Adaptive Particle Swarm Optimization. Mathematical Problems in Engineering. 2020. Vol. 2020, no. 2020, pp.1-10.
https://search.emarefa.net/detail/BIM-1196908
Data Type
Journal Articles
Language
English
Notes
Includes bibliographical references
Record ID
BIM-1196908