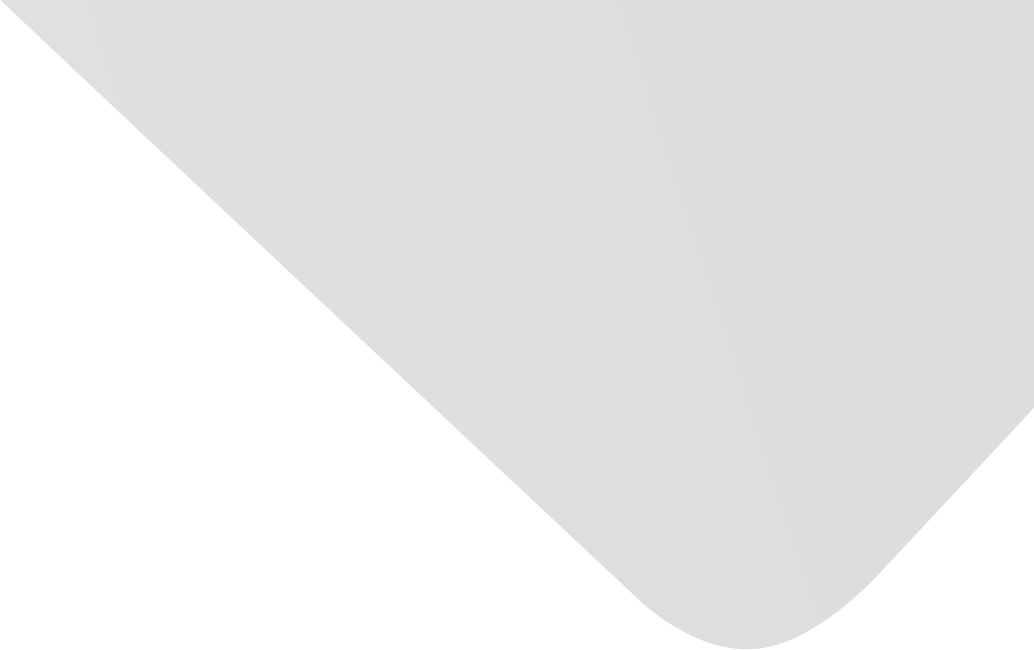
A Fault Diagnosis Method of Rolling Bearing Integrated with Cooperative Energy Feature Extraction and Improved Least-Squares Support Vector Machine
Joint Authors
Xu, Zhang
Huang, Darong
Min, Tang
Ou, Yunhui
Source
Mathematical Problems in Engineering
Issue
Vol. 2020, Issue 2020 (31 Dec. 2020), pp.1-13, 13 p.
Publisher
Hindawi Publishing Corporation
Publication Date
2020-12-24
Country of Publication
Egypt
No. of Pages
13
Main Subjects
Abstract EN
To solve the problem that the bearing fault of variable working conditions is challenging to identify and classify in the industrial field, this paper proposes a new method based on optimization of multidimension fault energy characteristics and integrates with an improved least-squares support vector machine (LSSVM).
First, because the traditional wavelet energy feature is difficult to effectively reflect the characteristics of rolling bearing under different working conditions, based on analyzing the wavelet energy feature extraction in detail, a collaborative method of multidimension fault energy feature extraction combined with the method of Transfer Component Analysis (TCA) is constructed, which improves the discrimination between the different features and the compactness between the same features of rolling bearing faults.
Then, for solving the problem of the local optimal of particle swarm optimization (PSO) in fault diagnosis and recognition of rolling bearing, an improved LSSVM based on particle swarm optimization and wavelet mutation optimization is established to realize the collaborative optimization and adjustment of LSSVM dynamic parameters.
Based on the improved LSSVM and optimization of multidimensional energy characteristics, a new method for fault diagnosis of rolling bearing is designed.
Finally, the simulation and analysis of the proposed algorithm are verified by the experimental data of different working conditions.
The experimental results show that this method can effectively extract the multidimensional fault characteristics under variable working conditions and has a high fault recognition rate.
American Psychological Association (APA)
Xu, Zhang& Huang, Darong& Min, Tang& Ou, Yunhui. 2020. A Fault Diagnosis Method of Rolling Bearing Integrated with Cooperative Energy Feature Extraction and Improved Least-Squares Support Vector Machine. Mathematical Problems in Engineering،Vol. 2020, no. 2020, pp.1-13.
https://search.emarefa.net/detail/BIM-1197037
Modern Language Association (MLA)
Xu, Zhang…[et al.]. A Fault Diagnosis Method of Rolling Bearing Integrated with Cooperative Energy Feature Extraction and Improved Least-Squares Support Vector Machine. Mathematical Problems in Engineering No. 2020 (2020), pp.1-13.
https://search.emarefa.net/detail/BIM-1197037
American Medical Association (AMA)
Xu, Zhang& Huang, Darong& Min, Tang& Ou, Yunhui. A Fault Diagnosis Method of Rolling Bearing Integrated with Cooperative Energy Feature Extraction and Improved Least-Squares Support Vector Machine. Mathematical Problems in Engineering. 2020. Vol. 2020, no. 2020, pp.1-13.
https://search.emarefa.net/detail/BIM-1197037
Data Type
Journal Articles
Language
English
Notes
Includes bibliographical references
Record ID
BIM-1197037