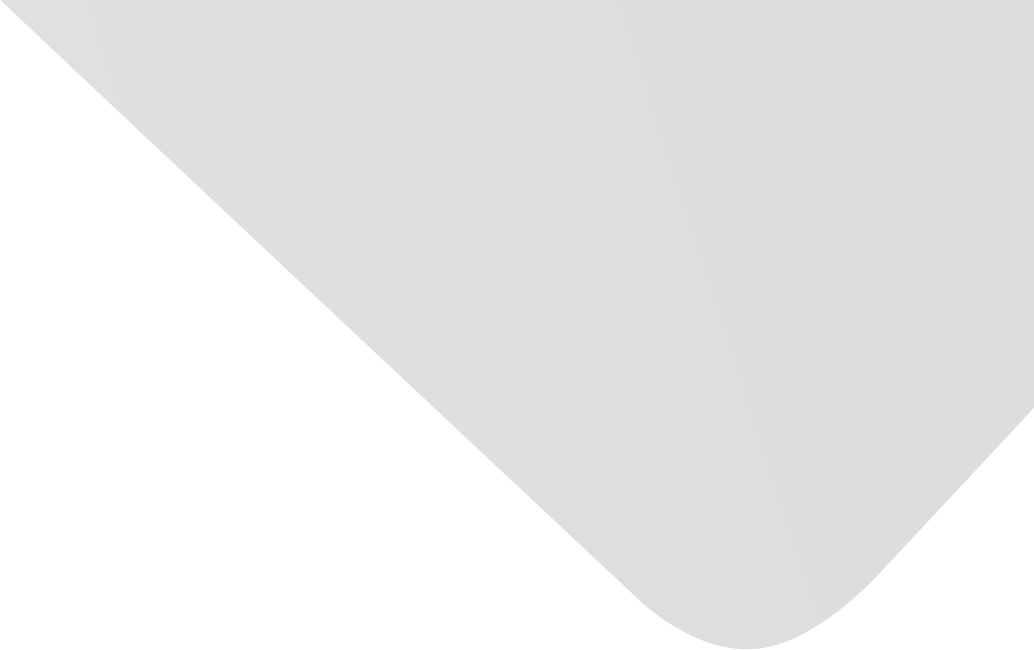
Differential Privacy for Evolving Network Based on GHRG
Joint Authors
Yang, Jing
Wang, Yuye
Zhang, Jianpei
Source
Mathematical Problems in Engineering
Issue
Vol. 2020, Issue 2020 (31 Dec. 2020), pp.1-12, 12 p.
Publisher
Hindawi Publishing Corporation
Publication Date
2020-12-03
Country of Publication
Egypt
No. of Pages
12
Main Subjects
Abstract EN
Releasing evolving networks which contain sensitive information could compromise individual privacy.
In this paper, we study the problem of releasing evolving networks under differential privacy.
We explore the possibility of designing a differentially private evolving networks releasing algorithm.
We found that the majority of traditional methods provide a snapshot of the networks under differential privacy over a brief period of time.
As the network structure only changes in local part, the amount of required noise entirely is large and it leads to an inefficient utility.
To this end, we propose GHRG-DP, a novel differentially private evolving networks releasing algorithm which reduces the noise scale and achieves high data utility.
In the GHRG-DP algorithm, we learn the online connection probabilities between vertices in the evolving networks by generalized hierarchical random graph (GHRG) model.
To fit the dynamic environment, a dendrogram structure adjusting method in local areas is proposed to reduce the noise scale in the whole period of time.
Moreover, to avoid the unhelpful outcome of the connection probabilities, a Bayesian noisy probabilities calculating method is proposed.
Through formal privacy analysis, we show that the GHRG-DP algorithm is ε-differentially private.
Experiments on real evolving network datasets illustrate that GHRG-DP algorithm can privately release evolving networks with high accuracy.
American Psychological Association (APA)
Yang, Jing& Wang, Yuye& Zhang, Jianpei. 2020. Differential Privacy for Evolving Network Based on GHRG. Mathematical Problems in Engineering،Vol. 2020, no. 2020, pp.1-12.
https://search.emarefa.net/detail/BIM-1197293
Modern Language Association (MLA)
Yang, Jing…[et al.]. Differential Privacy for Evolving Network Based on GHRG. Mathematical Problems in Engineering No. 2020 (2020), pp.1-12.
https://search.emarefa.net/detail/BIM-1197293
American Medical Association (AMA)
Yang, Jing& Wang, Yuye& Zhang, Jianpei. Differential Privacy for Evolving Network Based on GHRG. Mathematical Problems in Engineering. 2020. Vol. 2020, no. 2020, pp.1-12.
https://search.emarefa.net/detail/BIM-1197293
Data Type
Journal Articles
Language
English
Notes
Includes bibliographical references
Record ID
BIM-1197293