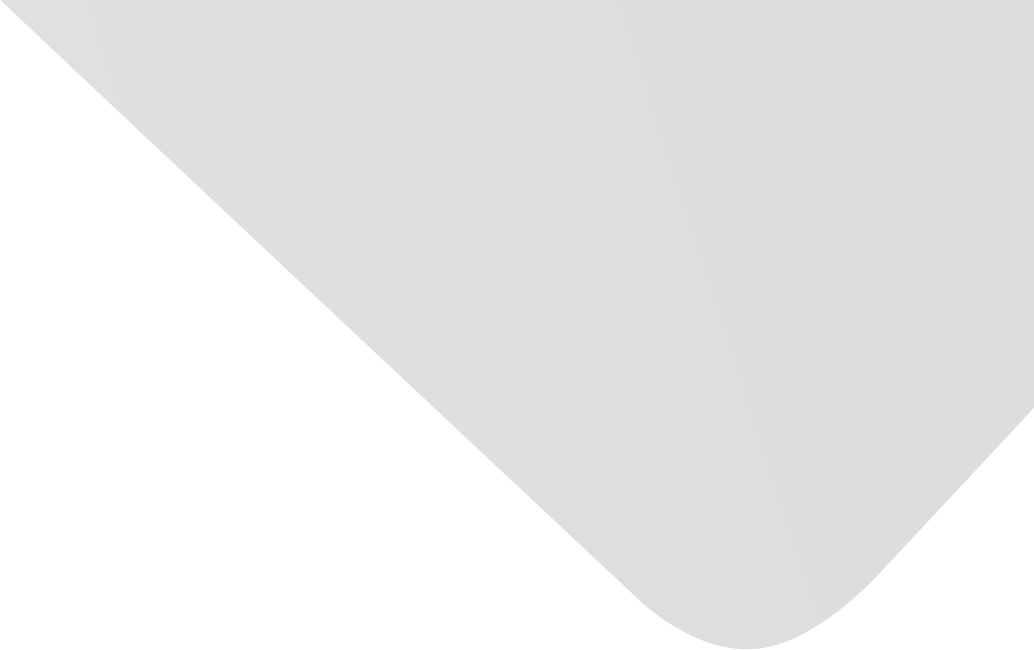
A Novel Entropy-Based Decoding Algorithm for a Generalized High-Order Discrete Hidden Markov Model
Joint Authors
Ong, Hong Choon
Chan, Jason Chin-Tiong
Source
Journal of Probability and Statistics
Issue
Vol. 2018, Issue 2018 (31 Dec. 2018), pp.1-15, 15 p.
Publisher
Hindawi Publishing Corporation
Publication Date
2018-05-02
Country of Publication
Egypt
No. of Pages
15
Main Subjects
Abstract EN
The optimal state sequence of a generalized High-Order Hidden Markov Model (HHMM) is tracked from a given observational sequence using the classical Viterbi algorithm.
This classical algorithm is based on maximum likelihood criterion.
We introduce an entropy-based Viterbi algorithm for tracking the optimal state sequence of a HHMM.
The entropy of a state sequence is a useful quantity, providing a measure of the uncertainty of a HHMM.
There will be no uncertainty if there is only one possible optimal state sequence for HHMM.
This entropy-based decoding algorithm can be formulated in an extended or a reduction approach.
We extend the entropy-based algorithm for computing the optimal state sequence that was developed from a first-order to a generalized HHMM with a single observational sequence.
This extended algorithm performs the computation exponentially with respect to the order of HMM.
The computational complexity of this extended algorithm is due to the growth of the model parameters.
We introduce an efficient entropy-based decoding algorithm that used reduction approach, namely, entropy-based order-transformation forward algorithm (EOTFA) to compute the optimal state sequence of any generalized HHMM.
This EOTFA algorithm involves a transformation of a generalized high-order HMM into an equivalent first-order HMM and an entropy-based decoding algorithm is developed based on the equivalent first-order HMM.
This algorithm performs the computation based on the observational sequence and it requires OTN~2 calculations, where N~ is the number of states in an equivalent first-order model and T is the length of observational sequence.
American Psychological Association (APA)
Chan, Jason Chin-Tiong& Ong, Hong Choon. 2018. A Novel Entropy-Based Decoding Algorithm for a Generalized High-Order Discrete Hidden Markov Model. Journal of Probability and Statistics،Vol. 2018, no. 2018, pp.1-15.
https://search.emarefa.net/detail/BIM-1197701
Modern Language Association (MLA)
Chan, Jason Chin-Tiong& Ong, Hong Choon. A Novel Entropy-Based Decoding Algorithm for a Generalized High-Order Discrete Hidden Markov Model. Journal of Probability and Statistics No. 2018 (2018), pp.1-15.
https://search.emarefa.net/detail/BIM-1197701
American Medical Association (AMA)
Chan, Jason Chin-Tiong& Ong, Hong Choon. A Novel Entropy-Based Decoding Algorithm for a Generalized High-Order Discrete Hidden Markov Model. Journal of Probability and Statistics. 2018. Vol. 2018, no. 2018, pp.1-15.
https://search.emarefa.net/detail/BIM-1197701
Data Type
Journal Articles
Language
English
Notes
Includes bibliographical references
Record ID
BIM-1197701