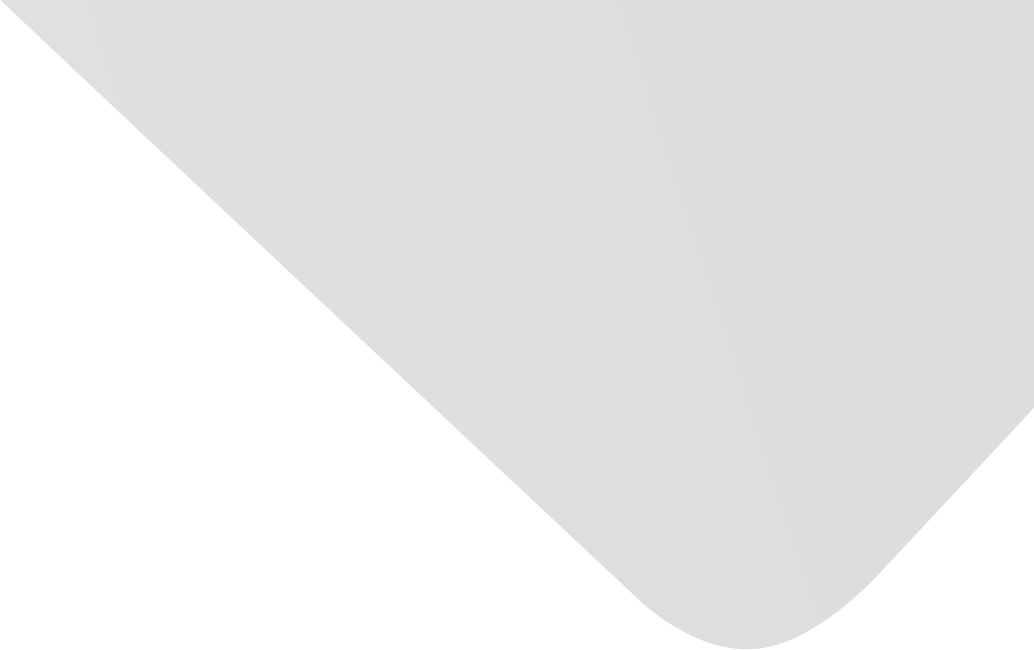
Elimination of End effects in LMD Based on LSTM Network and Applications for Rolling Bearing Fault Feature Extraction
Joint Authors
Wu, J.
Liang, Jianhong
Liu, Zhigui
Wang, Li-Ping
Source
Mathematical Problems in Engineering
Issue
Vol. 2020, Issue 2020 (31 Dec. 2020), pp.1-16, 16 p.
Publisher
Hindawi Publishing Corporation
Publication Date
2020-01-09
Country of Publication
Egypt
No. of Pages
16
Main Subjects
Abstract EN
Local mean decomposition (LMD) is widely used in the area of multicomponents signal processing and fault diagnosis.
One of the major problems is end effects, which distort the decomposed waveform at each end of the analyzed signal and influence feature frequency.
In order to solve this problem, this paper proposes a novel self-adaptive waveform point extended method based on long short-term memory (LSTM) network.
First, based on existing signal points, the LSTM network parameters of right and left ends are trained; then, these parameters are used to extend the waveform point at each end-side of signal; furthermore, the corresponding parameters are adaptively updated.
The proposed method is compared with the characteristic segment extension and the traditional neural network extension methods through a simulated signal to verify the effectiveness.
By combing the proposed method with LMD, an improved LMD algorithm is obtained.
Finally, application of rolling bearing fault signal is carried out by the improved LMD algorithm, and the results show that the feature frequencies of the rolling bearing’s ball and inner and outer rings are successfully extracted.
American Psychological Association (APA)
Liang, Jianhong& Wang, Li-Ping& Wu, J.& Liu, Zhigui. 2020. Elimination of End effects in LMD Based on LSTM Network and Applications for Rolling Bearing Fault Feature Extraction. Mathematical Problems in Engineering،Vol. 2020, no. 2020, pp.1-16.
https://search.emarefa.net/detail/BIM-1197860
Modern Language Association (MLA)
Liang, Jianhong…[et al.]. Elimination of End effects in LMD Based on LSTM Network and Applications for Rolling Bearing Fault Feature Extraction. Mathematical Problems in Engineering No. 2020 (2020), pp.1-16.
https://search.emarefa.net/detail/BIM-1197860
American Medical Association (AMA)
Liang, Jianhong& Wang, Li-Ping& Wu, J.& Liu, Zhigui. Elimination of End effects in LMD Based on LSTM Network and Applications for Rolling Bearing Fault Feature Extraction. Mathematical Problems in Engineering. 2020. Vol. 2020, no. 2020, pp.1-16.
https://search.emarefa.net/detail/BIM-1197860
Data Type
Journal Articles
Language
English
Notes
Includes bibliographical references
Record ID
BIM-1197860