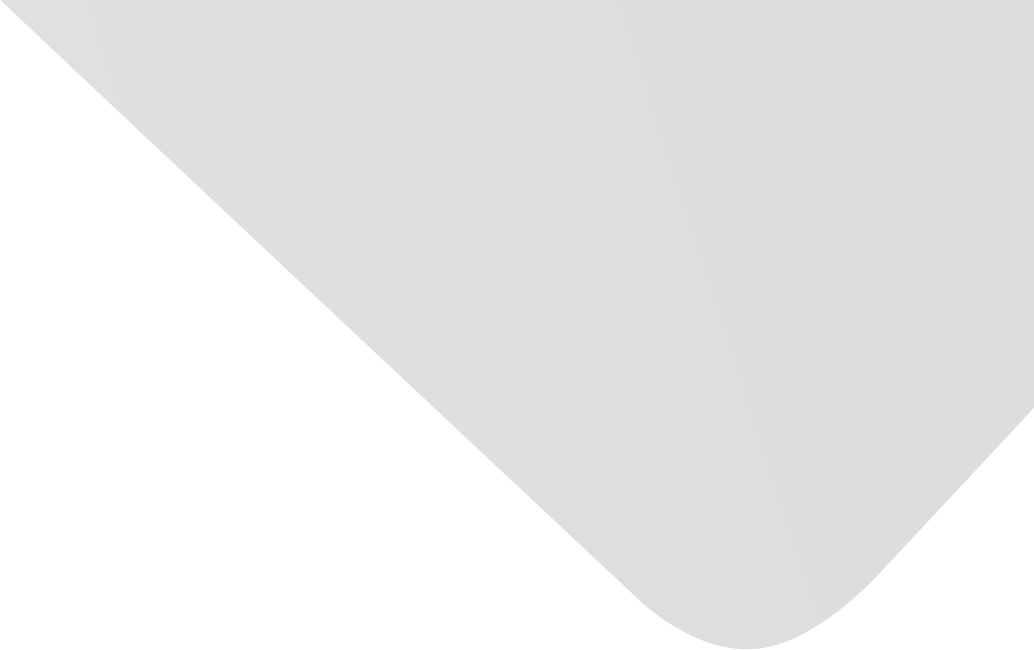
Forest Canopy Height Estimation Using Multiplatform Remote Sensing Dataset
Joint Authors
Source
Issue
Vol. 2018, Issue 2018 (31 Dec. 2018), pp.1-9, 9 p.
Publisher
Hindawi Publishing Corporation
Publication Date
2018-04-11
Country of Publication
Egypt
No. of Pages
9
Main Subjects
Abstract EN
Recently, numerous studies have attempted to determine forest height using remote sensing techniques that not only have the benefits of fast data acquisition, processing, and analysis but are also cost-effective.
However, if there was insufficient data to apply the latest remote sensing techniques, we need to consider many kinds of datasets as possible.
In this study, we tried to determine forest height using discrete-return LiDAR data, SRTM, satellite L-band SAR data, and Optical data.
We experimented with the differences between LiDAR DSM and DTM, as well as SRTM DSM and LiDAR DTM.
In addition, we applied an SBAS algorithm and linear regression to the dataset.
From the quantitative evaluation, the RMSE and R2 of the LiDAR-derived forest height (3.22 m and 0.43, resp.) and the SRTM-derived forest height (2.90 m and 0.50, resp.) were both reasonably good, especially when we consider data acquisition time differences and measurement errors in mountainous areas.
Moreover, we slightly improved the RMSE and R2 from 2.90 m and 0.50, respectively, to 2.75 m and 0.54, respectively, by correcting the SRTM using the SBAS algorithm.
Furthermore, we merged the datasets using linear regression and obtained improved forest heights with RMSE and R2 values of 2.68 m and 0.56, respectively.
To generate a forest height map, we used NDVI from Optical imagery and masked heights below 2 m from each sensor.
Thus, we excluded urban areas, “bare earth surfaces,” and mountain streams from each sensor’s imagery.
Finally, we generated a forest height map by overlapping the datasets.
The results of this study indicate that each sensor has the potential for not only determining forest height but also extracting complementary forest area information.
Furthermore, this study demonstrates the potential for improvement using the SBAS algorithm and linear regression.
American Psychological Association (APA)
Lee, Won-Jin& Lee, Chang-Wook. 2018. Forest Canopy Height Estimation Using Multiplatform Remote Sensing Dataset. Journal of Sensors،Vol. 2018, no. 2018, pp.1-9.
https://search.emarefa.net/detail/BIM-1200750
Modern Language Association (MLA)
Lee, Won-Jin& Lee, Chang-Wook. Forest Canopy Height Estimation Using Multiplatform Remote Sensing Dataset. Journal of Sensors No. 2018 (2018), pp.1-9.
https://search.emarefa.net/detail/BIM-1200750
American Medical Association (AMA)
Lee, Won-Jin& Lee, Chang-Wook. Forest Canopy Height Estimation Using Multiplatform Remote Sensing Dataset. Journal of Sensors. 2018. Vol. 2018, no. 2018, pp.1-9.
https://search.emarefa.net/detail/BIM-1200750
Data Type
Journal Articles
Language
English
Notes
Includes bibliographical references
Record ID
BIM-1200750