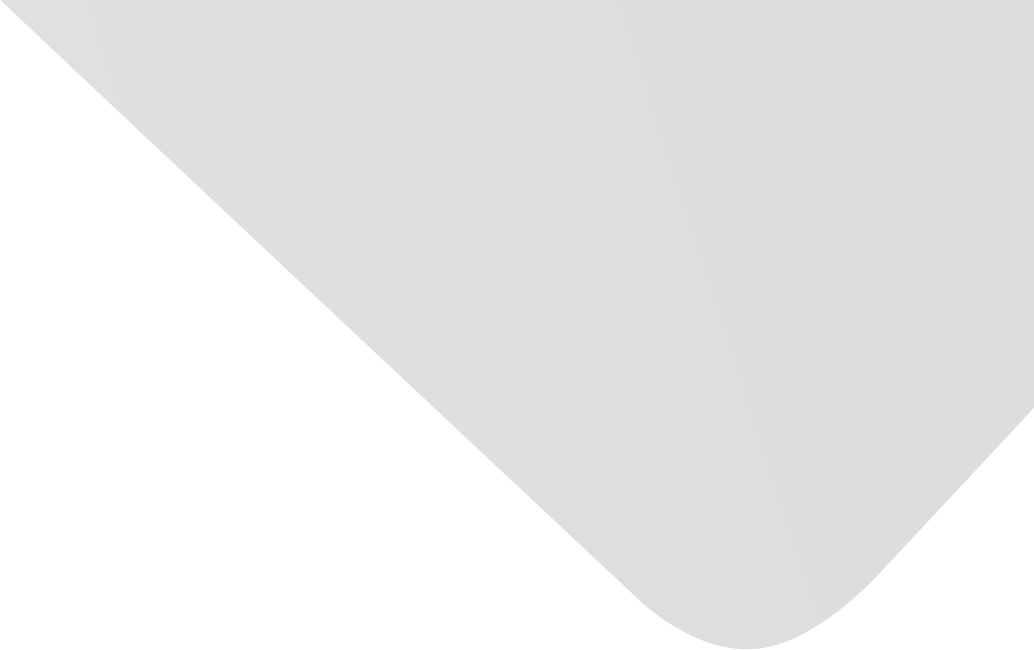
Object Detection with the Addition of New Classes Based on the Method of RNOL
Joint Authors
Source
Mathematical Problems in Engineering
Issue
Vol. 2020, Issue 2020 (31 Dec. 2020), pp.1-6, 6 p.
Publisher
Hindawi Publishing Corporation
Publication Date
2020-05-12
Country of Publication
Egypt
No. of Pages
6
Main Subjects
Abstract EN
Object detection plays an important role in many computer vision applications.
Innovative object detection methods based on deep learning such as Faster R-CNN, YOLO, and SSD have achieved state-of the-art results in terms of detection accuracy.
There have been few studies to date on object detection with the addition of new classes, however, though this problem is often encountered in the industry.
Therefore, this issue has important research significance and practical value.
On the premise that the old class samples are available, a method of reserving nodes in advance in the output layer (RNOL) was established in this study.
Experiments show that RNOL can achieve high detection accuracy in both new and old classes over a short training time while outperforming the traditional fine-tuning method.
American Psychological Association (APA)
Fang, Haiquan& Zhu, Feijia. 2020. Object Detection with the Addition of New Classes Based on the Method of RNOL. Mathematical Problems in Engineering،Vol. 2020, no. 2020, pp.1-6.
https://search.emarefa.net/detail/BIM-1202062
Modern Language Association (MLA)
Fang, Haiquan& Zhu, Feijia. Object Detection with the Addition of New Classes Based on the Method of RNOL. Mathematical Problems in Engineering No. 2020 (2020), pp.1-6.
https://search.emarefa.net/detail/BIM-1202062
American Medical Association (AMA)
Fang, Haiquan& Zhu, Feijia. Object Detection with the Addition of New Classes Based on the Method of RNOL. Mathematical Problems in Engineering. 2020. Vol. 2020, no. 2020, pp.1-6.
https://search.emarefa.net/detail/BIM-1202062
Data Type
Journal Articles
Language
English
Notes
Includes bibliographical references
Record ID
BIM-1202062