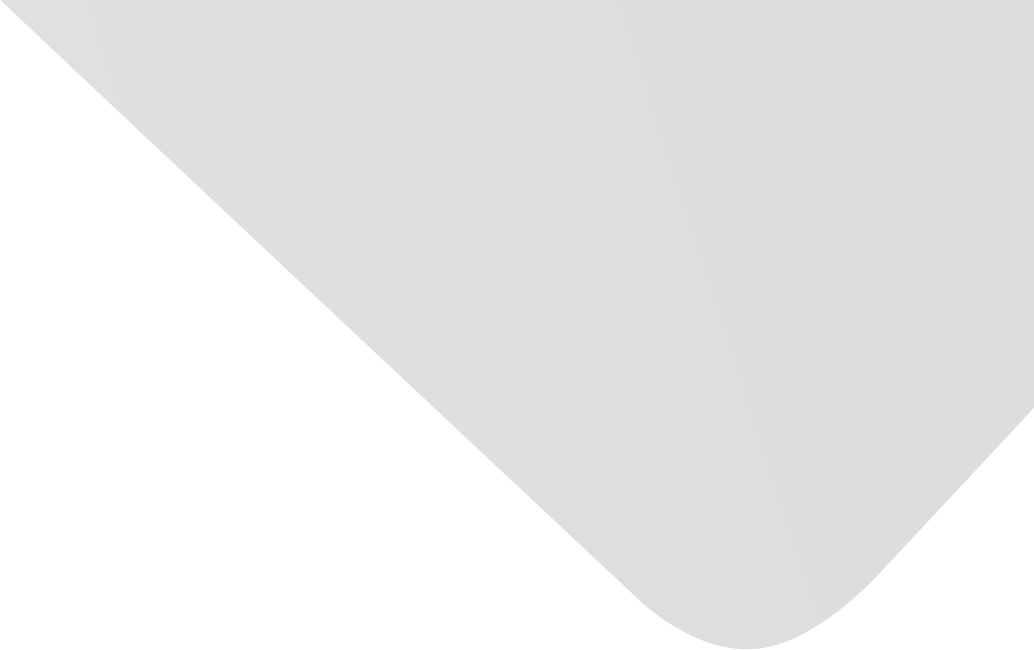
Deep Learning Based Abstractive Text Summarization: Approaches, Datasets, Evaluation Measures, and Challenges
Joint Authors
Source
Mathematical Problems in Engineering
Issue
Vol. 2020, Issue 2020 (31 Dec. 2020), pp.1-29, 29 p.
Publisher
Hindawi Publishing Corporation
Publication Date
2020-08-24
Country of Publication
Egypt
No. of Pages
29
Main Subjects
Abstract EN
In recent years, the volume of textual data has rapidly increased, which has generated a valuable resource for extracting and analysing information.
To retrieve useful knowledge within a reasonable time period, this information must be summarised.
This paper reviews recent approaches for abstractive text summarisation using deep learning models.
In addition, existing datasets for training and validating these approaches are reviewed, and their features and limitations are presented.
The Gigaword dataset is commonly employed for single-sentence summary approaches, while the Cable News Network (CNN)/Daily Mail dataset is commonly employed for multisentence summary approaches.
Furthermore, the measures that are utilised to evaluate the quality of summarisation are investigated, and Recall-Oriented Understudy for Gisting Evaluation 1 (ROUGE1), ROUGE2, and ROUGE-L are determined to be the most commonly applied metrics.
The challenges that are encountered during the summarisation process and the solutions proposed in each approach are analysed.
The analysis of the several approaches shows that recurrent neural networks with an attention mechanism and long short-term memory (LSTM) are the most prevalent techniques for abstractive text summarisation.
The experimental results show that text summarisation with a pretrained encoder model achieved the highest values for ROUGE1, ROUGE2, and ROUGE-L (43.85, 20.34, and 39.9, respectively).
Furthermore, it was determined that most abstractive text summarisation models faced challenges such as the unavailability of a golden token at testing time, out-of-vocabulary (OOV) words, summary sentence repetition, inaccurate sentences, and fake facts.
American Psychological Association (APA)
Suleiman, Dima& Awajan, Arafat. 2020. Deep Learning Based Abstractive Text Summarization: Approaches, Datasets, Evaluation Measures, and Challenges. Mathematical Problems in Engineering،Vol. 2020, no. 2020, pp.1-29.
https://search.emarefa.net/detail/BIM-1202185
Modern Language Association (MLA)
Suleiman, Dima& Awajan, Arafat. Deep Learning Based Abstractive Text Summarization: Approaches, Datasets, Evaluation Measures, and Challenges. Mathematical Problems in Engineering No. 2020 (2020), pp.1-29.
https://search.emarefa.net/detail/BIM-1202185
American Medical Association (AMA)
Suleiman, Dima& Awajan, Arafat. Deep Learning Based Abstractive Text Summarization: Approaches, Datasets, Evaluation Measures, and Challenges. Mathematical Problems in Engineering. 2020. Vol. 2020, no. 2020, pp.1-29.
https://search.emarefa.net/detail/BIM-1202185
Data Type
Journal Articles
Language
English
Notes
Includes bibliographical references
Record ID
BIM-1202185