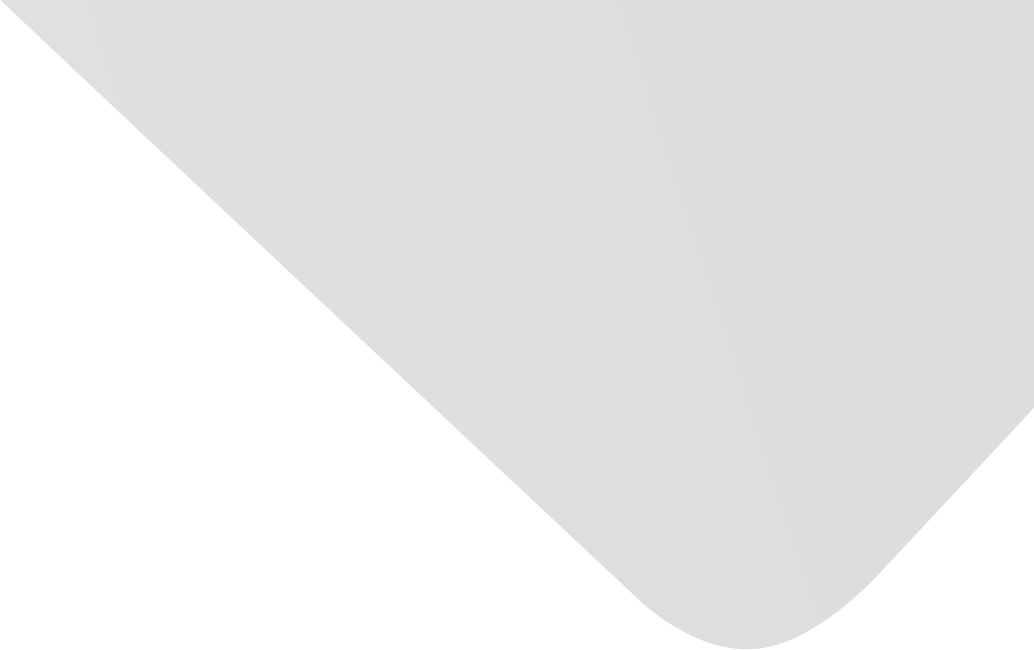
Dimensionality Reduction with Sparse Locality for Principal Component Analysis
Joint Authors
Youn, Hee Yong
Li, Pei Heng
Lee, Taeho
Source
Mathematical Problems in Engineering
Issue
Vol. 2020, Issue 2020 (31 Dec. 2020), pp.1-12, 12 p.
Publisher
Hindawi Publishing Corporation
Publication Date
2020-05-20
Country of Publication
Egypt
No. of Pages
12
Main Subjects
Abstract EN
Various dimensionality reduction (DR) schemes have been developed for projecting high-dimensional data into low-dimensional representation.
The existing schemes usually preserve either only the global structure or local structure of the original data, but not both.
To resolve this issue, a scheme called sparse locality for principal component analysis (SLPCA) is proposed.
In order to effectively consider the trade-off between the complexity and efficiency, a robust L2,p-norm-based principal component analysis (R2P-PCA) is introduced for global DR, while sparse representation-based locality preserving projection (SR-LPP) is used for local DR.
Sparse representation is also employed to construct the weighted matrix of the samples.
Being parameter-free, this allows the construction of an intrinsic graph more robust against the noise.
In addition, simultaneous learning of projection matrix and sparse similarity matrix is possible.
Experimental results demonstrate that the proposed scheme consistently outperforms the existing schemes in terms of clustering accuracy and data reconstruction error.
American Psychological Association (APA)
Li, Pei Heng& Lee, Taeho& Youn, Hee Yong. 2020. Dimensionality Reduction with Sparse Locality for Principal Component Analysis. Mathematical Problems in Engineering،Vol. 2020, no. 2020, pp.1-12.
https://search.emarefa.net/detail/BIM-1202425
Modern Language Association (MLA)
Li, Pei Heng…[et al.]. Dimensionality Reduction with Sparse Locality for Principal Component Analysis. Mathematical Problems in Engineering No. 2020 (2020), pp.1-12.
https://search.emarefa.net/detail/BIM-1202425
American Medical Association (AMA)
Li, Pei Heng& Lee, Taeho& Youn, Hee Yong. Dimensionality Reduction with Sparse Locality for Principal Component Analysis. Mathematical Problems in Engineering. 2020. Vol. 2020, no. 2020, pp.1-12.
https://search.emarefa.net/detail/BIM-1202425
Data Type
Journal Articles
Language
English
Notes
Includes bibliographical references
Record ID
BIM-1202425