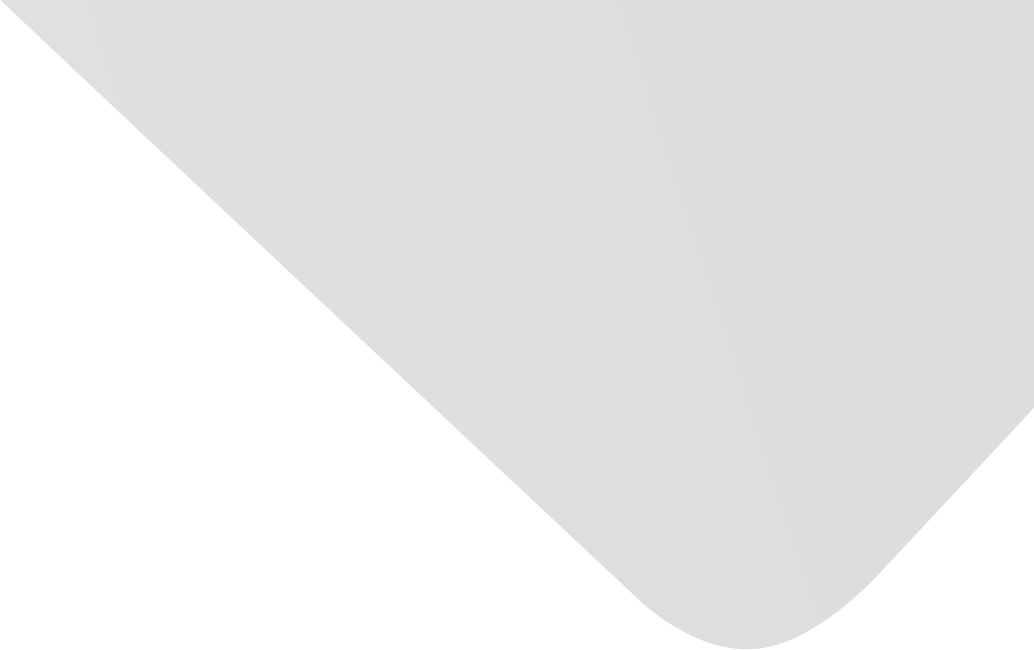
Nonlinear Regression with High-Dimensional Space Mapping for Blood Component Spectral Quantitative Analysis
Joint Authors
Zhou, Yan
Cao, Hui
Zhang, Yanbin
Zhang, Shiliang
Ma, Xiaoyan
Source
Issue
Vol. 2018, Issue 2018 (31 Dec. 2018), pp.1-8, 8 p.
Publisher
Hindawi Publishing Corporation
Publication Date
2018-01-10
Country of Publication
Egypt
No. of Pages
8
Main Subjects
Abstract EN
Accurate and fast determination of blood component concentration is very essential for the efficient diagnosis of patients.
This paper proposes a nonlinear regression method with high-dimensional space mapping for blood component spectral quantitative analysis.
Kernels are introduced to map the input data into high-dimensional space for nonlinear regression.
As the most famous kernel, Gaussian kernel is usually adopted by researchers.
More kernels need to be studied for each kernel describes its own high-dimensional feature space mapping which affects regression performance.
In this paper, eight kernels are used to discuss the influence of different space mapping to the blood component spectral quantitative analysis.
Each kernel and corresponding parameters are assessed to build the optimal regression model.
The proposed method is conducted on a real blood spectral data obtained from the uric acid determination.
Results verify that the prediction errors of proposed models are more precise than the ones obtained by linear models.
Support vector regression (SVR) provides better performance than partial least square (PLS) when combined with kernels.
The local kernels are recommended according to the blood spectral data features.
SVR with inverse multiquadric kernel has the best predictive performance that can be used for blood component spectral quantitative analysis.
American Psychological Association (APA)
Ma, Xiaoyan& Zhang, Yanbin& Cao, Hui& Zhang, Shiliang& Zhou, Yan. 2018. Nonlinear Regression with High-Dimensional Space Mapping for Blood Component Spectral Quantitative Analysis. Journal of Spectroscopy،Vol. 2018, no. 2018, pp.1-8.
https://search.emarefa.net/detail/BIM-1202449
Modern Language Association (MLA)
Ma, Xiaoyan…[et al.]. Nonlinear Regression with High-Dimensional Space Mapping for Blood Component Spectral Quantitative Analysis. Journal of Spectroscopy No. 2018 (2018), pp.1-8.
https://search.emarefa.net/detail/BIM-1202449
American Medical Association (AMA)
Ma, Xiaoyan& Zhang, Yanbin& Cao, Hui& Zhang, Shiliang& Zhou, Yan. Nonlinear Regression with High-Dimensional Space Mapping for Blood Component Spectral Quantitative Analysis. Journal of Spectroscopy. 2018. Vol. 2018, no. 2018, pp.1-8.
https://search.emarefa.net/detail/BIM-1202449
Data Type
Journal Articles
Language
English
Notes
Includes bibliographical references
Record ID
BIM-1202449