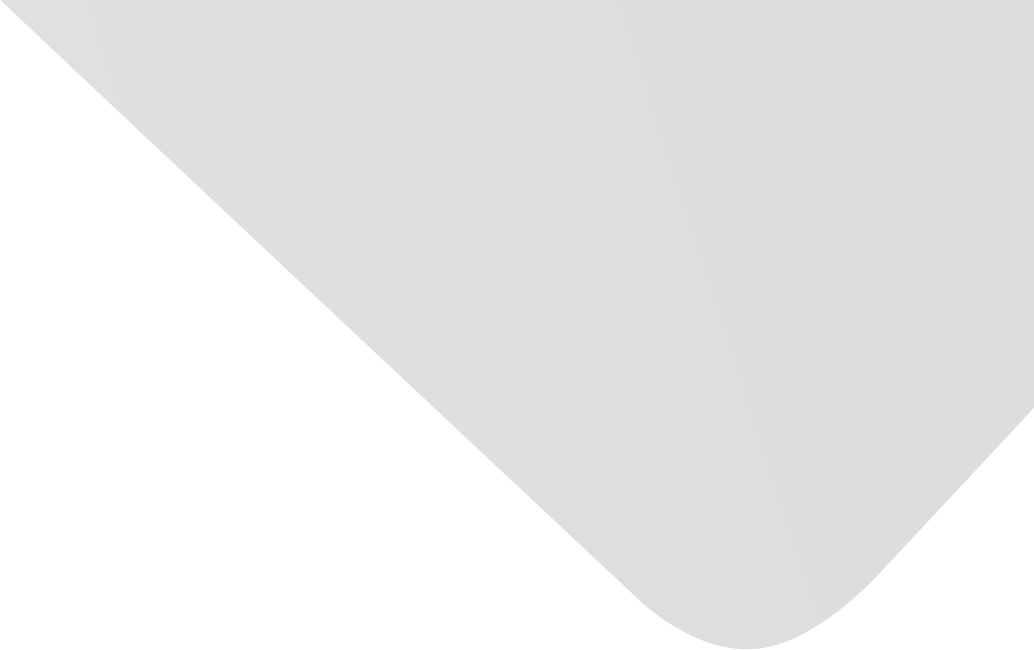
Apple Variety Identification Using Near-Infrared Spectroscopy
Joint Authors
Li, Caihong
Li, Lingling
Wu, Yuan
Lu, Min
Li, Lian
Yang, Yi
Source
Issue
Vol. 2018, Issue 2018 (31 Dec. 2018), pp.1-7, 7 p.
Publisher
Hindawi Publishing Corporation
Publication Date
2018-08-27
Country of Publication
Egypt
No. of Pages
7
Main Subjects
Abstract EN
Near-infrared (NIR) spectra of apple samples were submitted in this paper to principal component analysis (PCA) and successive projections algorithm (SPA) to conduct variable selection.
Three pattern recognition methods, backpropagation neural network (BPNN), support vector machine (SVM), and extreme learning machine (ELM), were applied to establish models for distinguishing apples of different varieties and geographical origins.
Experimental results show that ELM models performed better on identifying apple variety and geographical origin than others.
Especially, the SPA-ELM model could reach 98.33% identification accuracy on the calibration set and 96.67% on the prediction set.
This study suggests that it is feasible to identify apple variety and cultivation region by using NIR spectroscopy.
American Psychological Association (APA)
Li, Caihong& Li, Lingling& Wu, Yuan& Lu, Min& Yang, Yi& Li, Lian. 2018. Apple Variety Identification Using Near-Infrared Spectroscopy. Journal of Spectroscopy،Vol. 2018, no. 2018, pp.1-7.
https://search.emarefa.net/detail/BIM-1202589
Modern Language Association (MLA)
Li, Caihong…[et al.]. Apple Variety Identification Using Near-Infrared Spectroscopy. Journal of Spectroscopy No. 2018 (2018), pp.1-7.
https://search.emarefa.net/detail/BIM-1202589
American Medical Association (AMA)
Li, Caihong& Li, Lingling& Wu, Yuan& Lu, Min& Yang, Yi& Li, Lian. Apple Variety Identification Using Near-Infrared Spectroscopy. Journal of Spectroscopy. 2018. Vol. 2018, no. 2018, pp.1-7.
https://search.emarefa.net/detail/BIM-1202589
Data Type
Journal Articles
Language
English
Notes
Includes bibliographical references
Record ID
BIM-1202589