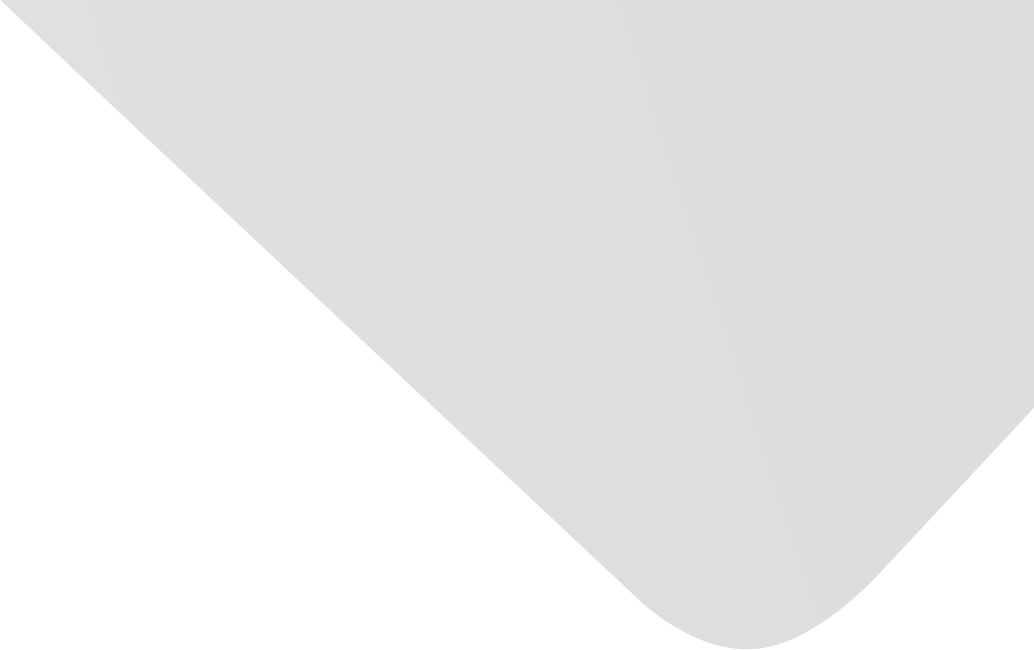
A Machine Learning and Cross-Validation Approach for the Discrimination of Vegetation Physiognomic Types Using Satellite Based Multispectral and Multitemporal Data
Joint Authors
Sharma, Ram C.
Hara, Keitarou
Hirayama, Hidetake
Source
Issue
Vol. 2017, Issue 2017 (31 Dec. 2017), pp.1-8, 8 p.
Publisher
Hindawi Publishing Corporation
Publication Date
2017-06-11
Country of Publication
Egypt
No. of Pages
8
Main Subjects
Abstract EN
This paper presents the performance and evaluation of a number of machine learning classifiers for the discrimination between the vegetation physiognomic classes using the satellite based time-series of the surface reflectance data.
Discrimination of six vegetation physiognomic classes, Evergreen Coniferous Forest, Evergreen Broadleaf Forest, Deciduous Coniferous Forest, Deciduous Broadleaf Forest, Shrubs, and Herbs, was dealt with in the research.
Rich-feature data were prepared from time-series of the satellite data for the discrimination and cross-validation of the vegetation physiognomic types using machine learning approach.
A set of machine learning experiments comprised of a number of supervised classifiers with different model parameters was conducted to assess how the discrimination of vegetation physiognomic classes varies with classifiers, input features, and ground truth data size.
The performance of each experiment was evaluated by using the 10-fold cross-validation method.
Experiment using the Random Forests classifier provided highest overall accuracy (0.81) and kappa coefficient (0.78).
However, accuracy metrics did not vary much with experiments.
Accuracy metrics were found to be very sensitive to input features and size of ground truth data.
The results obtained in the research are expected to be useful for improving the vegetation physiognomic mapping in Japan.
American Psychological Association (APA)
Sharma, Ram C.& Hara, Keitarou& Hirayama, Hidetake. 2017. A Machine Learning and Cross-Validation Approach for the Discrimination of Vegetation Physiognomic Types Using Satellite Based Multispectral and Multitemporal Data. Scientifica،Vol. 2017, no. 2017, pp.1-8.
https://search.emarefa.net/detail/BIM-1202690
Modern Language Association (MLA)
Sharma, Ram C.…[et al.]. A Machine Learning and Cross-Validation Approach for the Discrimination of Vegetation Physiognomic Types Using Satellite Based Multispectral and Multitemporal Data. Scientifica No. 2017 (2017), pp.1-8.
https://search.emarefa.net/detail/BIM-1202690
American Medical Association (AMA)
Sharma, Ram C.& Hara, Keitarou& Hirayama, Hidetake. A Machine Learning and Cross-Validation Approach for the Discrimination of Vegetation Physiognomic Types Using Satellite Based Multispectral and Multitemporal Data. Scientifica. 2017. Vol. 2017, no. 2017, pp.1-8.
https://search.emarefa.net/detail/BIM-1202690
Data Type
Journal Articles
Language
English
Notes
Includes bibliographical references
Record ID
BIM-1202690