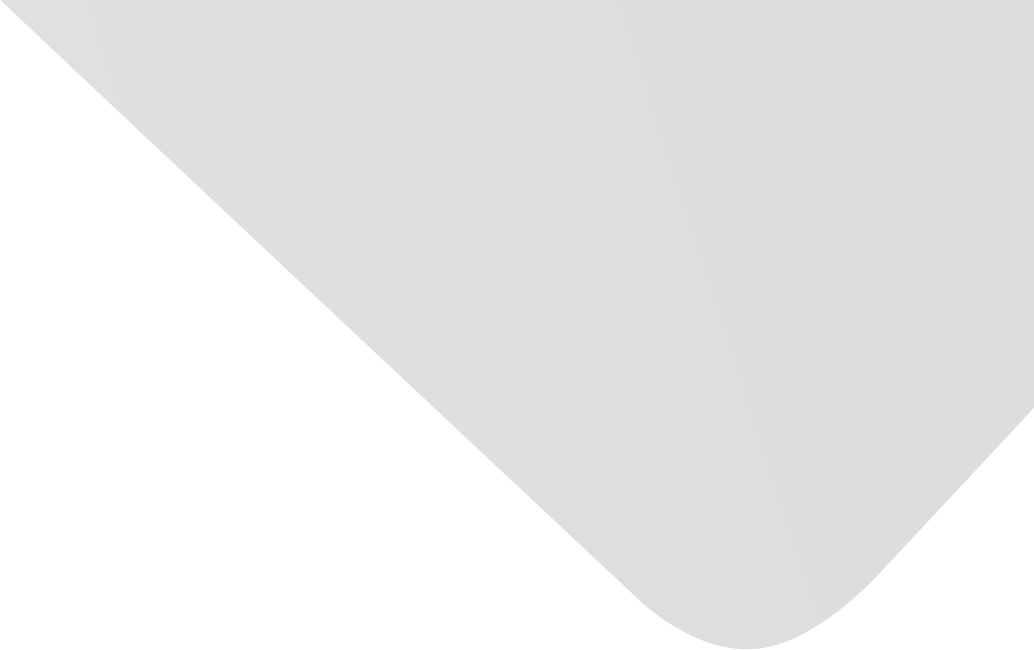
A Brain Network Constructed on an L1-Norm Regression Model Is More Sensitive in Detecting Small World Network Changes in Early AD
Joint Authors
Wang, Huiying
Li, Yunfei
Liu, Hao
Zhang, Sisi
Hu, Haimeng
Han, Jiahui
Qi, Huihui
Wang, Meimei
He, Huijin
Zhao, Xiaohu
Source
Issue
Vol. 2020, Issue 2020 (31 Dec. 2020), pp.1-11, 11 p.
Publisher
Hindawi Publishing Corporation
Publication Date
2020-07-01
Country of Publication
Egypt
No. of Pages
11
Main Subjects
Abstract EN
Most previous imaging studies have used traditional Pearson correlation analysis to construct brain networks.
This approach fails to adequately and completely account for the interaction between adjacent brain regions.
In this study, we used the L1-norm linear regression model to test the small-world attributes of the brain networks of three groups of patients, namely, those with mild cognitive impairment (MCI), Alzheimer’s disease (AD), and healthy controls (HCs); we attempted to identify the method that may detect minor differences in MCI and AD patients.
Twenty-four AD patients, 33 MCI patients, and 27 HC elderly subjects were subjected to functional MRI (fMRI).
We applied traditional Pearson correlation and the L1-norm to construct the brain networks and then tested the small-world attributes by calculating the following parameters: clustering coefficient (Cp), path length (Lp), global efficiency (Eg), and local efficiency (Eloc).
As expected, L1 could detect slight changes, mainly in MCI patients expressing higher Cp and Eloc; however, no statistical differences were found between MCI patients and HCs in terms of Cp, Lp, Eg, and Eloc, using Pearson correlation.
Compared with HCs, AD patients expressed a lower Cp, Eloc, and Lp and an increased Eg using both connectivity metrics.
The statistical differences between the groups indicated the brain networks constructed by the L1-norm were more sensitive to detect slight small-world network changes in early stages of AD.
American Psychological Association (APA)
Liu, Hao& Hu, Haimeng& Wang, Huiying& Han, Jiahui& Li, Yunfei& Qi, Huihui…[et al.]. 2020. A Brain Network Constructed on an L1-Norm Regression Model Is More Sensitive in Detecting Small World Network Changes in Early AD. Neural Plasticity،Vol. 2020, no. 2020, pp.1-11.
https://search.emarefa.net/detail/BIM-1203190
Modern Language Association (MLA)
Liu, Hao…[et al.]. A Brain Network Constructed on an L1-Norm Regression Model Is More Sensitive in Detecting Small World Network Changes in Early AD. Neural Plasticity No. 2020 (2020), pp.1-11.
https://search.emarefa.net/detail/BIM-1203190
American Medical Association (AMA)
Liu, Hao& Hu, Haimeng& Wang, Huiying& Han, Jiahui& Li, Yunfei& Qi, Huihui…[et al.]. A Brain Network Constructed on an L1-Norm Regression Model Is More Sensitive in Detecting Small World Network Changes in Early AD. Neural Plasticity. 2020. Vol. 2020, no. 2020, pp.1-11.
https://search.emarefa.net/detail/BIM-1203190
Data Type
Journal Articles
Language
English
Notes
Includes bibliographical references
Record ID
BIM-1203190